Synthetic data will never paint the whole picture
Can synthetic data help us to bridge gaps in consumer understanding? This appears to be the question on many researchers’ lips right now.
Sadly, the reality is that there is no easy answer to this question. As ever, it’s not black and white, but rather shades of grey. A layering of techniques is needed to generate the most accurate insights and while artificial intelligence (AI), synthetic data and large language models (LLMs) can all help, we need to keep humans at the centre of the process and include psychographic and behavioural techniques, even as we harness the undoubted potential of technology and data science.
Indeed, the use of psychographic algorithms can help us to understand and predict emotional needs and drivers – something that many claim that synthetic data sources lack – and, to this end, there’s no doubt whatsoever that AI has a huge role to play.
For instance, by creating a digital twin through the collection of key psychometric data points and modelling behaviour and sentiments, we can achieve truly meaningful and increasingly accurate profiling. Such models can help us to create comprehensive personas which evolve alongside changing consumer behaviours and preferences, in real time.
The benefits of real-time digital twins
These kinds of models can help us to create comprehensive personas which evolve alongside changing consumer behaviours and preferences, in real time. Our insights benefit from in-the-moment understanding, with technology helping to reveal previously hidden consumer truths and behavioural patterns, such as a luxury car buyer’s affinity for seafood or a health insurance customer’s love for DIY projects. This has the potential to drive deep connections with audiences through targeted, hyper-personalised communications.
No longer do researchers have to settle for gaps and lags in consumer insights. Moreover, there’s no longer any excuse for relying on fragmented, unreliable external data sources. Today, the technology exists to help us generate accurate insights directly from consumer interactions. Alongside this, we can harness a range of statistical approaches, research methodologies, and data science techniques to bring businesses ever closer to their customers.
For some time, businesses have found that their data sources are outdated, passive and disconnected from the reality of how individuals think and behave. Yet by bringing data points together with psychometric science – powered by proprietary algorithms unique to a business – it’s possible to harness transformative, consented zero-party data that evolves with every interaction, enabling businesses to see and understand their audience in more granular detail.
Synthetic data’s role is limited
Take the news a few weeks ago of Mostly AI’s synthetic text tool designed to help enterprises unlock value from their proprietary datasets without privacy concerns. It’s true that, as more companies invest in generative AI for bespoke use cases and products, proprietary data is becoming increasingly important to training LLMs.
Unlike ChatGPT, which was trained on billions of public data points – emails, scripts, social media, papers – scraped from the internet, enterprise generative AI often needs to be specified to that business’ customer data. In the words of Mostly AI chief executive officer Tobias Hann: “To harness high-quality, proprietary data, which offers far greater value and potential than the residual public data currently being used, global enterprises must take the leap and leverage both structured and unstructured synthetic data.”
But synthetic data remains only a part of the puzzle. We must remember, too, that consumers don’t always make the logical choices a computer might expect.
It’s clear that there are both opportunities and challenges ahead, especially when it comes to assessing the use cases for emergent techniques. While synthetic data can increase sample size, at lower cost and with greater speed, it can also bring bias or distortion, as can AI more broadly.
Layering new technologies and tools delivers the best results
Researchers must beware of the hype, or the latest shiny new toy. Insead, they must seek to combine multiple datasets and technologies – thinking always about how to implement AI responsibly and with precision and accuracy to transform the industry, through safe, value-adding implementations, which may or may not include synthetic data. The most experienced are already well versed in combining proprietary analytics frameworks, selecting the right tools for the job in hand – AI or otherwise.
Mark Ritson has spoken of ‘the era of synthetic data’, rightly pointing to significant implications for the industry. But it’s not human versus AI nor ‘qualitative’ versus ‘quantitative’ that we must focus on; making rudimentary and unhelpful distinctions, or blanket assertions. We must harness a layering of techniques in this fluid, evolving system in which breakthroughs are already well underway, with AI improving research processes, reducing time and costs, and enhancing analysis.
From our first rudimentary attempts at harnessing ChatGPT to develop hypotheses, AI has given researchers new powers, driving efficiencies in businesses. Synthetic data forms only a small part of this jigsaw, albeit one which is most in the spotlight at this time, and we must seek to harness a variety of research methods to drive participant engagement.
In a world of choice, with a myriad of options always at our fingertips, real people must be, and feel, listened to and heard. Conversational methodologies help, to this end, and we must invite real-life audiences to participate as co-creators of this brave new world.
Oscar Carlsson is strategy advisor at Jishi
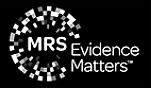
We hope you enjoyed this article.
Research Live is published by MRS.
The Market Research Society (MRS) exists to promote and protect the research sector, showcasing how research delivers impact for businesses and government.
Members of MRS enjoy many benefits including tailoured policy guidance, discounts on training and conferences, and access to member-only content.
For example, there's an archive of winning case studies from over a decade of MRS Awards.
Find out more about the benefits of joining MRS here.
0 Comments