The meeting of human and machine
In August 2018, Andy Haldane, chief economist of the Bank of England, warned that artificial intelligence (AI) would make many of our jobs redundant. He speculated that the changes in the job market could be worse even than during the Industrial Revolution, causing mass redundancies in white-collar jobs.
“This is the dark side of technological revolutions, and that dark side has always been there,” Haldane said. “That hollowing out is going to be, potentially, on a much greater scale in the future, when we have machines… replacing the cognitive and the technical skills of humans.”
As always when redundancies are announced, our first thought is: what happens to my job?
In 2016, Carl Benedikt Frey and Michael Osborne, of the University of Oxford, ranked all 702 statistically recorded occupations in order of the probability that they will be automated away. Ironically, they did this by training an AI to recognise the sort of activities that will be profitable for the machines to do, and to work out how much of each job is made up of those activities.
In their estimation, market research is going to change dramatically in the next few years. The probability that a job in research will disappear is well above average: 61%. This means research jobs are much more under threat than those of fashion designers, who have less than 1% chance of being replaced, but much less so than those of fashion models, whom Frey and Osborne predict have a 98% chance of being replaced.
Semi-skilled tasks
Research jobs are not, of course, homogenous. Frey explains that it’s not the job title that gets automated, but the activity. The jobs that will survive, he says, are “likely to be intensive in creativity, in complex social interactions… things that computers are still relatively bad at”.
Probably not all – or perhaps not even most – of the early impact of AI on research will be to create machines to do high-level analytical thinking. Optimising existing business processes can deliver significant benefits. Consider robotic process automation, or RPA, which is already using AI to automate semi-skilled office tasks.
Forrester Research predicts that the global RPA market, worth $250m in 2016, will grow to $2.9bn by 2021. Essentially, a company installs software that looks out for repeated administrative processes – for example, processing forms – and takes them over, working like an advanced version of an Excel macro. The AI component then gradually optimises and standardises the process across the organisation. “It’s not just high-volume, low-complexity work. Look at inefficient office-based activity… it might also be people carrying out complex tasks that consume a huge amount of time and are quite prone to error,” says Terry Walby, CEO of thoughtonomy, one of the largest RPA vendors.
RPA’s early successes have been in process-intensive sectors such as financial services, but it can be trained in any environment. Walby argues that any organisation that employs people to do repetitive clerical work can increase productivity by using AI.
Pippa Bailey, head of innovation at Ipsos Mori, says: “There are quick wins from AI in terms of automation and making some processes faster – sometimes things that have nothing to do with the research data. One of our offices uses AI for organising its office space.”
Quick wins
Lewis Reeves, CEO of Viga, says we should not underestimate the capabilities of AI to improve employee creativity and productivity on existing services. “We need to spend more time on the areas that have the most value,” he says. “Any tasks that don’t need to be done, we can pass to machine learning.”
Because so much of Viga’s survey work has been customised, it is not suitable for basic automation, but AI-driven standardisation is a focus for Viga. Reeves has found some quick wins by training an AI on its database of past surveys. “We have asked respondents 250m questions in the past couple of years, so we are working on predictive question building.”
This is one area in which human involvement has sometimes led to sub-optimal outcomes, he says. Viga’s clients may not want a fully automated solution, and prefer to create carefully structured, custom research. While experienced survey compilers may target questions effectively in their area, slight differences in phrasing or scales mean that responses may not be easy to compare over time or across regions. So a simple, expert system that guides whoever creates the survey to use standardised phrasing and structure leads to value for clients.
“AI will not always be about creating new avenues of research,” Reeves says. “These techniques give us greater power by bringing together more datasets for comparison.”
We must also resist the temptation to give too much power to AI in research, argues Ryan Howard, head of analytics at Simpson Carpenter – not because we’re Luddites, but because that’s a bad way to use the technology. He warns that one of the most important roles for people will be to ensure that using machine learning does not become an aimless process of mining data that wastes time on spurious correlations in the sample data.
“We have got to be as rigorous with machine learning as we are with more traditional analyses. If not, machine learning arrives at the wrong answer, just more quickly,” he says.
Howard identifies three tasks for an analyst working with research data in this world. The first is to understand the algorithms intimately, because then they can also understand how the AI will respond to inconsistencies in the data.
The second is to apply “our domain knowledge” – to investigate only the sorts of questions that are sensible, or that might benefit the client. AI can find interesting and complex patterns in data that may tell us something about the world, but which may also distract from the business problem.
