The true face of quality
Somewhere along the way ‘quality in online research’ came to mean the quality of online panels. At this year’s Online Research Methods conference James Sallows, vice president of EMEA client operations with Lightspeed Research, showed a slide with a crosshair targeting the logos of Lightspeed, Toluna, SSI, GMI and Research Now. It was the kind of presentation you might expect if Sarah Palin were a clientside researcher.
“Where the focus in survey research has often been on minimising sampling error, it should instead be on minimising all types of survey error”
The panel providers are right to feel under pressure. Since 2006, when Kim Dedeker, then of P&G, famously declared that results from online panels were widely inconsistent, panel companies have been criticised for the practices of many of their panellists, who are often more motivated by incentives than by the desire to provide honest feedback. Industry initiatives have been undertaken to address panel quality by organisations including AAPOR, ARF and the ISO, and tools designed to ensure data quality have been introduced by panel providers and technology firms.
But the quality of online quantitative research is not just the panel companies’ problem. Market researchers have focused on panel quality because it is ultimately outside their control. They fret about it. They lose sleep over it. They wonder if they are getting the honest opinions of American and European consumers, or of workers in a sweatshop in Asia who are just doing it for the incentives.
Researchers routinely overlook what is within their control: the quality of the survey instrument itself. In fact, Sallows noted that of his 800 clients only one had a ‘gatekeeper’ who reviewed questionnaires for quality, to make sure questions were asked unambiguously, with correct use of scales and with an eye on the experience of respondents.
Just as online researchers focus on panel quality as the most important issue affecting reliability, survey researchers in general focus too often on margin of error as the most important sign of quality. One of AAPOR’s contributions to the debate, in its report on online panels, has been to focus on the total effect of all errors that can arise at all stages of creating, fielding and analysing a survey. This helps broaden our approach to improving the quality of online quantitative research overall, not just the fieldwork.
Herbert Weisberg writes in The Total Survey Error Approach: “It was always recognised that there are several types of error involved in a survey, but the greatest emphasis was on the type that is easiest to estimate statistically: sampling error… However, sampling error is just the tip of the iceberg in surveys.”
Weisberg discusses three groups of errors, each with three categories of error: respondent selection errors, response accuracy issues and survey administration errors. It is important to minimise all these if we are to maximise the quality of online research.
Respondent selection errors
Sampling error is the error that arises from surveying a sample of the population rather than the entire population. Measurable and easily calculated for probability samples, this error can be minimised simply by increasing sample size. It is unmeasurable for non-probability samples which means it should not be reported for most online surveys. Researchers shouldn’t use non-probability samples when they need to estimate the occurrence of attributes or behaviours in the total population. As AAPOR has stated elsewhere, “the reporting of a margin of sampling error associated with an opt-in or self-identified sample (that is, in a survey or poll where respondents are self-selecting) is misleading.” Non-probability panels and website intercept surveys are subject to sampling error, but the range of that error cannot be calculated.
Coverage error is the error that arises when the sampling approach is not representative of the target population. For European and North American panels, the lack of internet usage in approximately a third of households means that a large group is excluded from the sample, with certain subgroups excluded disproportionately (e.g. non-whites, the elderly, the poor, the rich, the unmarried, the less educated, those with less civic involvement). Organisations surveying house lists need to take particular care that they understand how those house lists differ in coverage from the broader constituencies they serve. For those seeking to use panels to represent the internet-using population, panellists will differ from other online users in being more highly educated, and may participate only in surveys of greater topical interest to themselves. Recruitment strategies for non-probability panels (for instance partnering with specific websites) may also introduce coverage error for specific target groups. In panels that use priority routers to siphon off frequently targeted panellists to other studies (doctors or IT professionals for instance), the resulting routing bias may cause coverage errors. This error source is especially troublesome for those seeking results that are replicable within a panel.
Unit non-response error is the bias introduced when individuals invited to take the survey do not take the survey. Of course, you never get to find out how non-responders might differ from respondents. To minimise this error, researchers should send repeated reminders to non-responders to take the survey, until a response rate of at least 20% is achieved; at the threshold, the effect of non-response has been suitably minimised. The self-selection bias inherent in recruitment to open non-probability panels is a source of error: non-response during panel recruitment is enormous, with one study (Alvarez et al, 2003 ) observing that only 6% of clickthroughs to a panel invitation completed the full panel registration process, resulting in only one panellist signing up for every 5,000 banner ad impressions. Probability-sampled panels avoid this source of error by using detailed external selection criteria with a rigorous and extended process to attempt to convince identified households or individuals to join the panel.
