Mind meets machine
In 2008, Chris Anderson, editor-in-chief of Wired, and the populariser of the ‘long tail’ model of marketing that captured the imagination of a million start-ups, turned his attention to what he called the ‘petabyte age’. This would represent, he wrote, “the end of theory”.
“It calls for an entirely different approach, one that requires us to lose the tether of data as something that can be visualised in its totality. It forces us to view data mathematically first and establish a context for it later… Google’s founding philosophy is that we don’t know why this page is better than that one: if the statistics of incoming links say it is, that’s good enough. No semantic or causal analysis is required,” he argued.
Anderson’s hypothesis was that models of the world, and therefore the people who create those models, would be redundant in the decision-making process if only we had enough data. It was a powerful argument in the early days of big data – politicians, policy-makers and marketers no longer needed to know why, because knowing was enough. “Forget taxonomy, ontology and psychology. Who knows why people do what they do? The point is they do it, and we can track and measure it with unprecedented fidelity. With enough data, the numbers speak for themselves.”
Only, they didn’t. In the decade since, market researchers discovered the limits of the petabyte age, sometimes by the costly misstep of throwing petabytes of data at a problem. Rather than ending theory, most attempts to remove the human from decision-making have pointed out exactly why we are still, in many cases, essential.
This isn’t the first time that inflated expectations of technology have been cut down to size. In 1933, the Chicago World’s Fair had confidently promised that “science finds, industry applies, man conforms”, an apparently optimistic message about work at the time that, with hindsight, seems not just misguided, but a bit creepy. In the century since, we have consistently assumed that machines are replacements for the power of our minds. There is evidence in market research, however, that the most effective uses of technology are coming from innovators who understand that minds and machines are more effective when regarded as complements, rather than substitutes.
Part of the reason for this complementary approach is that we are no longer using technology to retool factories, but to nuance decision-making. Paul Twite, managing director, MENA, at Toluna, has seen at first hand the evolution from no need for automation, to a sudden rush to automate, to an accommodation of the two. Toluna innovated in automated quant research: “We launched QuickSurveys in 2007,” Twite recalls. “And, to be honest, at that time no-one in the market cared because brand owners didn’t need information back that quickly. Market research agencies could spend three months making a report and putting a bow on it, because manufacturing cycles were nine months long.”
When the length of those cycles shrank dramatically, there was a sudden need for rapid responses, which Toluna could provide. “Automation removed a lot of time from processes. You didn’t need to brief a corporate agency to brief an agency in the field to collect data that was entered into a computer and analysed, and we were ahead of the market.” But automation had also outpaced good decisions in some cases, because it fragmented decision-making and led to poor-quality questions that would never generate high-quality insight, no matter how quickly it arrived.
For some of Toluna’s clients, multiple markets or business units would ask similar questions, but responses were not being shared, standardised or reflected on. Hypotheses were not systematically developed, evaluated or discarded. At its worst, the allure of the machine trapped organisations in tactics, rushing to the next decision, moving faster but often becoming less effective as decision-makers.
One of the ways in which Toluna is adding the human mind back into the process is by working with its clients to make its surveys more consistent and more impactful by using the advice of experienced researchers to analyse the whole of a client’s activity. Twite says: “We were on a call with a client this morning, with its insight team, and our methodologist had been looking at the types of questions it was asking on our platforms. He was able to offer tips about how you could explore that data in a more interesting way, to start revealing better insights.”
The promise of mind and machine working empathetically, Twite adds, will also be to make the best of rapid hypothesis testing: to examine the data, consider a question of interest, but then use automation to put that into the field immediately. In the next iteration of Toluna’s platform, clients can script questions and get response data back in real time – a process that, perversely, makes the human part of the research process more important.
The rush to automate
This swing from manual to fully automated, to a hybrid that recognises the shortcomings of each, has also taken place in qual. “There was a step change around the 2008 recession, when everyone had their budgets slashed,” recalls Jem Fawcus, group CEO at Firefish. “All our clients wanted to do more with less, and decided that technology was going to solve all their problems.”
The moment coincided with the apex of the big data hype cycle, and Firefish, like many agencies, found it was being pressured to replace minds with machines in its qual research. “At the time, it was presented as a binary, either/or thing. There were many technology providers with no background in research who were selling their services direct to the client. So we had to adapt pretty fast.”
