Rethinking the questionnaire
Falling levels of respondent engagement is a big deal. Not all respondents toil diligently though large grid questions nor reflect deeply upon bank after bank of attitudinal agreement statements, asked by anonymous brands.
Customer research, unlike pure market research, is less vulnerable. Here, light touch questionnaires befit a desirable two-way dialogue. It is a formal opportunity to share experiences and nudge their chosen brands toward greater relevance, responsiveness and value. Nevertheless, patience with long, wandering questionnaires that try to cover all the bases, wears thin.
Despite best efforts, there is a limit to how absorbing questionnaires can be. There remains an irreconcilable disconnect among rigorous questionnaires designed to tease out insight, the metrics a business requires and what respondents are prepared to offer. Ultimately, research needs to be on respondents’ own terms or data quality suffers. With faulty information, organisations lose touch then flag and fail.
Open-ended questioning, on the other hand, allows respondents to do exactly what they are co-opted for – to have their say and to do so without restraint. This is the holy covenant, which is frequently broken. Freedom to express views is buried, reserved for the ‘anything else?’ – a researcher’s catchall gambit.
Open-ended questioning is rarely prominent. Practised respondents acknowledge this and as a result, typically offer one-word responses. Some, already in the throes of disbelief and frustration, bash their keyboard in outright rebellion.
To be fair, until recently, no one really knew what to do with text, save for word counts. Pretty word clouds were nice to have but hardly insightful and so hurriedly discarded in favour of the easily quantifiable.
It is now time to embrace next generation Natural Language Processing (NLP) – which allows for deeper analyses at scale by identifying patterns of words. It is agile enough to operate alongside research objectives and stakeholder expectation. It filters out noise, adds numerical structure, draws relationships and lays the bedrock for sophisticated modelling. The data is rich. With subtleties retained, it always surprises.
The internet is flooded with spontaneous, volunteered feedback: winding commentaries, praise, complaints and reviews with detail to challenge War and Peace. Sharing experiences is a big thing right now and researchers are missing out. Under the right conditions, customers are anything but cagey. Rants are golden.
This zeitgeist begs us to replace 35 minutes of questionnaire monotony to make the most of respondents’ attention and intention. The economics of sample management also encourage this.
Don’t bother with, ‘So what did you think about this advert?’ Rather, ‘What do you think the brand was trying to convey in this campaign?’ Similarly, ‘If you were the branch manager, how would you go about improving things on your first day?’
Many brilliant research programmes have already embraced this and are benefiting from compact and frequent, earnest, to-the-point questioning. Once the covenant is restored, so too does an attention span for a few grid questions.
Let’s also not lose sight that data points, no matter how rich and interesting, must be reliable and inform business metrics, otherwise they are merely anecdotal. Text needs to be controlled for verbosity and calibrated. We desperately need to recruit more NLP experts to work with unstructured data with the same relish and fluency that our statisticians reserve for grids and banks.
In doing so, the public perception of surveys is rehabilitated by forcing questionnaires into step with a sharing society that is willing to answer the mission-critical questions that big data will be forever sorely incapable of posing. This is a society that places a greater importance on their time, data and opinion and that demands personalised, unfettered relationships with brands.
Before we can achieve this, we must revisit our own bizarre predilections about survey research, chief of which is the unconscionable risk of surrendering wholesale control of results to respondents. Perish the thought.
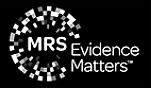
We hope you enjoyed this article.
Research Live is published by MRS.
The Market Research Society (MRS) exists to promote and protect the research sector, showcasing how research delivers impact for businesses and government.
Members of MRS enjoy many benefits including tailoured policy guidance, discounts on training and conferences, and access to member-only content.
For example, there's an archive of winning case studies from over a decade of MRS Awards.
Find out more about the benefits of joining MRS here.
2 Comments
Saul Dobney
7 years ago
Open-ended data provides the best insights in small nuggets of wisdom. That's why it's always worth reading the open-ends in a survey in full (a survey of 1,000 people will have around 600 open-ends of which around 10-15 will be particularly pertinent and insightful, but many of the quickly typed remainder will aggregate up to 'nice'). Obviously, on-line text mining will have comments in thousands but then you run into problems of rep, bots, shills. And comments come in discussions which reduces repetition - you don't get hundreds of people repeating the same point in a discussion, though they might like or uptick to show agreement. Text mining will therefore show the range of opinions and give excellent detail, but might not show the representative opinion. That's not to say it isn't useful, but rather you can't substitute this one form of data directly for the other.
Like Reply Report
Rick Harris - CEO, Customer Faithful
7 years ago
Hugely important topic and well balanced piece, Ryan :-) Also @Saul - agree that text analytics is not a replacement for closed questions. In practice, I like to offer optional 'open-end' comments boxes alongside a closed question - so that participants can choose to describe why they scored a "strongly agree". Again - this is not seeking representation, but can improve interpretation. The biggest criticism of quant surveys is that they offer little explanation of "why?" Text analytics can play a role here, especially if the research design allows for some qual F2F afterwards, to look further into some of the arising themes.
Like Reply Report