Know your must-haves and delighters
One of my favourite models is KANO, which I have found many clients are not aware of, despite the fact that it has been around for a while.
It is in fact 20 years since Noriaki Kano, professor emeritus of the Tokyo University of Science, published the book Guide to TQM (Total Quality Management) in Service Industries. In it, he described the KANO framework and how it can help better understand how product and service features affect acquisition and retention.
Kano questioned the common belief that improving any attribute of a company’s product or service has an effect on uptake and satisfaction. Rather, he suggested that different attributes have different effects, and that it is essential to know the nature of this effect for each attribute in order to prioritise development efforts on things that have the greatest impact.
The Kano model distinguishes between essential and differentiating or delighting elements of products, services or marketing communications. It classifies each element into one of five categories:
- Must-haves or hygiene factors – these are elements that create dissatisfaction if not (fully) provided, but have no effect if they are present.
- Delighters – The opposite of must-haves. These are elements that customers do not expect, but have a positive effect if provided. The literal cherry on top. Delighters can be the key differentiators in a competitive environment.
- Satisfiers – These elements result in satisfaction when fulfilled and dissatisfaction when not fulfilled, i.e. where the principle of “the more the better” applies.
- Indifferent – This refers to aspects that are neither good nor bad, and that have no significant impact on either acquisition or retention.
- Reverse – These are elements that put customers off if present. For example, some customers appreciate all the bells and whistles, while others prefer the basic model of a product and will be dissatisfied if a product has too many extra features.
What excites me about the KANO framework is its adaptability, flexibility and simplicity.
Adaptability
The model can be applied to a range of fields, from customer satisfaction, over new product development to message optimisation to answer essential questions in each. What are the must-have messages we have to include in our communications? What product features can delight customers and provide a competitive edge? Which customer service touch-points need to be prioritised in order to maximise customer loyalty?
Flexibility
KANO is agnostic to the data input. How we identify the nature of each attribute is totally up to us. We can use sales and CRM data, or survey data, or we combine different data sources. We can derive the KANO category of each element through statistical analysis of customer satisfaction surveys, or we use trade-off exercises like conjoint or max diff to and apply the analysis on part-worth utilities.
Simplicity
KANO provides an intuitive framework, using simple language to describe the nature of each element, making it easy to communicate even to less research savvy audiences.
And there is yet another reason to consider KANO in particular for product optimisation. By introduction of the concept of ‘must-haves' and their opposite, the ‘reverse’ features, KANO accounts for non-compensatory behaviour, i.e. the lack of a must-have feature cannot be made good by adding more or improving other features.
In this respect, KANO is superior to conventional conjoint approaches which in principle allow the negative impact (or utility) of a feature to be compensated by adding more and more desirable features. After applying the KANO framework and identifying the nature of the impact of each element, it is easy to set up simulations to model the likely uptake of different product or service configurations.
Frank Hedler is director of advanced analytics at Simpson Carpenter
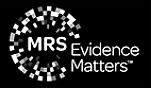
We hope you enjoyed this article.
Research Live is published by MRS.
The Market Research Society (MRS) exists to promote and protect the research sector, showcasing how research delivers impact for businesses and government.
Members of MRS enjoy many benefits including tailoured policy guidance, discounts on training and conferences, and access to member-only content.
For example, there's an archive of winning case studies from over a decade of MRS Awards.
Find out more about the benefits of joining MRS here.
0 Comments