The promise of automated ‘mass qual’ – what Fawcus has termed ‘Qual 2.0’ – was alluring, but the results were often superficial.
“There was a change in the definition of what was ‘good’… it used to mean the best quality you could get, the most actionable answer. Now, it often means what we can get in the time and for the budget that will help me make a decision. And sometimes it just means generating a bit of stuff, rather than a really robust argument.”
That ‘bit of stuff’ might mean a few video vox pops, for example, devoid of the context in which they were collected. Or huge amounts of information collected from online communities, but without any sense of how well those views represent the insights and opinions of community members.
Fawcus argues that many clients have learned from experience that they can get lots of information very cheaply, but not necessarily make better decisions from it – but this doesn’t mean rejecting all forms of automation. Nor does it mean that researchers should set themselves up as Luddites. One of their functions, he argues, is to use the baseline skills of moderation, or data analysis, or storytelling, to identify where technology can assist group moderation or a homemade video can make a telling point. The result is ‘Qual 3.0’, a synthesis of automation where it works best (for recruitment, for example), but shaped and directed by people.
“The researcher now needs to be adept at bringing together different information and data sources, filtering out the signal from the noise, putting it into a context of human understanding, and producing a strategy. At its best, it is a valuable skill that is not matched by technology. Nor is it matched by other disciplines,” Fawcus says.
Better together
At its most basic level, the combination of mind and machine can simply mean reallocating tasks where they are best done– a sort of basic optimisation of work. Alex Wheatley is director of digital and data technical innovation for Stan (Social Text AI and Natural language), an AI toolkit offered by the Kantar Analytics practice, which melds the abilities of minds and machines to derive meaning from social text, images, maps and all types of contextual data. “We take large amounts of conversation and try to find the themes and semantic correlations by running it through natural language algorithms. At the moment, it is mostly used to pull out and review trends. A client might be deciding where to invest, for example,” Wheatley explains.
While the automated part of Stan can spit out all sorts of correlations in the data, it can’t attach meaning, sense or causation to those ideas, and that’s where the minds take over. Some of the work involves catching errors that machines may make – for example, by conflating CBD, the cannabidiol, and a central business district, and finding an unusual trend as a result. But much of it involves triangulating results with qualitative, survey and panel data – what Wheatley calls a ‘curation’ process.
Counterintuitively, as AI improves, it has not lessened the reliance on human skill. Rather, just as the Industrial Revolution actually increased employment, converting skilled weavers into loom supervisors, AI is creating a richer set of ideas to curate. There are no plans to reduce the role of the minds in the process, Wheatley says. “As soon as we improve the output, there’s another finer level of granularity that needs our team again to make sense of it.”
With a company name of Digital Taxonomy, and a mission to use AI to code unstructured data, you would expect Tim Brandwood, CEO and co-founder, to enthusiastically minimise the role of the human in coding surveys. Not so.
“We’re purposely keeping people in the process and allowing them to do more,” he says.
Brandwood is a rare researcher-coder who also has experience at the sharp end of market research, having spent three years at Millward Brown. In 2015, Digital Taxonomy identified a lucrative “niche within a niche in market research”, as he explains it, coding the responses to open-ended questions in surveys. With repetition and consistency important, and manual processes that had hardly changed since the introduction of the desktop computer, this process was often still taking weeks for researcher teams to perform, or sometimes not being done at all.
But, having experience in AI, Digital Taxonomy has never advocated removing the human from the coding process. “There’s no point in paying a person to constantly categorise the phrase ‘good service’. Get a machine to code that,” Brandwood says. “The machine can do as much as it can, but then you need human coders to come in.”
The company has purposely downplayed the promise of technology, because Brandwood has witnessed fully automated coding applications that produce low-level insight from free text – a sentiment score, for example – but not the fresh information about the business that clients value. “What we can say is that the machine will double your speed by doing the 50% that it can do. But coding is nuanced, detailed and fine-grained – I want to free people from doing the grunt work, to spend the time doing the valuable work.” Ultimately, he says, the value might be from reversing the trend away from open-ended survey questions, which have often been abandoned precisely because they are not machine-readable.
