Paddling the data lake
Most of us, by now, will have heard of the infamous ‘data lake’ – another annoying piece of jargon being thrown about by data scientists, data analysts, business intelligence analysts, data magicians, or whatever other crazy job titles you might have come across.
It seems like we’re always talking in metaphors nowadays, and the data lake is no exception. If you’re dealing with anything vaguely resembling data, you’ll struggle to avoid it. Personally, I don’t like it.
First, it’s completely unhelpful for those who don’t work with data or haven’t come across it before – if you’re one of those people, it essentially means ‘a heck of a lot of data’ (enough to fill a lake, I guess). Second, I think it conveys a desperate image of a huge expanse of water, unable to see land, being stuck, lost and forlorn in a never-ending sea of doubts. This isn’t the ideal picture we want to create of our industry, the people working in it, or the problems we’re solving.
The more time passes, the more complicated our industry becomes to the innocent bystander. Suddenly, what was just plain old boring statistics becomes a new, shiny, ‘trendy’ field of data science. We start throwing words around that essentially don’t mean a lot – machine learning and artificial intelligence being two of the biggest offenders.
Don’t get me wrong, there are many people who are incredibly skilled in these areas. I’m not saying they don’t exist, or are pointless, or should be ignored. We should all be working hard to avoid this type of jargon to be less intimidating, and easier to understand for Average Joe (or Josephine).
With that in mind, I have some tips that might help, no matter what your level of understanding. I may not be able to teach you how to ‘navigate the ever expanding and terrifying death trap of the data lake’, but everyone loves a bit of doggy paddle while they’re learning to swim, right?
1. Paddle with a purpose
Don’t set off on your analysis quest without an objective in mind. If you’re not looking for something in particular, the likelihood is you won’t find anything. Make sure you have some questions at the forefront of what you’re doing and keep bringing yourself back to these questions.
2. Clean up your act
No matter what sort of data you’re working with, how long it’s been around, or where you got it from, it could probably do with a good clean. The cleaning process is likely to change according to your different questions. Is your currency pounds, dollars, euros or something else? Do you need to convert these? How many Not Applicables (NA) do you have? Is it a valid response? In some cases, you may want to replace an NA with a zero. In others, that would skew your results and change your data.
3. Bitesized chunks
If you start by opening your dataset of however many million records, you might feel a bit overwhelmed. Start with something smaller and more manageable. There are several ways you can split up your data: by sampling or by objective.
By sampling – sometimes, it’s as simple as giving yourself a fraction of the original data to begin with. Make sure your data is randomly sorted and take a smaller selection of it. Use this to test your hypotheses and answer your original questions, and then go back to the bigger dataset to ensure these answers are the same when looking at everything in one go.
By objective – I’m a big believer in only taking what you need. You may not need all your data points to answer some of your questions. If you decide that your customer’s brother’s dog’s favourite day of the week isn’t relevant, it probably isn’t. Get rid of it. Focus on what you need to begin with, and you can always add in more data at a later stage.
4. Eyes wide open
You may have heard of EDA (or exploratory data analysis). This is always one of the most useful parts of the process for me. Get to know what’s going on in your dataset. Explore it, summarise it and visualise it. Get to know the context of your variables. What are your currency variables; is £10 normal, or is £100,000 closer to the average? What does this mean for you, for the data, for the questions you’re asking?
5. Mind the gap
You’ll often find yourself thinking ‘I wish I knew…’ There is a huge amount of data out there, in the public domain, for anyone to use. Have a look and consider whether any of this additional data could add another dimension. Looking at clothes sales? How much does weather have an impact on this? If you’re analysing customer engagement, would it help to know the demographics of the customers’ local area?
6. Question time
They say common sense is not so common, and in the context of data analysis, they may be right. It’s important to question all your findings, all the time. Does it make sense? Is it what I expected? Does it answer my original questions? Is it telling me something I can use? Is it useful for the business? Could I explain it to someone else? Would they believe me? Sometimes, talking someone through the insight can be beneficial – if you’re met with blank stares or confused faces, the chances are you may have missed something.
7. Beware the black box
Automation is everywhere now, but that doesn’t mean we should use it everywhere. It’s easy to find software and algorithms that can ‘analyse your data for you, at the click of a button’. Please approach these types of applications with caution. You know so much that they don’t. You know more about the data, the context, the analysis process so far. You know and understand your questions. You know what’s logical in the real world and what isn’t. Use these types of solutions to aid your analysis – but don’t blindly rely on it to do everything, no matter what the cheesy sales pitch says.
8. Document everything
It may seem like a pain when you begin, but I can guarantee that after you’ve accidentally misplaced your file, deleted your code, or killed off your laptop, you will be glad you did. Keep a note of all your steps, even if they seem small and insignificant. We’re not talking a publishable novel here; these notes are for your eyes only. Just jot everything down as you’re going – your variable transformations, how you decided to clean your currency, what you did with those NAs. It means if mistakes are made, it’s easy enough to go back to where you were.
And there you have it – an eight-step guide to paddling your way through the data lake; no need for an oar, or a snazzy automated-machine-learning-data-wizardry-speedboat-with-added-AI. Just you, your knowledge, your expertise, confidence, and some curiosity.
Bethan Blakeley is a director at Honeycomb
This article was first published in Impact.
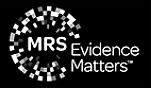
We hope you enjoyed this article.
Research Live is published by MRS.
The Market Research Society (MRS) exists to promote and protect the research sector, showcasing how research delivers impact for businesses and government.
Members of MRS enjoy many benefits including tailoured policy guidance, discounts on training and conferences, and access to member-only content.
For example, there's an archive of winning case studies from over a decade of MRS Awards.
Find out more about the benefits of joining MRS here.
2 Comments
Jenny Shep
6 years ago
Thanks for sharing - this makes a lot of sense. From a doggy paddler!
Like Reply Report
thesocializers
6 years ago
I agree about point number one, paddling with a purpose, in general. However, in our business of analyzing conversation data (from public social media posts), I do also encourage a some measure of "wandering", so as to find surprises in what consumers talk about.
Like Reply Report