The golden age of NLP?
Most data in this world is not neatly structured numerical data. The overwhelming majority of data surrounding us is (more or less messy) text data. And there is more of it every day. With an estimated 3.2 billion internet users worldwide, the amount of content generated every day is staggering. The English Wikipedia for instance, holds more than five million articles. If anyone wanted to publish a Wiki print edition it would require about 7500 volumes of 700 pages each.
According to various estimates, the amount of data we hold globally is doubling every 18-24 months. Driven by the introduction of Big Data technologies like Hadoop, enterprises nowadays store and process huge volumes of unstructured text data. Any internal document, report or even email now becomes data which potentially holds valuable insights – but only if it is properly analysed and cross-referenced with other internal or external data.
Text mining, text analytics, Natural Language Processing (NLP) – they have all been around for some time now. And if you tried some software or text mining services a couple of years ago, you might not have been very impressed by the results. Until relatively recently, text mining was rarely more than a process of automated or semi-automated coding, the extraction of single word phrases and the application of sentiment models which more often got it wrong than right.
But recent innovations in NLP, artificial intelligence and machine learning have vastly improved our abilities to structure, summarise and model large amounts of text data.
Using state-of-the-art topic modelling approaches, we can now automatically extract key themes from text data, which is so much more powerful than searching for key words. Speech tagging and other linguistic analysis has improved and now allows us to programmatically parse sentences to understand who refers to what and how. Sophisticated machine learning algorithms are able to ‘consider’ the context in which sentiment is expressed to detect sarcasm and irony.
The applications for NLP are numerous, ranging from Customer Service, New Product Development and Brand Reputation to Fraud Detection and Corporate Compliance. Each has its particular objectives, which in turn define was is required from NLP. But most importantly – it requires someone who knows how to choose and implement the right text mining solution for your particular application.
There is now a wide variety of text analytics software and cloud services on offer. Each of them provides a smaller or larger range of common text analytics technologies, such as information retrieval, named entity extraction, pattern analysis, summarisation and sentiment analysis.
However, I have never come across one that’s applicable to each and every possible case of text analytics. There is simply no such thing as ‘plug & play’ when it comes to NLP and text analytics. It requires expert knowledge in NLP and related technologies. Otherwise any text mining project risks disappointing the often huge expectations that have been set by glossy websites of the software vendors.
Frank Hedler is director advanced analytics at Simpson Carpenter.
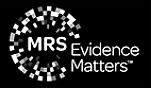
We hope you enjoyed this article.
Research Live is published by MRS.
The Market Research Society (MRS) exists to promote and protect the research sector, showcasing how research delivers impact for businesses and government.
Members of MRS enjoy many benefits including tailoured policy guidance, discounts on training and conferences, and access to member-only content.
For example, there's an archive of winning case studies from over a decade of MRS Awards.
Find out more about the benefits of joining MRS here.
0 Comments