Drag, drop and done?
Behold the age of automated insight. We’re building machine learning (ML) pipelines, fine tuning and artfully blending cutting edge algorithms into even more powerful prediction engines. Except, we’re not. Not anymore. That is all old hat. Shocking, yes, I know. Until very recently, ML required a guiding hand, intuition and long hours of tinkering. No longer. It’s been automated.
‘AutoML’ has a certain air of tautology, don’t you think? We have been freed from the drudgery and guesswork of ML as it iterates through all the likely algorithms and options, tests everything out and returns the best performing model. All you need is a dataset with a variable (aka target) you’d like to predict, and everything else is handled by the same four lines of copy and paste code.
This approach has become commonplace overnight as open source ML packages approach maturity. Commercial versions, known as ‘no code ML’ platforms, are already among the most dominant analysis options available. ‘You don’t really need to know what you’re doing’ may seem a little hyperbolic, but as the months roll on, ever closer to the truth.
ML hasn’t cracked ‘feature engineering’ – this is the hypothesising of meaningful inputs for a model and arguably remains the task of the analyst. Perhaps not for much longer – AutoML’s brute strength computing achieves surprisingly serviceable results. On a practical level, within marketing at least, differences between ‘human guided’ ML and fully automatic models are near negligible and therefore commercially inconsequential.
This finds me hard pressed to sell my ‘hand crafted’ attempt, developed upon years of maths, domain knowledge, intuition, painstaking exploration and sweat, given that whatever I produce may very well perform comparably when pitted against an infinitely cheaper, automatically generated alternative. Though I hasten to add that there is nothing to guarantee such automatic models will be intuitive or make the slightest bit of real world sense. This risk though, is inherent to ML generally and not a particular failure of its automation.
AutoML is not winning data science tournaments yet, but it’s just a matter of time before it forever ruins competitive predictive analytics. Just as intended, the most talented, battle hardened geeks among us may soon be free to pursue more worthy objectives, say weekend walks in the fresh air.
Implications to market research are hardly as trifling.
How we value analysis
We increasingly combine studious, hands-on statistical techniques with quicker, hands-off ML. Over the course of the past four years, we’ve left many traditional methods behind, progressively automating, shaving the time we spend on modelling from weeks and days, to hours and minutes. The definition of what constitutes ‘real analysis’ must invariably shift to reflect the competitive analytics landscape.
How we upskill
We’re changing the skills we hire and cultivate. Not long ago, we stepped away from enterprise software, in favour of open source and do-it-yourself coding. We are now finding our path back again. This means that the saintly trifecta of business, statistics and coding skills is no longer a prerequisite to success – ‘citizen data scientists’ are doing just fine.
This is echoed in the big data landscape. The once touted industry disruptors are in freefall as cloud-based services from Microsoft, Amazon and Google bite down. Being able to intuitively navigate the fineries of algorithms or spin up big data clusters are not marketable skills in a drag-and-drop world. If you were expecting megabucks after mastering this stuff, I have rude news; CVs bloated with names of algorithms are already first to the shredder.
How we leverage data
Finally, this latest generation of ML makes bespoke transferable models economically viable for the very first time. Models can be trained on one dataset, and then carry on learning from another, progressively getting ‘smarter’ and less error prone. We will soon be growing our own brand or context-specific cognitive engines, no doubt taking great pains to boast about them. It’s going to be a lot of fun. Expect this to be the shift in focus in the coming months and be ready to know a lot more about it.
The next era
Now that we’ve settled on realistic expectations for ML, its strengths, weaknesses and trajectory, it prompts us to address its commercial reality. We start by reconciling ML’s promise and majesty with its stupefying mediocrity. ML has been democratised, making this once big ticket and aspirational consultancy piece a cheap commodity. Everyone’s got it. Everyone can do more or less the same job with it. To this point, I have heard many voices of late, some outraged and defensive, others exasperated and deflated.
These are joined, however, by a full-throated acknowledgement of the glaringly obvious – automated ML does not break boundaries. No marketing problem is solved by cookie cutter approaches. AutoML cannot formulate winning data solutions. Rather it represents fruitful ground for truly novel, game-changing opportunities that can only accelerate data-driven thinking, widen the scope of, and deepen investment in, analytics.
Most notably, this progress allows for smaller, diverse teams, with more space reserved for innovation, problem solving, learned explanation and storytelling, and less for data wrangling, number crunching and superficial description. In this roundabout way, this new era ultimately ensures that market research, the kind that lends competitive advantage, remains the calling of a new trifecta; that of the technical, curious and resourceful.
Ryan Howard is director advanced analytics at Simpson Carpenter
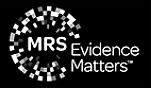
We hope you enjoyed this article.
Research Live is published by MRS.
The Market Research Society (MRS) exists to promote and protect the research sector, showcasing how research delivers impact for businesses and government.
Members of MRS enjoy many benefits including tailoured policy guidance, discounts on training and conferences, and access to member-only content.
For example, there's an archive of winning case studies from over a decade of MRS Awards.
Find out more about the benefits of joining MRS here.
0 Comments