A problem shared: Collaborating in research
I often think one of the hardest things about being an adult is answering the question, “So, what do you do?”. Sometimes, I work in market research. Other times, it’s analytics or data science, or research analytics. I consider myself lucky to be ‘cross-discipline’; it keeps my life interesting.
Another thing that keeps my life interesting are the tools I use across these disciplines. For the data science part of my role, I mainly use open-source computer programs. Open source is a bit like Wikipedia – written by the general public, accessible to everyone, and anyone can edit the pages if they want to.
Unlike Wikipedia, however, this doesn’t call the programs’ reputation into question – you won’t find university lecturers slapping students’ wrists if they use open-source programs such as R or Python, in the same way you would if they listed Wikipedia as a reference. In this case, it means there is a wealth of advice on the internet written by those who use and edit the code behind these programs. You’ll often find me copying and pasting error messages into Google, or asking “how do I… in R?” – and I know I’m not the only one. Even Hadley Wickham, a huge name in the industry, who is responsible for overhauling the building blocks of the coding language used in R, has said he’s the same.
Websites such as Stack Overflow are full of helpful troubleshooting tips, Q&As and code from other people you can copy and paste into your own work. The data science world is filled with people who want to help you, and the community is set up to enable that type of hive-mind problem-solving.
This got me thinking – what makes the data science industry different from the others I work across? I might be wrong – and please let me know if you disagree – but the market research industry just doesn’t seem as forthcoming in sharing the ‘nuts and bolts’ of the techniques and methodologies we use. I know, from helping organise various events in the research analytics world, that there is often a nervousness about giving away ‘state secrets’ to the competition. So, how do data scientists overcome this?
I asked the supportive network of those in that space for their opinions. A few key points were raised to explain how data scientists excel at helping each other, while also staying competitive and true to their companies and clients, and I think the market research industry could benefit from similar thinking.
If there is detail on the internet about how to run a certain model, conduct a type of analysis, or use a specific methodology, it’s important to recognise that’s all it is. Most often, it isn’t the methodology your client is paying for – it’s you. You are the one who knows the client, their objectives and their needs; you understand the nuances in the market and in the data; you get how their consumers feel, and what the board wants to see. Using someone else’s code or research design can’t help with that critical contextual expertise. To quote Matt Squire, chief technology officer at Fuzzy Labs: “The general approach is open source; the application is secret sauce.”
Innovation breeds innovation. If we share our ideas, our techniques, our innovation, we will collectively innovate better, faster, and more efficiently. It’s that hive-mind approach, the give and take – I’ll give you this idea and take that one – that enables us to improve on what we have. If you are not part of this sharing and innovating cycle, you risk being left behind.
Most clients are, understandably, a bit nervy about paying what can be thousands of pounds for something unproven, untested, brand new and out of the box. So, sharing, testing, improving and making these techniques fail-safe before spending clients’ money on them is another win-win situation. You can iron out kinks in the details before it’s too late. New techniques are only helpful and meaningful if we can use them in a real-world setting. A new whizzy methodology that nobody trusts is worthless.
I’m not saying the market research industry doesn’t do any of these things. Conferences are held all over the world where people share details of the projects they are running. There are small pockets of individuals keen to share thoughts and ideas to keep that innovation turning – the Advanced Data Analytics Network from the Market Research Society, the Association for Survey Computing, and the Sawtooth community are just a few.
What I am saying is that I believe we could get better at this. After all, why wouldn’t we want to improve our work and our networks, and learn a thing or two along the way?
This article is from the July 2022 edition of Impact magazine.
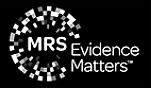
We hope you enjoyed this article.
Research Live is published by MRS.
The Market Research Society (MRS) exists to promote and protect the research sector, showcasing how research delivers impact for businesses and government.
Members of MRS enjoy many benefits including tailoured policy guidance, discounts on training and conferences, and access to member-only content.
For example, there's an archive of winning case studies from over a decade of MRS Awards.
Find out more about the benefits of joining MRS here.
1 Comment
Dr. Stephen Needel
3 years ago
The issue, Bethan, is that marketing research techniques are often proprietary - most data analysis is not. I'm happy to share how I analyze my data, but I'm not about to share how we build our virtual reality simulations - that's what we sell. It's the same with all the AI claims these days - nobody will actually tell you how the machine learned or the model created by the AI mechanism, they simply tell you what they put in and leave the rest to the imagination. You also assume most techniques have been validated - most have not. It's why most proprietary methodologies die off in a couple of years. Look at what was presented at IIeX a few years ago and see how few are operating today. Sharing is great, but the current model of a marketing research business will keep that from happening to any meaningful extent.
Like Reply Report