The dog that doesn't bark
“Where AI and automation work well is in tasks that are repeatable, scalable and follow a common structure,” says Paul Hudson, CEO of FlexMR. “The desire of everyone to get the most from their budget is understandable, but it can lead us into oversimplification – the desire to hit an ‘analysis’ button.”
Hudson’s three rules for adopting machines are well-founded in technology, but don’t match well to what many of his clients want to discover – the insight that clients (and researchers) don’t yet know exists, or the good things that respondents or community members are not saying, to listen for the metaphorical dog that didn’t bark. Hudson has experimented with automating his qual research, notably by seeing how far he could push the use of moderator bots. But it has confirmed his insight that AI is rarely able to make sense of something on which it hasn’t been trained. Therefore, new ideas, or missing ideas, would be extremely hard to spot using machines alone.
His research on research has shown that, for large chunks of customer service analytics, where the AI may be looking for a tick up or a tick down in a predetermined set of KPIs, and marginal change in other indicators that may drive them, automation is a huge benefit. “That’s brilliant, it works really well. But apply this to any type of community data, such as a forum, and it doesn’t work well because there are open-ended questions and moderation. It has a group dynamic and doesn’t have a common structure. It isn’t repeatable, and the scale is smaller, so there is less to train the algorithm on.
“AI does not have wisdom, it has learning. We concluded very early that there is a benefit to having both a mind and a machine involved.”
Skim has taken this research-on-research method a step further, creating a project with its client Danone on how best to create insight. The experiment, which took place in 2017, led to a research paper called (Wo)man vs. Machine: From Competition to Collaboration. Its conclusion was that knowing when to think and when to automate has become a valuable skill for researchers.
“We were thinking about how we could speed up our qual research, make it cheaper, because many clients wanted to do qual with a limited time and budget,” recalls Marcel Slavenburg, the head of methods and innovation for Europe at Skim, “so we knew there was some place for automation.”
To find out where that place might be, Skim designed an experiment in which it would create three reports for Danone on its new product. The first would be entirely automated, the second would be done using traditional, human-only research, and the third would combine the two methods. The three teams would work in parallel, and the client would not know which methods had been used to generate the insights. The research output was generated by videos recorded using Voxpopme, and transcribed before analysis.
The result: a clear preference for the hybrid approach. “The human analysis took two weeks and was expensive, but had a lot of granularity,” Slavenburg explains. “Combining automation with human insight took half the time and we could do it for half the budget. But the machines did not connect the dots. We found it was better to hypothesise, and use that hypothesis to dig into the data.”
The advantage isn’t just in generating hypotheses or saving money, he argues; it enables more agile ways of working, for which clients and agencies need to collaborate. Early passes at the data can reset the research agenda, create new iterations.
And so, alongside the creativity and empathy of research, we have the third dimension that, in Carl Benedikt Frey’s research, protects jobs from being automated – negotiation.
Research, meet operational data
“We see that there is a lot of data that can be reused. There are outputs of machines that people just aren’t using,” says Nick Baker, CEO of Savanta. In the agency’s work with large clients such as Severn Trent Water, Savanta often discovers potential goldmines of insight that are outside the research framework.
One example is the digital experience analytics platform at Severn Trent that is employed to optimise the design of its online presence and analyse customer journeys in that context. Baker says: “We’re always trying to reapply data within the survey architecture, and have more decisions informed by more data, more often. We’re trying to get stuff in place so we can, theoretically, connect research to customer information, and when that ability is in place, you have something that’s like a heartbeat monitor for the organisation.” This not only provides real-time feedback on the company’s health, but can help direct the research agenda when more traditional methodologies are required.
To make the most of this opportunity though, researchers will have to build bridges with the human face of the machine. Rather than keeping the analytics function at arm’s length (“take this data away and show me something clever”, as Baker puts it), it can be used to adapt traditional market research methods. An example is the data analyst’s habit of iteratively adjusting the way the data is interrogated – asking different questions of it until it yields an insight – which is alien to a researcher’s instincts. “Having more people involved with different skills and experience helps us,” says Baker. “There aren’t many research agencies with tech capabilities in them. It’s either a massive miss or a massive potential for growth in the sector.”
The value of being human
Since the turn of the century, economists have been trying to quantify the decline in demand for routine work done by humans – what they refer to as the ‘hollowing out’ of the labour market. This term comes from the nature of change in employment – high-skill jobs cannot yet be automated, and low-skill jobs are done cheaply enough by humans to ensure that automation is not efficient; therefore, it is routine, mid-skill jobs that are being automated away.
