Analytic bias: challenges for the industry
Bias in data analytics is a broad subject, but an important one that I want to address to ensure that we manage bias effectively within the analytics industry. This is because I believe there will be a more public debate on how perceived data bias impacts groups of people, and we need to be ahead of that conversation.
Data analytics has been at the forefront of the national news throughout the pandemic. One of the more challenging areas connected to analytics was the proposed use of algorithms to estimate the exam results of children in 2020. In principle, using predictive modelling to replace exams was not a bad idea. As questions were raised on fairness to pupils, however, the algorithm was dropped and replaced with teacher predictions.
The proposed approach brought to the public conscience the idea of bias in data-led algorithms and the negative impact it can have on a large community of people. There will, of course, have been some potential bias in the teacher-based results, but it is the direct link to data algorithms – and their use in large data sets – that will raise questions.
This is a reminder that, as proponents of analytics, we have a duty to continue to try to address the challenge of bias to
ensure the best results. It is not a new issue for our industry.
I, however, would like to think our research samples are selected carefully, and we collect the right data for the job to ensure we deliver quality recommendations. Despite that, there are specific areas of potential data bias, of which I believe we need to be cognisant.
As a male analytic director, I am aware of the danger that how I lead my team may come with my own unconscious bias. The role of unconscious bias in data analysis is a watch out, and was brought to my attention further recently after reading Invisible Women by Caroline Criado Perez. The book brings to life several observations of analytics where women are potentially disadvantaged because of predominately male-led, data-based decisions. The point is that we can strive to address this type of bias from whatever position we hold in our industry.
I recall, several years ago, many a visit to a shopper insight director in Cheltenham. On the wall was a statement, ‘90% of stores are designed by men, 90% of shopping trips are taken by women’. I am not sure about the source, but it has always served as a reminder to me that I must ensure we have the right mix of minds and instil the importance of diversity when building teams working on a project. Let us challenge ourselves on the personal bias that we might bring. It is a sign of leadership to step back, and accept the input and challenge from others to deliver the rounded approach that removes bias where we can.
The second area to address is how we deal with confirmation bias. Many of us work with internal customers or clients who want data to tell a specific story. We must be prepared to spend time on how an analytic approach is built to provide results with integrity. I have worked on many projects relating to media or promotional evaluation of food or drink items where the exclusion of a simple variable, such as weather data, could lead to misleading results or misattribution of campaign success. We must control the data inputs to build the best analytic approach with the data we have available. That might mean pushing for more information to input into our model because we know it will make a difference to removing a biased result.
Facing up to bias is an industry question, and as artificial intelligence grows, so too do the questions on how algorithmic bias impacts results. There will always be something in any analytic approach that can be challenged, but being aware and sharing our thinking will mean we can protect the reputation of the data analysis, drive out the unconscious bias, and deliver great outputs that secure the role of analytics as a ‘go to’ place for our organisation’s issues.
THIS ARTICLE WAS FIRST PUBLISHED IN THE OCTOBER 2021 ISSUE OF IMPACT.
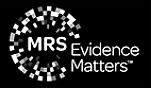
We hope you enjoyed this article.
Research Live is published by MRS.
The Market Research Society (MRS) exists to promote and protect the research sector, showcasing how research delivers impact for businesses and government.
Members of MRS enjoy many benefits including tailoured policy guidance, discounts on training and conferences, and access to member-only content.
For example, there's an archive of winning case studies from over a decade of MRS Awards.
Find out more about the benefits of joining MRS here.
0 Comments