Response accuracy issues
These errors concern the difference between what is reported and the true response.
Item non-response error is the error introduced when respondents don’t answer questions at all or select choices such as ‘Don’t know’. For questions where a response isn’t mandatory, survey authors should exclude ‘Don’t know’ and ‘No opinion’ as choices when presenting scales.
Measurement error due to respondents is the error occurring from dishonest responses, responses that aren’t fully thought through (satisficing) and from poorly written survey instruments which can lead to acquiescence bias and other questionnaire design effects. Gatekeepers for questionnaire design should review and shorten questionnaires to reduce this. Project managers should avoid incentive strategies that might lead to abuse. The desire to maximise incentives leads some panellists to over-report product ownership and purchasing authority to qualify for more surveys, meaning it may be necessary to better hide the purpose of screener questions, which are often transparent in revealing the type of respondents sought or not sought.
Measurement error due to interviewer is the error that can be introduced when administering the questionnaire. Interviewer effects are rarely a problem with online quantitative studies, although some firms are now having online surveys transition to live chats with interviewers after a screener or profile section of the survey is completed.
Survey administration issues
Post-survey error – These are the mistakes or artefacts that arise from processing and analysing the survey data. A common source of post-survey error is differences in coding open-ended responses by different coders. The weighting of data derived from online panels is another source of error. While weighting has enabled marketing research firms to differentiate themselves from one another, the academic literature has yet to validate a weighting scheme for general market research (according to AAPOR’s report). Only a few narrow domains, such as political polling, have demonstrated greater accuracy from using weighting.
Mode effects – These are the effects introduced by the type of survey conducted. Social desirability bias and satisficing appear to be reduced in online research, but as some online survey authors seek to introduce a more human element to their questionnaires, with photos or avatars of virtual interviewers, they actually increase social desirability bias, taking on some of the mode effects of personal interviewing.
Compatibility effects – The difficulty of comparing surveys done at different times by different groups using different methods. Researchers must always be cautious about contrasting findings from different types of surveys. The same questionnaire fielded to multiple non-probability panels often shows wide variances in answers, as the recruitment and quality practices differ significantly from panel provider to panel provider.
All things considered
Where the focus in survey research has often been on minimising sampling error, the focus should instead be on minimising all types of survey error. The Total Survey Error approach is a comprehensive method for doing so.
Some criticise this approach because the components are correlated and can cancel one another out. For these critics it is simply a list of possible problems, most of which can’t be quantified. This is an understandable reaction given that Total Survey Error was formulated in response to a focus on sampling error. Perhaps it’s more helpful to talk about ‘total survey quality’, focusing on potential sources of error and seeking to minimise or eliminate them.
To improve the quality of online quantitative research we need to embrace the entire life cycle of survey creation, fieldwork and analysis. Panel providers shouldn’t be in our crosshairs. As Sarah Palin might say if she were a market researcher, we each need to take on more personal responsibility for the quality of our online research.
Jeffrey Henning was one of the co-founders of EFM business Perseus, which merged with WebSurveyor in 2006 to become Vovici. He previously worked with BIS Strategic Decisions (now part of Forrester) in the US and Europe, managing survey research projects for technology firms. He is a former columnist for Computerworld, and blogs at blog.vovici.com
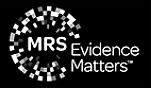
We hope you enjoyed this article.
Research Live is published by MRS.
The Market Research Society (MRS) exists to promote and protect the research sector, showcasing how research delivers impact for businesses and government.
Members of MRS enjoy many benefits including tailoured policy guidance, discounts on training and conferences, and access to member-only content.
For example, there's an archive of winning case studies from over a decade of MRS Awards.
Find out more about the benefits of joining MRS here.
1 Comment
Nallan Suresh
14 years ago
Great article, Jeffrey. I couldn't agree with you more on the need to look at error from all aspects and not just relegate it to the panel companies as their responsibilty. Over the past year, we at the Markettools TrueSample team presented two papers at CASRO on precisely those topics - the first one, also published in Quirks last year, was on designing surveys better (using our SurveyScore and lessons learned from past good surveys) to get better data quality, and the second was on identifying and removing unengaged respondents - basically underscoring the need to approach the problem from both sides. All of the arguments you make above seem very intuitive and quite a propos. However, it does not hurt to reiterate these points as the importance of this total approach cannot be understated. My recent blog also shows evidence of several causes of errors in case you are interested (http://www.markettools.com/blog/online-research-data-quality-problem-it-respondent-or-survey). Regards, Suresh MarketTools
Like Reply Report