Ironically, they have been able to measure this hollowing-out better through estimates provided by machine learning. The most recent contribution, by Nir Jaimovich, Henry Siu, Itay Saporta-Eksten and Yaniv Yedid-Levi, was published in February 2020 in a working paper titled The Macroeconomics of Automation. This focuses on the reduction in demand for routine work – such as, of course, coding surveys. It concludes that ‘routine-type’ individuals have experienced a fall of about 16% in the likelihood of working in routine occupations since the 1990s. Two-thirds of those workers have left the labour force, and the others have taken low-skilled jobs.
The definitive work on which jobs will remain human occupations was done in 2016 by Carl Benedikt Frey and Michael Osborne, at the University of Oxford. They used AI to rank 702 occupations in order of the probability that workers in them would be automated. For market researchers, the probability was well above average: 61%. The jobs in a sector that do not get automated, they concluded, are the ones that require creativity, empathy and negotiation skills, a pattern that is now playing out in the research industry.
Human-centred design
One of the most influential researchers and designers of the hi-tech revolution based his thinking on a profound belief that technology and our minds are complementary. Donald Norman, author of The Design of Everyday Things, and known as ‘the father of user experience’, has been vice-president of advanced technology at Apple, the founder of UX research pioneer the Nielsen Norman Group, and a professor at Harvard.
In 1997, he was already cautioning against tech fetishism that was making products harder for humans to use: “According to today’s machine-centred point of view, humans would rate all the negative characteristics (vague, disorganised, distractible, emotional, illogical), while computers would earn all the positive ones (precise, orderly, undistractible, unemotional, logical). A complementary approach, however, would assign humans all the positive traits (creative, compliant, attentive to change, resourceful) and computers all the negative ones (dumb, rigid, insensitive to change, unimaginative).”
At the same time, Norman gave an early warning that the fad for technology at work was placing too much focus on numbers, and too little on understanding the information that was being generated.
In reporting, he advocated “eliminating or minimising the need for people to provide precise numerical information, so they are free to do higher-level evaluation, to state intentions, to make midcourse corrections, and to reformulate the problem”.
Telling a story
“We’re all competing to get people to focus on the things that we think matter. Throwing more data at that problem is never going to convince people, so we need to be much smarter about the engagement process,” says Caroline Florence, founder of Insight Narrator.
One way in which the mind can definitely complement machines is that researchers can find a narrative in data that helps the business act on insight – a skill with which the data-driven side of the business often struggles.
In 2012, Florence set up Insight Narrator to coach ‘anyone who focuses on data’ to creatively communicate the insights that data is providing. It’s not just about making a better PowerPoint, she says: “It is the opportunity to inject a little bit of good thinking into the process without slowing it down.”
The common problem among her clients is that many of them are in fast-paced or agile environments, and have little time (or confidence) to convey context and narrative that leads to action. For example, Florence recently spent three days working with 60 data specialists from the government of Jersey. One of them was responsible for reporting costs in the health service. “She gets consistently asked by politicians and lobby groups how much a hospital bed in Jersey costs,” Florence says, “but she couldn’t answer because the models underneath are so complicated.”
The team eventually hit on the very human idea to mock up the price of the hospital bed as if it was an Airbnb room. In that way, the people who needed to know could intuitively understand the variation in price according to what was being delivered, without having to know the details of the data science behind those costs. It also made discussions about where to invest or how to cut costs possible, without misrepresenting or oversimplifying the data.
“Sometimes ‘big data’ is not the answer, it just creates more noise, and we need to be more sympathetic to the end audience – think about their expectations,” Florence says.
This article was first published in the April 2020 issue of Impact.
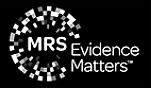
We hope you enjoyed this article.
Research Live is published by MRS.
The Market Research Society (MRS) exists to promote and protect the research sector, showcasing how research delivers impact for businesses and government.
Members of MRS enjoy many benefits including tailoured policy guidance, discounts on training and conferences, and access to member-only content.
For example, there's an archive of winning case studies from over a decade of MRS Awards.
Find out more about the benefits of joining MRS here.
0 Comments