Rise of the machines
A session at this year’s Market Research Society (MRS) Annual Conference explored the likelihood of the industry’s ‘curious minds’ being replaced by ‘curious computers’. In other words: what impact is artificial intelligence likely to have on market research?
According to a show of hands, half of the session attendees didn’t believe that their job would eventually be taken by a computer, a third were unsure, but the remaining (about 18%) did think that there was a chance that they could become ‘professionally obsolete’.
It would be interesting to know how many of those voting had heard of the EgoNet project, a predictive network model that has demonstrated the ability to predict which objects a person might be interested in, based on a database of annotated video footage.
EgoNet is the brainchild of Gedas Bertasius, a PhD student in the department of Computer and Information Science at the University of Pennsylvania, US. In EgoNet’s initial development, two students at the university were asked to wear two GoPro video cameras and film first-person views of their daily lives, then annotate the videos frame-by-frame, to show where their attention was focused at any one time.
“By mounting first-person GoPro cameras on people, we allow the machines to see almost exactly what that person is seeing,” says Bertasius. “This, in turn, allows the machine to tap indirectly into the human mind.”
The two cameras, along with the annotations, generated information for EgoNet’s two ‘neural networks’: the semantic gaze pathway, which identifies objects that grab the attention; and the 3D spatial pathway, which focuses on the object’s size and position relative to the camera-wearer, as well as object characteristics that might affect how a person would interact with it, such as the handle on a mug.
EgoNet is then able to take the information from these pathways, add another layer that integrates the two together (the joint pathway) and use these to predict behaviour via a ‘per-pixel action-object likelihood map’. The predictions from the three pathways are complementary – one can correct the mistakes made by another.
The team has demonstrated the ability of EgoNet to predict behaviour, and its superiority to other prediction methods, via quantitative and qualitative evaluation. The qualitative evaluation, which involved predicting action-objects in YouTube videos, even demonstrated the network’s ability to predict these interactions in novel scenes (i.e ones that don’t feature in EgoNet’s database) and from a non-human viewpoint (one of the videos was filmed from a dog’s perspective).
The researchers suggest that EgoNet could have applications ranging from the diagnosis of behavioural disorders in children, to aiding the acquisition of dexterous hand skills. But Bertasius believes it could also be used in market research.
“There are all sorts of visual appearance factors that could increase the probability of a person buying a specific product,” he says. “Of course, these are all hypothetical scenarios, and currently it’s challenging to validate any of them in practice.
“However, my belief is that by collecting first-person data that records these interactions – such as what people purchase in the shops – we could make the machine learn some of these subconscious behaviour patterns from the data and then use it to target the consumers more effectively.”
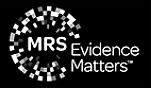
We hope you enjoyed this article.
Research Live is published by MRS.
The Market Research Society (MRS) exists to promote and protect the research sector, showcasing how research delivers impact for businesses and government.
Members of MRS enjoy many benefits including tailoured policy guidance, discounts on training and conferences, and access to member-only content.
For example, there's an archive of winning case studies from over a decade of MRS Awards.
Find out more about the benefits of joining MRS here.
0 Comments