Key takeaways from the MRS Data Analytics Virtual Summit
Twitter is king (for data)
A number of projects cited at the conference used Twitter as a means of accruing large amounts of open access data for analysis. As a public forum, of sorts, Twitter is a much easier tool to use for brand purposes, with Facebook and Instagram having ringfenced their platforms to some extent in recent years.
In one example, Oliver Lewis, global head of insight at Convosphere, worked with the Swiss Ice Hockey Federation on building its interaction with fans. The federation runs the national ice hockey team in Switzerland, and the aim of the project was to try to create engaging content to boost support and identify new avenues for sponsorship.
This meant conducting an analysis of people’s relationship with the federation online, the common characteristics of those followers and the reasons they had for following the federation. Most were millennial males working in major Swiss cities. Key interests, outside sports, were travel, finance, animal welfare, automotive and science.
Lewis’ project focused on Twitter because of the glut of good data that was easily available to him. “We needed to use Twitter as our primary platform due to the openness and availability of its data,” he said.
“Facebook and Instagram are much more closed off now than they once were, and with Twitter you get access to the entire social graph and the content people are posting. You get a much broader view of people’s interests, behaviours and the type of content they engage with. Twitter is much more transparent and there is a lot more data to work with.”
Segmentation is still useful
Tom Morgan, director of analytics at Tapestry Research, defended the value of segmentation as a data analysis tool. “Segmentations have a strange reputation in the data science and analytics world, where they are seen as passe or as slightly old-fashioned,” he said. “But we need these techniques more than ever, and they are incredibly valuable and useful. Just by taking some really simple analysis from the cluster analysis stable, we can get a much greater insight into our customers and our clients’ problems.”
He said that segmentation essentially works on the same basis as the human brain – taking complex systems and boiling them down into a handful of key groups. Segmentation is a “complexity reduction technique”, according to Morgan, and a chance to turn 7.7 billion people worldwide into a set of defined characteristics that can be studied and can therefore drive policy.
Morgan advocated using silhouette analysis, which allows him to understand the quality of segmentation. He said it was important to understand how segmentations can evolve. This could be due to events like Covid-19, for example, but also how they can be affected by how people and organisations in a segment evolves over time, and also the relationship between the segments themselves.
Use a variety of sources for your data
“Data is the new gold, and there has been an explosion in data in the past decade,” said Andy Hopcraft, technical director at SDG Group. The key is to know where to find it.
Hopcraft pointed to four major sources of data he uses: internal, survey, social media and external. Internal data was classified as sales, operations and finance within an organisation – what is happening, and the trends and predictions that come from that data. Survey data shows customer experience and brand preferences, with social media showing what people are saying in the moment and their honest opinions. External data includes data on the economy, weather, world events and what competitors are up to.
Hopcraft captures data from the various sources and then organises it into a data ‘warehouse’, ‘lake’ or even a “cloud data lakehouse”, before analysing it using machine learning and artificial intelligence, as well as simpler techniques. Once that is done, it can be visualised to make it accessible to users. Most of the solutions Hopcraft uses now are cloud-based, and he argued this technology has eclipsed traditional physical forms of data storage.
“The cloud tools we use allow us to ingest vast volumes of data with no impact on the underlying technology,” Hopcraft said. “Getting high value out of low volume data is the challenge. Volume is something that can be easily managed and maintained through pipeline techniques.”
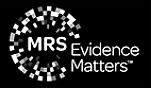
We hope you enjoyed this article.
Research Live is published by MRS.
The Market Research Society (MRS) exists to promote and protect the research sector, showcasing how research delivers impact for businesses and government.
Members of MRS enjoy many benefits including tailoured policy guidance, discounts on training and conferences, and access to member-only content.
For example, there's an archive of winning case studies from over a decade of MRS Awards.
Find out more about the benefits of joining MRS here.
0 Comments