Driving in automatic: How Jaguar Land Rover used artificial intelligence to track sentiment
Humans are experts at deciphering language and picking up the nuances in speech, and the meaning behind words. In the ever demanding world of brand tracking, can rigour and analysis be retained in research while increasing speed and reducing cost?
A partnership between research agency MM Eye and Jaguar Land Rover aimed to explore whether an artificial intelligence (AI) system could help uncover customer sentiment in answers to open-ended questions and save the brand time and money.
The project started in 2017 and was intended to help Jaguar’s brand health survey, examining customer views and feelings towards the company. Specifically, its goal was to explore whether long-form, verbatim responses to questions posed by the survey could be analysed by AI instead of human coders without losing the necessary level of detail and quality.
MM Eye has worked with Jaguar since 2010, and was involved with its brand health survey before the AI project. The survey has typically used stream-of-consciousness interviewing techniques to analyse consumers’ emotional engagement with the brand. The questions are open-ended, so are more likely to elicit a better understanding of people’s emotional connection with particular car brands.
“It’s like taking a look inside the consumer’s head and getting a much clearer picture of how they see the world,” explains Claire Catmull, head of continuous research at MM Eye. “This approach is simple to conduct, but rich in outcome.”
The brand health survey works by turning answers to the survey questions into data, using a forensic coding system that identifies and categorises words, descriptive attributes, and recounted experiences with the brand.
A single paragraph can provide numerous pieces of data, which are then also analysed for their emotional sentiment – whether they are said in a negative, positive or neutral way. This generates thousands of pieces of data, and the previous approach of using human coders to analyse the information collected took, on average, 53 weeks.
In 2017, MM Eye proposed changing the methodology behind the survey. It decided that an AI ‘cyborg’ – which would automate much of the analysis, with support from human coders – could help reduce the time and cost involved in the coding process, and it began scouting the market for an off-the-shelf tool.
However, MM Eye concluded that existing text-analytic packages were unable to deliver the model the companies wanted, so it began looking at how to make its own bespoke system.
The system had to have the necessary “granularity” to allocate codes to a clause or subsentence in consumers’ responses, according to Catmull – this would make the coding as accurate as possible, and similar to how a human would be able to analyse speech. It needed to be able to identify the correct sentiment used during a conversation and work with non-English languages.
“Our journey wasn’t a straightforward one – more a voyage of discovery that, when we embarked upon it, we didn’t know would be successful,” Catmull says. “We needed the end product to seamlessly transition so as not to disrupt years of data tracking; we needed the AI to match the granularity of our existing code frame, and we needed the AI to code the sentiment.”
Several approaches were considered, with the most promising being ‘StarSpace’, a general-purpose, neural model for efficient learning of entity embedding, used by Facebook to identify people’s interests and outlook. In January 2019, MM Eye reworked StarSpace to operate within the subsentences often analysed in the brand health survey. This, says Catmull, turned out to be a “breakthrough”.
“Tapping into expert advice gave us a significant step forward and the route into using the StarSpace approach to allocations. Another significant step came when we appreciated that some element of ‘human’ verification would still be required if we wanted to meet our goals.”
The new coding system is self-learning, so it can improve its analysis continuously, based on previous data. This is coupled with human oversight, so accuracy is maintained at pre-AI levels. Accuracy of the initial AI analysis of sentiment and meaning is at 70%, up from an initial 50% and with aims to increase this to 80%.
In the first year of using the AI system, there was a 33% reduction in coding costs, which the companies expect will drop further, and there has been a significant fall in the amount of time needed to complete the coding process.
Jaguar Land Rover has used the cost savings to expand the AI tool and are exploring how it could be used elsewhere in the business. For example, the recent Jaguar ‘Bend the Rules’ campaign was supported by the AI system.
Iulia Calin, brand and communication lead, global customer insights at Jaguar Land Rover, says: “The new coding system has helped us to have data much quicker, which meant we could have early discussions with the UK marketing team, for example, and our creative agency – and like this the ‘Bend the Rules’ campaign was born.
“The new coding process gave Jaguar Land Rover more time and money back that we have reinvested in other areas of the brand health study. The new AI has been tested successfully on a completely different internal project, and our aim is that this tool will be used widely within the business – whenever we have a lot of verbatims – in the next 12 months.”
This article was first published in the April 2021 issue of Impact.
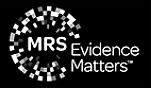
We hope you enjoyed this article.
Research Live is published by MRS.
The Market Research Society (MRS) exists to promote and protect the research sector, showcasing how research delivers impact for businesses and government.
Members of MRS enjoy many benefits including tailoured policy guidance, discounts on training and conferences, and access to member-only content.
For example, there's an archive of winning case studies from over a decade of MRS Awards.
Find out more about the benefits of joining MRS here.
0 Comments