View from Silicon Valley
Previously I’ve written about the pronounced differences that you can find in tech companies between teams specialising in qual, ‘traditional’ quant and big/behavioural data. This isn’t unique to tech but it’s certainly more obvious in our industry.
I’ve written about some of the opportunities for research teams and agencies to complement behavioural data by adding context to what the data is telling us. I have to be honest though – at some point we’re all going to have to take the plunge and learn some data science. Simple as that.
If you’re running a brand tracker, what happens when your stakeholder completes a predictive model to identify patterns in their data that foreshadow changes in market share in real time? If you’re a qual specialist, what happens when your client launches an AI tool that automatically mines social media data and has in-depth discussions with consumers on its own?
These examples are happening right now in the companies I talk to every week, and the implications of work like this will be felt by everyone in our industry.
We don’t all need to become data scientists but if we’re to continue helping our clients and stakeholders make more informed decisions, then we’re going to have to understand that world more than we do right now.
But I’ll admit something: I was nervous about starting.
For years we’ve been excitedly talking about big data but nobody ever seems willing to admit that taking the first step can be daunting. I wanted to share my experience of starting my journey in the hope it might encourage others to do the same.
I’m a quant by training but I’ve always safely cowered behind the walls of statistics software, SPSS. The idea of learning a programming language was confusing and I had no idea where to even start.
I’ll let you into a secret – it’s much easier and faster to pick up than you think. And to be clear, I had zero previous experience of writing code.
So don’t be worried, just give it a go. Here are the three things I’ve learned from engineers in San Francisco that you should keep in mind:
1. Focus on one language – I recommend Python
Don’t be put off by the dizzying array of possible programming languages. Just start with one. Python is the lingua franca of data science and there are a huge number of very accessible – and free – courses you can do online.
2. That code you’re struggling with has already been written before
Engineers are expected to share their code. Think of it like building with Lego. You don’t need to know how the bricks are made, you just need to click them together. Websites such as GitHub and Stack Overflow are full of chunks of code that you are expected to borrow, so don’t be shy in asking or searching for help. This is an open source world.
3. You’ll never need to write models from scratch
Even with something like machine learning, which sounds terrifyingly complicated, the models have all been packaged up for you. You just need an idea of what you’re trying to do and then you take a model off the shelf – try it and see what happens.
I hope that knowing you’re not alone in being nervous about starting helps, but keep those three things in mind and try that first step. We all need to do it and we’re running out of time. Let me know you how get on.
Matt Taylor is consumer insight lead at Twitter
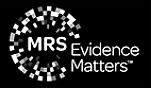
We hope you enjoyed this article.
Research Live is published by MRS.
The Market Research Society (MRS) exists to promote and protect the research sector, showcasing how research delivers impact for businesses and government.
Members of MRS enjoy many benefits including tailoured policy guidance, discounts on training and conferences, and access to member-only content.
For example, there's an archive of winning case studies from over a decade of MRS Awards.
Find out more about the benefits of joining MRS here.
0 Comments