And the winner of Euro 2016 will be…
Euro 2016 is well and truly underway. And for the third tournament running – including Euro 2012 and the 2014 World Cup – we are being offered various predictions based on statistical models. The analysts at Goldman Sachs published their Euro 2016 model last week, predicting host nation France as the winner with a probability of 23%, beating Spain in the final.
The model of Blue Yonder, a predictive analytics company, comes to the same result, with an even higher probability ( 34%) for France to clinch the title – whereas the Bing prediction model punts Germany as the tournament’s most likely winner.
Interestingly, none of these predictions is based on opinions of professionals in the game, punters nor commentators. Instead, they have all been created using machine learning (ML) algorithms. In the case of Blue Yonder, analysts used the NeuroBayes algorithm, an advanced neural network model that was initially developed to predict particle collisions in the Large Hadron Collider.
This sounds impressive. Using an algorithm that has been developed to help answer some of the fundamental open questions in physics, such as the structure of space and time, and applying this algorithm to predict the outcome of a football tournament.....armed with such advanced technology, the prediction model surely cannot fail?
The recent buzz around ML algorithms and Artificial Intelligence (AI) certainly suggests that nearly everything is possible: automation, optimisation and prediction of almost every aspect of our daily lives. There have even been articles claiming that the next generation of algorithms would make data scientists redundant, and provide insights and predictions from data without any need to formulate a model.
So can we really just feed past football results into, say, a Random Forest algorithm, and receive accurate predictions for the winner of Euro 2016? It cannot be as simple as this; otherwise it would not need a team of highly skilled analysts at Goldman Sachs to create a football prediction model, let alone a model to predict the financial markets.
The accuracy of prediction models does not depend wholly on the particular algorithm that is being used. No, the art of modelling and prediction is called Feature Selection, i.e. deciding which information is fed into the model, and how this information is derived from available data.
The results of past football matches are one such feature that we might enter into the model. But we can assume that the older these results are, the less relevant they will be in predicting success today. Hence, we would weigh more recent results higher than older ones when feeding this information into the model.
We could further refine this feature and add information about the strength of the opposition against whom these past results have been achieved, by e.g. using the official FIFA ranking of the teams, expressed as the difference in their ranking before the match. Then we could think about including information about the squad of each team and possibly injured players. In terms of data this could be expressed through the average player rating for each team, as captured by statistics services like OPTA.
There are numerous ways of going about a model to predict the outcome of football matches. Apparently, Bing uses among other data the content of thousands of football blogs that discuss recent form and prospects of players and teams to succeed at the Euros. This adds a crowd sourcing element to it, where the crowd is a large, highly engaged and knowledgeable group of football fans and experts. Bing too uses past results, team rankings and similar quantitative data, but combines this with very dense information from qualitative sources.
Incidentally, Goldman Sachs’ model predicted in 2014 that Brazil would win the World Cup with a probability of more than 50% – which is a very high certainty given that 32 teams started into the tournament. At the end Germany won the title, beating Brazil in the semi-final, as was correctly predicted by Bing.
Any predictions on who will get it right this year? Whatever happens – enjoy the football.
Frank Hedler is director advanced analytics at Simpson Carpenter
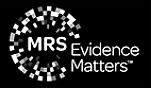
We hope you enjoyed this article.
Research Live is published by MRS.
The Market Research Society (MRS) exists to promote and protect the research sector, showcasing how research delivers impact for businesses and government.
Members of MRS enjoy many benefits including tailoured policy guidance, discounts on training and conferences, and access to member-only content.
For example, there's an archive of winning case studies from over a decade of MRS Awards.
Find out more about the benefits of joining MRS here.
1 Comment
matt Draper
9 years ago
and then Germany win on penalties
Like Reply Report