The final caution is to test and validate conclusions to guard against overfitting. This is common in machine learning: the AI creates a complex relationship between all the data points that fits the sample data extremely well – but some of those relationships are just noise, not signal. This means it is a bad model of underlying patterns, and so has little predictive power.
Adding value
Rather than remove the need for expertise, Howard says, AI makes it even more important, because the researchers have to use the first phase of number-crunching to formulate some hypothesis – one that is both testable in the data and useful to the client.
“That’s how we move from just code that predicts an answer to being a consultant, adding value to business,” he adds.
Constant communication with the client is vital, Bailey adds. Because machines that learn can only do so with fresh data, it’s important there is a shared understanding that all conclusions are contingent on a cycle of testing and refining, that all predictions have some margin of error, and that it’s a joint project to reduce it over time.
As a result of the hype around big data, many clients listen more to their data scientists, and AI extends that trend. Kyle Findley, director of data science innovations for Kantar Insights, has helped develop products such as the ConversionModel – a measure of brand equity – and FutureView, a measure of consumer early adoption and in-market influence. He believes that AI emphasises how important the researcher’s view is for the client, because the researcher can bridge data science and what it means in terms of a real-world brief.
“There has been an influx of data, and methodologies and techniques, most from a non-market research background – from tech companies or, at best, digital marketing companies,” Findley says.
“As market researchers, when we have not had those abilities ourselves, we have relied on these companies to supply them. But they do not have the same paradigm in their heads that we’ve cultivated with our clients over decades.
“A lot of those companies and suppliers describe the way that consumers think and speak. We have to fill the gaps, translating that into research insight for our clients – which often relies on higher-order concepts. Anyone can deliver sentiment in social media, but the client question is, what are the emotions that I am tapping into, the basic human needs that I’m fulfilling?”
Rosie Hawkins, global director of client solutions for Kantar TNS, says: “We have always needed people skills, but given that we are now starting with massive amounts of unstructured data, we need absolute clarity on what the client needs to get out of it.”
This will boost qualitative research, Hawkins argues. Remember that Frey and Osborne’s research found that the most secure jobs after automation will be those that require creative, imaginative or interpretative skills. AI, Hawkins says, increases the value of some of the researchers who we would often consider to be under threat.
Fast feedback
AI, nevertheless, has advantages that existing techniques cannot match. One of the most important is to create fast feedback on what doesn’t work.
Scott Young is the European CEO of PRS In Vivo. His company works mostly with FMCG brands and has created the AI Pack Screening Model, which uses AI based on its experience to predict which packaging designs work best.
“We see many new products that fail in the market, and a lot of our evidence suggests it’s because of packaging not breaking through clutter and communicating the key proposition clearly,” he says. This packaging has been researched, but Young argues that marketers are increasingly forced to cut corners, and are putting too much resource into bad ideas. “They are using ‘judgement’,” he jokes. “This may involve very cheap and sub-optimal research. It can mean marketers sitting in a room, looking at 10 designs and picking the three they like, or using automated tools that show the product out of context, or doing a very quick online survey.”
When PRS In Vivo looked at the outcomes from packaging changes, it discovered that those surveys missed some potential problems – most often, how a product would stand out on the shelf – and didn’t pick up emerging trends. So it trained an AI on its database of designs, coupled with data of their post-launch success. “That allowed us to create the beginnings of a predictive model,” he says. “A way of looking at new designs that we know corresponds to how we do studies with shoppers and the metrics that link most to market success.”
Supplement not substitute
AI, again, is only part of the creative process. Young does not plan to use the tool with his clients to replace product testing. Instead, it will force them to look at new ideas in a consistent manner – basically, to tell them which ideas to throw out, so they can put all testing and development resources into ideas that have a better chance of succeeding.
“It’s a supplement to a human process, rather than a substitute,” Young says. “That’s why we call it AI screening. We can’t put a design into a system so it can spit out a sales number. We are not there yet – nor do we necessarily think you are ever going to get there.”
While most AI in research emphasises its limitations, it is important to recognise the biases and myopia of human intuition, and to use AI to save us from ourselves. The marketers and respondents who make poor packaging choices are trying, and failing, to make a good decision. In future, knowing where to draw the line – so that we get the best of the AI and the best of the human in drawing inferences – will be an essential research skill.
