The new insight power couple
As the old saying goes, ‘two heads are better than one’, and the new insight power couple – data science and behavioural science – illustrates this perfectly. The relationship may be in its infancy, but we are already seeing clear evidence of the mutual benefits of this pairing.
Behavioural science can add value to data science in two simple ways – by helping to define the underlying questions and hypotheses that guide data collection, and by offering a behavioural lens through which data can be explored.
Data science can amplify behavioural science understandings by analysing human behaviour on a larger scale: exploring millions of variations, looking for trends or patterns that may have been obscured by large amounts of data. This can also lead to greater personalisation of behavioural science interventions, targeted at an individual level.
Tackling fraudulent claims
Most people who claim and receive benefits are honest. Some, however, receive benefits for which they are ineligible. This might not always be because of dishonesty; sometimes, the application forms are unclear, or there is a misunderstanding about what counts as ‘work’ and what doesn’t. Nonetheless, paying benefits to people who shouldn’t be receiving them is a burden for governments.
In 2018, the New Mexico state government, in the US, decided to tackle this problem with a new strategy. It used data science to analyse benefits claims to establish patterns in applications. This data could suggest which applications had a higher-than-average chance of being fraudulent, but the information was not proof enough to act. Investigating each individual with a higher-than-average chance of committing fraud would also have been time-consuming and costly – and mislabelling someone as making fraudulent benefit claims risked causing long-lasting detriment.
Partnering with Deloitte and Harvard University, the New Mexico government realised that incorporating behavioural science might offer the answer to this ‘last mile problem’. Re-examining the big data they had collected through a behavioural science lens, the team identified three key moments in the application process when claimants gave inaccurate information: entering the reason for leaving employment; answers to ‘Did you work this week?’; and responses to whether claimants had completed at least two ‘work search activities’.
The researchers realised they could use simple, yet effective, behavioural science nudges at these moments:
● Key moment 1: although claimants knew their previous employers would be contacted, this detail was not salient and so was easily ignored. To fix this, people were shown a copy of the letter that would be sent to their previous employer to verify their reason for leaving before they submitted their answer.
● Key moment 2: the team realised that individuals were finding it easier to be dishonest when dealing with an inanimate computer screen, rather than a person. On top of this, lying about working for only one or two hours didn’t seem immediately dishonest. To address this, the team used priming and social norms: a pop-up screen would appear before claimants entered the information, saying most people report their earnings honestly. They also made claimants sign their initials next to their statements, because research has shown that personal attestation can improve honesty.
● Key moment 3: Every week, claimants had to make a plan and commit to enquire about, apply for, or interview for at least two jobs in the coming week. When they completed the next week’s form, they were reminded to review these goals.
The New Mexico state government had used data science for years to try to reduce fraudulent benefit claims, but it was not until it applied behavioural science insights that it understood the significant problem of mundane deception.
One of the key ways big data can work with behavioural science is to create more targeted behavioural interventions. Data science can pull out macro and micro trends or patterns, and extract material specific to smaller groups – even down to an individual level. New tech, such as the Internet of Things, means data can become available in real time, allowing for advanced personalisation and nudges tailored to context.
Nudge* is a financial education agency specialising in computer-based training programmes to help adults understand their financial rewards and benefits in the workplace. It discovered that, in spite of the benefits to users, most of these programmes have low levels of engagement and people tend not to complete the training.
Nudge* realised this was primarily caused by two things: the financial education training does not occur at a salient time; and people tend to prefer the ‘head in the sand’ method of thinking about their financial future – known as the Ostrich Effect.
Nudge* had access to a huge volume of information about those who had signed up to take the course, ranging from small details – such as when they tended to log on to the system – to larger ones, such as their salary. Using this information and inspiration from behavioural science literature, it developed a way of offering personalised nudges to encourage people to do the training at the right moment.
Research shows that instigating a change of behaviour is more likely if behavioural routines or habits are disrupted at the same time. The most successful nudges are triggered when someone experiences a significant life change, such as when they move home or change salary.
The nudges include emails, texts or WhatsApp messages – depending on people’s personal preference – which contain advice and information specific to an individual’s current life change; for example, what happens to your pension now that you have moved home?
Messages are personalised depending on the person’s age and salary, and encourage behavioural goal setting and benchmarks (what we call anchors) for saving, and use social norms by offering tips and tricks from peers.
These strategies increased platform logins by 34.9% in the month after the introduction of nudge communications.
This article was first published in the July issue of Impact.
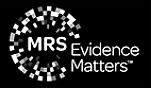
We hope you enjoyed this article.
Research Live is published by MRS.
The Market Research Society (MRS) exists to promote and protect the research sector, showcasing how research delivers impact for businesses and government.
Members of MRS enjoy many benefits including tailoured policy guidance, discounts on training and conferences, and access to member-only content.
For example, there's an archive of winning case studies from over a decade of MRS Awards.
Find out more about the benefits of joining MRS here.
0 Comments