Human intervention
Machine-learning algorithms are everywhere. They are used by taxi apps such as Uber to calculate when to impose surge pricing during peak times, and by Facebook to decide what content – and ads – to display.
They are also beginning to be used by some financial services companies, to inform decisions on loan lending, and by police forces to engage in predictive policing. Past crime data is used in predictive-policing algorithms to highlight people and areas that are most at risk of future crimes, to help police chiefs decide where best to allocate resources. However, recent studies have highlighted that this approach may reinforce bad policing habits.
A review by the Human Rights Data Analysis Group – a not-for-profit organisation that uses science to analyse potential violations of human rights around the world – showed that, as these algorithms are based on databases of crimes known to police, they cannot predict patterns of crime that are different from existing patterns.
This approach is flawed for a number of reasons. First, the predicted patterns are likely to be substantially different from the true patterns because only half of crimes are known to police. Second, it may exacerbate the over-policing of certain communities.
counterfactual fairness
To combat this, academics – led by Matt Kusner and Chris Russell, at the Alan Turing Institute in London – are developing a framework to identify and eliminate algorithmic bias. They propose a definition called ‘counterfactual fairness’, which is based around the theory that a decision is fair towards an individual if it gives the same predictions in the observed world and in a world where the individual had always belonged to a different demographic group (where other background causes of the outcome are equal).
Kusner, Russell and their colleagues map out variables in a data set and test how they might skew decision-making processes. If they find evidence of bias, they find a way to remove or compensate for them, rather than ignore these attributes.
For example, the team looked at data from the New York City Police Department on ‘stop and frisk’ events, in an attempt to model variables that would influence an officer’s decision to stop someone. First, they looked at all variables, including skin colour and appearance; then they considered only data related to criminality, such as whether they were carrying a weapon or had been arrested.
It was found that, in general, police considered black and Hispanic men as more criminal than white men, and stopped them more often. But evidence of criminality was found to be similar across the different groups.
“We propose that fairness should be regulated by explicitly modelling the causal structure of the world,” the report concludes.
Marie-Claude Gervais, research director at Dub, is a social psychologist by training, and has led consultation, research and evaluation programmes in a number of policy areas, including policing and community cohesion, equality and diversity. She says she is “delighted” that people are starting to understand the importance of putting algorithms and structural equation modelling in context.
“Numbers by themselves – although they look like magic little things that can solve every problem – are actually full of consequences, and they have social, ethical, political and financial implications for people,” she says. “As a mindset, I think that’s very powerful.”
But Gervais doesn’t believe this advance takes us that much closer to a solution. “They’re absolutely right in identifying the core problem, which is that – to work out algorithms that might be suitable – you have to understand two things. One is the nature of the service that you are delivering, and how discrimination has played out historically – and could play out now – in relation to that service and in different groups.”
Ethnicity and race are two categories of interest, Gervais says. Sexual orientation and gender identity – as well as age and disability – are others.
The other point, which Gervais says is well known and has been picked up by the study, is that there is a difference between individual discrimination and group-level discrimination.
“You could have somebody who is prejudiced – whether they’re conscious of their prejudices or not – or an organisation that has structurally discriminatory procedures in place, although the individuals within it could all be perfectly lovely.“
These two issues, says Gervais, mean the technical solutions for each service or target audience must be worked out on the back of serious consultation with experts around likely discrimination. What’s more, it’s vital to consider not just the data, but the interpretation of that data, as there is room for subconscious discrimination or prejudice there, too.
equality impact assessment
While the value of these approaches is high, the risk is too, Gervais says. “Because public services, by definition, affect everybody, there’s a real risk that the impact would be huge if they get it wrong.”
In some situations, the number of factors that must be considered to make a fair, thought-through decision means a more traditional decision-making approach could be more suitable.
Gervais describes a consultation project that she conducted with the Greater London Authority, which was an equality impact assessment on the London housing strategy for 10 years.
“The project involved consulting with all categories – that included looking into ethnicity, gender, disability, asylum seekers, people with refugee status, gypsies and travellers. They all had completely different issues in relation to housing – so if you wanted to create an algorithm for housing allocation, it would be incredibly difficult.
“There used to be a kind of algorithm, but the local authority moved away from that system and now it uses an approach called choice-based lettings; it puts all available properties in the area on its website, and leaves individuals to pick the one that suits their needs best.
“It realised that trying to work it out on people’s behalf was creating all sorts of distortions, and spatial and employment segregation. So the authority shifted away from trying to process it itself to giving choice to residents – and it worked.
“Leaving it in the capable hands of the people who live with the consequences of their decision is a good idea.”
Counterfactual Fairness, by Matt Kusner, Joshua Loftus, Chris Russell, Ricardo Silva (Preprint) arxiv.org/abs/1703.06856
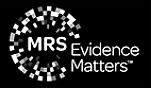
We hope you enjoyed this article.
Research Live is published by MRS.
The Market Research Society (MRS) exists to promote and protect the research sector, showcasing how research delivers impact for businesses and government.
Members of MRS enjoy many benefits including tailoured policy guidance, discounts on training and conferences, and access to member-only content.
For example, there's an archive of winning case studies from over a decade of MRS Awards.
Find out more about the benefits of joining MRS here.
0 Comments