Co-founder of Pulsar, Francesco D’Orazio, was employing deep-learning AI tools to create insight earlier than most researchers. Pulsar Vision, the first market research AI tool to make sense of images on social media, was launched in December 2015. Six months later, Pulsar introduced Pulsar Modules – a suite of horizontal tools that clients can use to spot emotion, or do text extraction or image tagging in different types of (predominantly social) data.
One of the things that D’Orazio’s team has learned is the potential of targeted tools – what it calls ‘vertical AI’. New modules, launched at the beginning of September, focus on food, travel, apparel, colour, logo detection, celebrity, video analysis and demographics, with algorithms trained to spot these aspects of visual data more precisely.
D’Orazio explains that research questions are often highly specific, so tools should reflect that focus.
“We are working with a food-industry client now, looking at 15,000 vegan meals on Instagram. Whenever someone posts something about a vegan brunch or vegan dinner, we compile the list of ingredients that we have recognised. Our aim is to come up with recipes for the best vegan three-course meal, based on what people like the most.”
A standard, horizontal image-recognition AI module would produce many image tags – such as plate or glass – that tell the researcher nothing of interest. The more focused food AI module picked up 200 ingredients and dishes, which, after eliminating a few false positives, created a list of popular ingredients (intuition would have misled many of us: avocado came in tenth, and chocolate was used in 36% of the dishes).
Identifying patterns
D’Orazio explains: “I’m also working with a retailer to understand the perfect festival look. So, take 100,000 images of people going to festivals in the UK, identify the items they are wearing in those pictures, and quantitatively put together the look that is most common or receiving the best comments, or the most engagements, based on how people react to them.”
For this type of research, however, do we know that techniques using 100,000 images will do better than an ethnographer with 100? D’Orazio, trained in ethnographic research himself, is trying to find out. Pulsar is working with Manchester University’s School of Arts on the differences between pattern recognition in humans and machines, and sponsoring a PhD on this subject, starting in 2019.
“We have built something that allows you to scale up that approach on a quantitative basis,” he says. “As humans, we are good at identifying patterns, but, sometimes, our appetite for spotting those patterns can be misleading because we want to reduce complexity – and, sometimes, we reduce it at the expense of the important information.”
Even the most powerful AI needs a researcher to help interpret the world. “We’re the ones that populate the hypothesis. We define the lens that we use to look at the data,” says D’Orazio.
“The biggest challenge is getting out of the ‘big data’ paradigm that we’ve been sold for the past 10 or 15 years. It says that the more data you have, the more interesting the ideas that emerge from that data. That is not true. That is just not what happens.”
What is artificial intelligence?
In 1945, Vannevar Bush, who had headed the US Office of Scientific Research and Development, wrote an article for Atlantic Monthly called ‘As We May Think’. At a time when even the existence of what would become the computer was still a state secret, he speculated on a machine that would soon aggregate “the associated opinions and decisions of [our] whole experience”, to help us make decisions. In 1956, the first academic conference on the subject was held, where the name ‘artificial intelligence’ was coined. Then, as now, however, the participants struggled to define what this was, and AI today covers many applications.
AI – as applied to the problems in which a researcher would be interested – is an expert system with three components: a database; a way to interpret that data and make inferences from it; and a means of communicating its insights to the outside world.
These insights can have two forms. Decision support offers options and issues to human decision-makers, usually with some expression of their likelihood. Decision-making goes beyond a human level of knowledge and experience, and is at the heart of automation.
The inference engine at the heart of an AI uses algorithms – sets of rules that define a computation. These algorithms are the product of machine learning, which has used some way of associating the data to create rules and processes, rather than having them completely defined by a human. This can be continuous, ideally improving the algorithm over time, either with or without human intervention.
Today, all AIs are task-specific – a general AI is still the elusive goal. Dr Abdalla Kablan, CEO of AI specialist Hippo Data, calls them ‘Einsteins’ – while they can be trained to trade stocks or translate Japanese, we have yet to create an AI that, when shown a kitchen, can make a cup of tea.
Defining the moral boundaries
Because AI has to learn from data, this creates an ethical problem: what if the data is biased or incomplete? By the principle of ‘garbage in, garbage out’, it is likely to make bad decisions. If these are pricing recommendations, for example, then perhaps it’s no big deal, and it can safely learn from its mistakes.
As a member of the Esomar ethics committee, Jon Puleston, Lightspeed’s vice-president of innovation, has been discussing the implications of this. “The moral boundaries of marketing and research were quite clear in the era when a researcher was advising a marketer what newspaper to advertise in, or what car men over 50 like to drive,” he says.
