Journey to the dark side
Machine learning (ML) and marketing go way back. By the mid-80s, few areas of quantitative work had escaped its cold touch. Alongside traditional methods, it helped optimise products and services, develop segmentations and predict customer churn. ML was never a crutch, more a dirty and lazy shortcut, when traditional methods could achieve the same or better on small, simple surveys. When the aim is to investigate, test hypotheses, and then transcribe understanding into a summary model, ML was considered cheating and very few dared lead with a ‘black box’ algorithm.
To be fair, there isn’t much particularly different about the maths; it is all in the execution. Classical statistics and ML represent a clash of philosophies about how data should be analysed. We have long hoped to reconcile the two.
With the detail and breadth of today’s data and the need to wring every bit of value from it, the old ways no longer cut the mustard. We rely almost exclusively on ML and there are five chilling reasons why:
Exhaustive Search
ML considers all combinations, tenaciously hunting out unanticipated relationships in data. Admittedly, this ‘everything against everything in every way’ analysis builds on anomalies. These happen-chance relationships, even those that make sense in hindsight, combine to form a tempting but short path for insight analysts to tread. This hunt, however, can also snare mind-blowing insights that survive scrutiny, leading to those ‘Hey, why didn’t we think of that?’ moments. The race for competitive edge is a high stakes parlour game of risk and reward for anyone who wants to play.
Objectivity
Perhaps worryingly so, the results of traditional models vary with levels of analytical experience, favoured approach and domain expertise because the modelling process requires iterative interpretation. Alternatively, ML leaves no room for tinkering and judgement calls. ML is agnostic. It does not care for agendas nor endeavours to rehash ‘known truths’. There is something so refreshing about this.
Flexibility
The argument that traditional approaches are the holistic and pragmatic options is one I find increasingly difficult to entertain, considering how woefully they cope with disparate data types and sources. Shown enough data, any ML algorithm will grow to handle any level of complexity. There is no fuss over whether a particular data distribution violates one too many assumptions or whether the most appropriate test is applied. Classical statistics busies itself with the accounting system of doubt while ML coerces confidence.
Speed
Classical analysis is an exploration lasting a few days to a few weeks, whereas a machine may well return a serviceable answer within minutes. This has dramatic implications on cost and turnaround. Conscientiously sifting through data does not pay dividends when the competition are machine-learning circles around you. There is no alternative but to reach for the same devastating weapon.
Accuracy
Traditional models often expose ML as temperamental and haphazard, suckered by quirky, sparse data. However, I can cite many more examples where ML has left traditional approaches in its dust. Deployed directly into the business to impact customer experience, this increased accuracy is a money printer.
Posturing between classical statistics and ML would be wholly academic if our mission were not to weave real stories from data. We can falter easily when we post-rationalise ML’s brute force solutions in search of fresh insight. It is seductive. We have never found balance.
Its insatiable greed is undeterred by biased, dodgy data. It does not care about context, transparency, narrative, the rhyme or reason. Nothing it does can be accepted at face value and so it remains ever our dirtiest solution to complex problems.
Given our datasets can no longer be leveraged thoroughly inside reasonable budgets and timelines without ML, sacrifices must be brought. While we are very much at the mercy of ML, truth be told, we are already drunk on its power and would not change a thing. Perhaps you too have sensed it. We are loving every moment. Our journey to the dark side is complete.
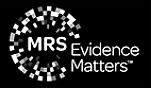
We hope you enjoyed this article.
Research Live is published by MRS.
The Market Research Society (MRS) exists to promote and protect the research sector, showcasing how research delivers impact for businesses and government.
Members of MRS enjoy many benefits including tailoured policy guidance, discounts on training and conferences, and access to member-only content.
For example, there's an archive of winning case studies from over a decade of MRS Awards.
Find out more about the benefits of joining MRS here.
0 Comments