Rory Sutherland: All else being equal
I don’t know the answer to this question, but I would like your opinion. When asked on a form to state your religion or ethnicity, should you leave the section blank? Or might it be better to lie, or answer ‘other’? Or would it be better to tell the truth? Under perfect conditions, I would, of course, tell the truth. The problem is, perfect conditions never exist.
And here’s my issue. It seems to me an inarguable law that there must be far more semi-competent or indifferent statisticians among the population than there are great statisticians. If we assume a normal distribution of statistical competence, there will be a left-hand tail of people who are shockingly bad at assessing and understanding statistics, a large rump in the middle of people who are quite poor, then an equally large group of people who are confident enough to be dangerous. Tailing off towards the right will be a tiny number of true experts.
I’m not worried by the people who are atrocious at statistics. When they are wrong, it is easy for many people to spot and correct their errors. What worries me is the profusion of GCSE-level statisticians, because it seems to me a peculiar property of statistical understanding that high, but not spectacular, ability may be worse than none. In statistical questions, it is possible to be confidently and monumentally wrong, yet for the fallaciousness of your inference to be invisible to large groups of otherwise educated people – especially yourself. Here, a little learning really is a dangerous thing. Take the OJ Simpson trial:
“When the prosecution presented evidence that Simpson had been violent toward his wife, the defence argued that there was only one woman murdered for every 2,500 women who were subjected to spousal abuse, hence any history of Simpson being violent toward his wife was ‘irrelevant to the trial’.
“Yet, according to Gerd Gigerenzer, the correct probability requires the wider context — that Simpson’s wife had not only been subjected to domestic violence, but rather subjected to domestic violence (by Simpson) and killed (by someone). Gigerenzer writes ‘the chances that a batterer actually murdered his partner, given that she has been killed, is about eight in nine, or approximately 90%’. While most cases of spousal abuse do not end in murder, most cases of murder where there is a history of spousal abuse were committed by the spouse.”
I don’t suppose one person in a hundred would instinctively understand this distinction without having it explained. No-one in the courtroom challenged it. Yet the subtle distinction here affects the jurors’ likely inference by a factor of more than 2,200 – from a 0.04% implication of guilt to perhaps 89%.
Wider context also matters in the assessment of DNA evidence. If one in 200,000 people, including the suspect, shares DNA with the perpetrator, you have to consider how that DNA was first used. If it was used to find a suspect (perhaps by trawling a database of two million people) it has little value. If, on the other hand, someone seen fleeing the scene is arrested and found to have such a match, it adds to a reasonable presumption of guilt. Contextual blindness of this kind led to the unsafe convictions of Sally Clark in the UK and Lucia de Berk in the Netherlands.
Here’s why I am slightly alarmed by surveys routinely containing questions about ethnicity or religion, but that fail to collect enough information to provide wider context. For every valuable finding that emerges, we may end up with 10 spurious findings – spurious correlations, or those that are blind to the many confounding variables that simply aren’t collected.
There is, for instance, a significant correlation between your own personal success and the number of books your parents owned. I have never been asked for that information on a form. This is not to deny racial inequality or bias. It is simply a question of understanding it better. To lump together someone who arrives in Britain as a newly qualified surgeon and someone who arrives in later life with no qualifications simply because they share skin colour is not illuminating – it is misleading. Our selective collection of data risks doing exactly that. The act of averaging also risks blinding us to areas where racial bias is most acute.
Data broken down by race and nothing else will lead us to believe that all inequality proceeds from overt or unconscious bias, while leaving unseen the influence of other things (social networks in particular) that may play an even more powerful role in perpetuating inequality. The practice is largely well intentioned. Whether it is helpful is a different question.
This article is from the July 2022 edition of Impact magazine.
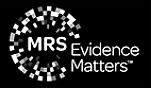
We hope you enjoyed this article.
Research Live is published by MRS.
The Market Research Society (MRS) exists to promote and protect the research sector, showcasing how research delivers impact for businesses and government.
Members of MRS enjoy many benefits including tailoured policy guidance, discounts on training and conferences, and access to member-only content.
For example, there's an archive of winning case studies from over a decade of MRS Awards.
Find out more about the benefits of joining MRS here.
0 Comments