“We now have the capabilities, with AI, to microtarget, not just by demographic, but by personality type and with highly customised pieces of communication and marketing strategies – which raises all sorts of potential ethical issues. AI decision-making algorithms can become discriminatory without careful consideration of how decisions are made, and it is difficult to bake human ethics into an algorithm.
Hetan Shah, executive director of the Royal Statistical Society, explains: “Let’s say you’ve got a recruitment algorithm and it has been trained using the data of all the people you’ve hired that you thought were good. Then, if your algorithm starts recruiting lots of old, white men, you have a problem.”
There are several initiatives to try to set up ethical frameworks, with inquiries currently by both houses of Parliament into how these might work. For decision support, it will be possible to continue to impose rules: for example, making price discrimination based on race illegal. For automatic decision-making, however, this is more complex and subtle. An AI can’t tell you why it is making a decision, so this probably implies that AIs will need to be regularly tested. Defining those tests – who would administer them, and what to do about the outcomes – will be a hard problem to solve.
AI takes longer than you think
One of the insights that AI has given us is just how difficult many ‘intelligence’ problems are to solve. While AIs in narrow, rules-based systems – for example, for playing chess – have been successful, AI research has taught us how complex human communication is. Recent chatbot and machine-translation breakthroughs, for example, are the result of half a century of research:
1950: Alan Turing creates the ‘Turing Test’ for AI. If a machine can trick 30% of humans into thinking it is a human being in five minutes of conversation, we can call it intelligent.
1954: IBM demonstrates machine translation from Russian to English. But the system has only 250 words, and is focused on chemistry.
1964: The first chatbot, called Eliza, is created at MIT.
1966: The US ALPAC committee publishes a report for the US government that concludes machine translation is more expensive, less accurate and slower than human translation.
1970: AI pioneer Marvin Minsky tells Life magazine that the problem is almost solved: “From three to eight years, we will have a machine with the general intelligence of an average human being.”
1997: Systran, after 29 years of development, launches Babel Fish, the first online translation engine.
2011: Google Brain launches, and rapidly improves translation accuracy by 2016.
2014: Eugene Goostman controversially passes the Turing Test. Critics point out that it is a chatbot posing as a 13-year-old Ukrainian boy with limited English.
2016: Microsoft’s Tay chatbot is released on social media. It quickly learns to make offensive racist remarks.
Building smart technologies – BY Lewis Reeves, CEO, Viga
AI within market research is more frequently discussed than it is executed, and is very much in its infancy. While cutting-edge tech is evolving constantly, AI’s application is still limited and many are also talking about its uses incorrectly.
However, from identifying or profiling through AI, to creating more interactive survey experiences and enhancing real-time results, the potential for AI is clear to see. I founded Viga two years ago, based on our proprietary tech, and we’ve developed this ever since. It underpins the speed, relevancy and cost-effectiveness of our delivery, but we value human-to-human interaction above all else. Key for us is building smart techniques into our processes, but ensuring there’s always a human and machine combination.
The crucial question for us is how to power AI in a model that has a human at the end. AI should make laborious tasks disappear, allowing people to offer context and nuance – things that are missed when you fully automate. Tech shouldn’t dilute the value, but augment it by making processes more efficient.
It’s very important to distinguish between automation and true AI. At Viga, we asked 500m questions last year – these were enabled by
AI. If we were merely making use of automation,
this figure would look closer to 10 surveys asked multiple times.
Our use of AI involves generating content, not pre-populating – for example, use of predictive question text. Just as your smartphone can predict the next word you’ll use, even if you’ve never written the sentence before, AI can predict the next question needed before it has been written. It may not be the sexiest function, but if it frees humans up from building questions, so they can have more time on the key elements of interface and interaction, it’s integral.
Our clients shouldn’t be left to interact with a machine. Tech is there for the hygiene elements; however, there’s always a person at the end of the line on a Sunday evening before a big Monday morning presentation, to ensure there’s never a ‘computer says no’ moment.
This article was first published in Issue 23 of Impact (October 2018 ).
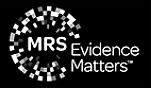
We hope you enjoyed this article.
Research Live is published by MRS.
The Market Research Society (MRS) exists to promote and protect the research sector, showcasing how research delivers impact for businesses and government.
Members of MRS enjoy many benefits including tailoured policy guidance, discounts on training and conferences, and access to member-only content.
For example, there's an archive of winning case studies from over a decade of MRS Awards.
Find out more about the benefits of joining MRS here.
0 Comments