Brain training
Communication is, in many ways, shifting from words to images. The ease of creating – and deleting – images via smartphones and other portable digital devices means that more than one trillion photos are now shared across Facebook, WhatsApp, Instagram and Snapchat every year.
The content of these images is of great interest to brands, because it can offer insight into the lives and aspirations of current and potential customers.
As this trend – and the amount of imagery – grows, there is more and more demand for automation of image processing. While machines are becoming more sophisticated, they are still a long way behind humans when it comes to tasks such as object recognition.
Not only is the pattern of errors made by current algorithms apparently quite different from those of humans performing the same task, but the algorithms are also reportedly easy to fool. Research has shown that, by changing an image – in a way imperceptible to human eyes – that has originally been classified correctly, a deep neural network can be tricked into labelling it as something else entirely.
Similarly, these networks can easily be tricked into thinking that images of TV static are objects – in some cases with more than 99% confidence.
In an attempt to make machine thinking more closely resemble that of a human brain, a team of academics at Harvard University – led by David Cox – has been looking into improving the process by using activity from human brains as a kind of training tool.
Cox’s team is investigating a ‘neurally weighted’ machine-learning approach, which involves feeding data from functional magnetic resonance imaging (fMRI) measurements of human brain activity into the process of an object-recognition learning algorithm.
According to the research paper, “the human brain is a natural frame of reference for machine learning, because it has evolved to operate with extraordinary efficiency and accuracy in complicated and ambiguous environments.” However, despite the brain having “long served as a source of inspiration for machine learning”, the paper claims “little effort has been made to directly use data collected from working brains as a guide for machine-learning algorithms”.
The study used data from fMRI scans taken while a subject viewed nearly 1,400 images, which included four different types of object: humans, animals, buildings and food. The team analysed how regions of the brain’s visual cortex responded to these images; the different objects had corresponding patterns of brain activity, with the strength of the signals indicating how difficult each image was to classify.
This information was then used to train machine-learning algorithms: if an algorithm made a mistake on an image that should have been easy to classify, it was penalised more heavily than if it made a mistake when classifying a ‘difficult’ image.
This meant the system was being told what information it should base its classifications on, to minimise errors. As a result, it performed better on images that were easily recognised by the brain and, in doing so, was learning to make decisions in a more human way.
The results revealed that training a basic image classifier with the fMRI data improved its accuracy by between 10% and 30% across the different categories.
Nick Gadsby – founder and principal of semiotics and cultural insight agency The Answer, which recently launched a method for analysing consumer visual language – believes that this approach could be a starting point for the relatively accurate tagging of large numbers of images.
“The system’s unique claim – that it infuses fMRI data from the brain of a human subject viewing images into the training process of a machine-learning algorithm – is testament to the distinctive way in which humans perceive and process visual information – and sensory information more generally – and, of course, make it meaningful in the process,” says Gadsby.
“Although the data from the study shows that even the most successful combination of algorithms and loss functions fell short of human accuracy, it does promise the development of an approach capable of near, if not absolute, accuracy.”
But Gadsby points out that, even at this advanced stage, a program capable of identifying the content of images at a commercial level would still need to take into account a much wider body of fMRI data. This includes differences in cultural perceptions, as studies comparing Western and East Asian processing have demonstrated.
The other consideration around machine learning based on models of the human brain, Gadsby says, is that it almost always treats human learning as a process based on individual trial-and-error experience. But in related fields, such as cognitive psychology and neuroscience, he adds, the emerging consensus is that humans are evolutionarily predisposed to social learning – so our perceptions are not necessarily based primarily on what is objectively out there, but rather by the way objects are collectively classified.
“While simple object recognition may present itself as a probable activity for individual classification, for the general field of machine learning to advance, much consideration needs to be devoted to modelling social learning,” Gadsby says.
The study also highlights the fact that – as the volume of visual research data increases – the research industry will need to become better informed about neuroscientific understanding of vision. This includes debates about the relationship between feed-forward, feed-backward and re-entrant models of visual processing, the dynamics of lower- and higher-level processing, and broader shifts in theories of perception toward predictive models.
Reference:
Using human brain activity to guide machine learning, by Ruth Fong, Walter Scheirer, David Cox on the Computing Research Repository (CoRR) arXiv:1703.05463
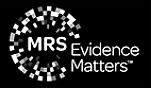
We hope you enjoyed this article.
Research Live is published by MRS.
The Market Research Society (MRS) exists to promote and protect the research sector, showcasing how research delivers impact for businesses and government.
Members of MRS enjoy many benefits including tailoured policy guidance, discounts on training and conferences, and access to member-only content.
For example, there's an archive of winning case studies from over a decade of MRS Awards.
Find out more about the benefits of joining MRS here.
0 Comments