Benchmark the buzz
The general consensus seems to be “a bit of both”, with a leaning towards qual; I wouldn’t disagree with that, but there’s immense potential for more quant-focused approaches. However, there are plenty of challenges, not least the best way to report on the numbers.
Numeric values in isolation are meaningless without some sort of context to put them in. Let’s say a brand has a hefty advertising campaign (online or offline) which results in a 20% increase in social media buzz. A client might rightly ask “Does that mean anything?” Similarly, following an adverse event (and there are no shortage of corporate comms nightmares at the moment), it’s easy to say glibly that social media conversational volumes have doubled, and it’s all negative. But what clients should be asking their agencies – whether they are research, reputation management or social media consultancies – is “What is the significance of this?”
“Numeric values in isolation are meaningless without some sort of context to put them in. Clients should be asking their agencies ‘What is the significance of this?’”
When tracking reputational issues, for instance, we can measure the presence and persistence of an issue or event based on how many people are talking relative to normal, and at what time the buzz reverts to normal background levels of conversation. When LinkedIn admitted recently that six million passwords had been leaked, daily social media mentions jumped around seven-fold compared to normal levels. But LinkedIn is a large, mainstream tech brand with a high level of background chatter. Other organisations might experience sharper spikes.
The key challenge here is to establish a system of norms and benchmarks which is relevant to the sector and market in question. Benchmarking can be done over time, or against competitors, or against previous events. But make sure you’re measuring like for like.
Volumes of social media conversations are increasing all the time, although not at a uniform rate, so a system of normalisation should be considered if you’re working with a longitudinal tracker to make sure that you are measuring the share of social media conversations rather than absolute numbers. There are also more technical considerations, like Firehose-vs-non-Firehose data.
Ultimately, though, the best way to augment quantitative or longitudinal social media research results is to link them to another form of research, such as a brand health tracker – this way the tracker can validate the social listening. The reverse is also true: if the wording of the tracking survey is very generic, metrics which might otherwise be hard to explain can be brought to life with social listening which coincides with fieldwork dates. When taking a sample of social media data and coding manually, it can become immediately apparent when compared to a traditional tracker that the overall brand sentiment might be very different to the numbers in the survey data, and different brand attributes might be being talked about.
However, this is where it’s imperative to think about relative, rather than absolute numbers. How are the metrics changing over time, and how do they compare to key competitors? When digging deeper into comparable attributes, what is being said?
Social listening certainly won’t answer all your prayers. But with a suitably creative approach, combined with some robust benchmarks, it can really bring results from traditional techniques to life.
Eoghan O’Neill is a social listening analyst at Ipsos Mori
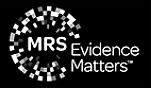
We hope you enjoyed this article.
Research Live is published by MRS.
The Market Research Society (MRS) exists to promote and protect the research sector, showcasing how research delivers impact for businesses and government.
Members of MRS enjoy many benefits including tailoured policy guidance, discounts on training and conferences, and access to member-only content.
For example, there's an archive of winning case studies from over a decade of MRS Awards.
Find out more about the benefits of joining MRS here.
1 Comment
Annie Pettit
13 years ago
I always enjoy the sample size discussions. Wow! My brand is getting more and more mentions every day! Well, of course it is. EVERY brand is getting more and more mentions every day. The trick is knowing when your mentions are growing at a rate that is different than your competitors. As for qual vs quant, this is a very simple issue for me. When we analyze surveys, we always discuss them in context. We consider responses to the open-ended questions, we pull together results from several related quant questions. We never simply report on one question in a silo. And the same holds for social media research. Any result, whether quant or qual, needs to be discussed in conjunction with other related data points. Qual helps to flush out quant results. Quant results help to ground qual results. It always comes down to quality work done by skilled researchers.
Like Reply Report