All that glitters is not gold
It’s time to face facts. Building data science products is a risky affair. Few ideas gain traction and those that do are short-lived. Even the flushest among us struggle to compete. When we dare, our punts arrive as either unsound, redundant, or as one of many in a maturing market.
All the while, we’re barraged by stories of great triumph, syrupy press releases that will never mention return on investment. We know why, though. Without exclusive rights to rare data, it’s a tune which repeats itself with abandon.
As its tempo quickens and more analytics platforms join the fray, our choice is to work harder and cheaper, and in doing so, rinse our industry of all remaining research craft… or continue to innovate, where our highest ambition can only be to co-habit the data industry politely. The third option is to license ever more software, adding overhead without competitive advantage – good luck with that.
So, it raises tougher questions: what are the ingredients of a lucrative analytics offer? Where might our small teams venture where enterprise software cannot? How can we differentiate what we build from self-serve? In the cold light of day, the answers are so direly mundane that they fly in the face of what Silicon Valley would have us believe. Whether you’re head of marketing or a graduate analyst about to run your first logistic regression, developing your own analytical capital will share these four patterns:
Consolidation, never exploration
Our most compelling analytics offers grew by leveraging existing revenue streams where the goal posts did not shift; the business cases are self-evident. They landed with immediate impact, addressing current pains instead of selling new ideas or adding workload.
Teams were never hot-housed away in lab coats but lived the realities of commercial research. This eclipses anything owed to ‘blue-sky thinking’ – whom we always invite to the party, but secretly hope doesn’t show. Too many times this fickle friend drank the good stuff then left without paying the bill.
Shared responsibility
The hassle-free option is to put your faith in a technical whizz. They plough ahead but the trap is soon sprung. The demand for these folks is eye watering; they don’t stick around, their work never resurrected. When teams progress slowly, stability and ownership are maintained. Shared knowledge and thorough documentation are the hallmarks of intellectual property – not code bases which, for performance and security reasons, need overhauling every few years. Code alone is fool’s gold.
Prototyping and iteration
Without incremental improvement, enthusiasm wanes overnight unless sharp bursts of work gather momentum and interest. Teams follow a flexible map, sans drum-rolls and curtain reveals. Agile is the watchword. This turns out to be the only way of delivering something not already two years behind the competition, chock-full of features initially requested, but for which no one truly cares, nor is willing to fund.
Creative assembly
In my arrogance, I spent years sweating away on novel ideas, only for computer science students, half the world away, to share better versions as throwaway mid-term submissions. Again, and again, I would hang my head as their code hummed into life on my server. It took me too long to accept this as the rule.
But I’ve also been privileged to work on equally original propositions. The ones that shine most brightly didn’t break new ground or reinvent the wheel. My colleague Frank Hedler and I were once pinned in front of Research Live’s innovation award panel, Dragons’ Den style, and I paraphrase, “But isn’t this just mostly open source?” We smiled and nodded.
Ultimately, humility must be our niche; this industry has never humoured scores of programmers in five-year development cycles. It rewards mashing up obscure and freely available code snippets, and shamelessly piggybacking on big tech’s services. It cries for a modicum of restraint before setting sail to frontiers new in the hunt for buried treasures, and an appreciation for the economics of the less flashy and focused.
The usual investment template does not sit comfortably within any data ecosystem, less so, in market research. In denial, or in search of an alternative blueprint, one might point to the top research platforms – though couldn’t, without discounting their commitment and silent failure rate; they’ve been digging away for 20 years. Artificial intelligence startups they are not.
The marketing tech race has made building tailored analytics propositions a prerequisite to the next chapter of our story. Its pages are a litany of blistering invention, little gems that inform everything marketers do quantitatively, indeed a great deal of what is now termed applied data science. It is why we always have shiny things.
This would not square if it weren’t for our four enduring success patterns. They guard our way still. Elsewhere, flopping data science programmes are cartoon memes.
It turns out that being constantly under pressure to demonstrate our worth, defend limited budgets and test ideas at the coalface, as we switch tack from one project to the next, is our serious strength. We cannot afford to sustain data science teams for show, nor kid ourselves that all data has value because evidence matters. It makes us less eager to entertain pipe dreams. It forces our hand on the ripcord while the less data-savvy sleepwalk into the next round of funding. Under these tempering conditions, and these alone, we’re surprisingly good at producing diamonds.
Ryan Howard is a freelance data science consultant
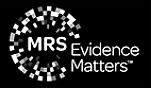
We hope you enjoyed this article.
Research Live is published by MRS.
The Market Research Society (MRS) exists to promote and protect the research sector, showcasing how research delivers impact for businesses and government.
Members of MRS enjoy many benefits including tailoured policy guidance, discounts on training and conferences, and access to member-only content.
For example, there's an archive of winning case studies from over a decade of MRS Awards.
Find out more about the benefits of joining MRS here.
0 Comments