The misconceptions of AI
Scientists claim that artificial intelligence (AI) is the most profound ‘general-purpose technology’ of our era, a harbinger of progress and growth akin to the discovery of electricity or the invention of the steam engine.
AI is everywhere these days. Once a concept visited only by science fiction authors, these two letters are now omnipresent in our environment. McKinsey’s AI adoption report estimates its global economic impact will reach $13tn by 2030; companies that embrace AI will double their cash flow. Unsurprisingly, businesses are scrambling to develop their AI strategies, and work out how best to make use of this exciting new field.
In the excitement to understand and adapt to the changing landscape, however, numerous misconceptions have arisen around AI’s nature, abilities and implications. These threaten to prevent businesses from unlocking the full potential of AI and hinder its uptake.
AI is unbiased
The popular media image of androids is of unemotional, decision-making machines that use pure, cold logic to weigh the options and arrive at a course of action that is rational and free from the discriminatory biases that pervade human judgements. Contemporary research, however, shows that AI systems can perpetuate – and even amplify – gender and racial discrimination. Where would an artificial intelligence pick up such a human flaw? The answer lies in its training.
Most AIs are trained to perform their tasks by being exposed to large volumes of data. By extracting the relationships between characteristics of the training data and the end outcome that represents the decision the AI is aiming to emulate, the AI eventually generalises a set of rules that allows it to perform its function.
In this sense, it is merely generalising relationships that exist within its training data and extrapolating these to new information when making decisions live, in situ. So if there is bias in the training data, this will be expressed in the AI’s functioning.
Given that biases are part of the human experience that generates data, this is a real concern, and something AI practitioners should keep in mind when training AI systems.
Deep learning is synonymous with AI
Almost all of the visible and highly touted breakthroughs of the AI renaissance, such as image and voice recognition, have been powered by deep learning – an AI-training methodology that is the culmination of a series of smaller breakthroughs in the parent field of neural networks.
Neural networks are themselves an example of the tendency of AI research to adopt and drop algorithms rapidly. Where once knowledge-based systems devised by costly human ‘knowledge engineers’ represented the peak of AI practice, neural networks – particularly those incorporating deep learning – have led the charge recently.
The success of these algorithms, made possible by the explosion of computing power available to AI researchers, has led to the term ‘deep learning’ entering general conversation. This, in turn, has created a perception that deep learning and AI are one and the same thing, which is an oversimplification.
While deep learning is an undeniably successful learning strategy in specific problem domains, contemporary scientists believe that AI will require dozens of unique techniques working in unison.
The US and Europe are AI leaders
In Kai-Fu Lee’s most recent book, AI Superpowers, the obsession with American and European AI superiority is questioned in light of the meteoric rise of China’s thriving AI ecosystem.
Lee is well positioned to argue this given his pioneering work in AI, both in academia and as an executive at Microsoft, Apple and Google China. He gives a range of insights into the diverse cultural, governmental and scientific factors illustrating why China is poised to emerge as a new world power in AI.
Unlike in the West, the growth of online-to-offline retailing in China has led to services – such as WeChat – that don’t merely exist in an online world. Owned by tech giant Tencent, WeChat has evolved from China’s answer to WhatsApp to an all-in-one app that lets users order food, hail rides and stream content, as well as a wide range of real-world services such as hiring a babysitter.
This vibrant ecosystem of services enables the accumulation of the world’s largest and most interconnected dataset, giving a 360-degree overview of consumers’ preferences and behaviours. This information is a goldmine for data-hungry AI algorithms that increasingly need more data to improve performance.
This gulf in rich and large consumer datasets will only increase between China and the West, paving the way for China’s dominance in the world of AI.
Human-level AI is around the corner
AI success stories abound, and the modern consumer has unprecedented access to powerful AI with uncanny abilities to understand speech, recommend films and even diagnose illness. AI has come so far in the past few years that it’s only natural to assume that human-level AIs will be among us soon.
The reality is, however, that while AIs have displayed remarkable feats, those attainments have occurred within tightly defined problem domains. Most of the ‘AI achievements’ reported in the media – for example, Google DeepMind’s Go-playing bot, called AlphaGo – are not AI breakthroughs, but are extraordinary engineering feats using innovative concoctions of existing techniques. While AlphaGo and its even more powerful successors can out-compete the very best human players, they would be less able than a five-year-old to distinguish and identify simple geometric shapes.
The AI boom is exponential
AI research has already experienced a series of hype cycles, with prolonged periods of funding cuts. Is another AI winter about to hit us in the near future? Given the pace and volume of venture capital cash flowing into AI start-ups these days, it is very likely that many of these companies are significantly overvalued.
Unlike in the past, however, we now have a plethora of transformative real-world business use cases that depend on deep-learning technologies, from self-driving cars to cancer-detecting ‘bots’. So although the AI hype is likely to dampen over the next few years, progress is expected to continue at a slower pace.
AI’s many triumphs are testament to a field that has come a long way since its inception in 1956. While its current capabilities are impressive, there’s still a long way to go before we see the emergence of artificial general intelligence matching human-level intellect.
Significant progress is being made, not only in the West, but in China too, and a wide variety of algorithms are contributing to the successes of AI. Careful attention is required to ensure that the AI systems of the future are unbiased – and, while the explosion of activity may recede somewhat, the future of AI is bright.
Aji Ghose is head of research and analytics at Sky
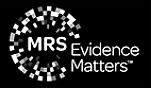
We hope you enjoyed this article.
Research Live is published by MRS.
The Market Research Society (MRS) exists to promote and protect the research sector, showcasing how research delivers impact for businesses and government.
Members of MRS enjoy many benefits including tailoured policy guidance, discounts on training and conferences, and access to member-only content.
For example, there's an archive of winning case studies from over a decade of MRS Awards.
Find out more about the benefits of joining MRS here.
0 Comments