Without truth, there is no future for AI
As humans we rightly question and challenge presumed facts. Given the volume of commentary on instances of bias or misunderstanding in algorithms that are being used, it stands to reason that although artificial intelligence (AI) is being introduced to provide quicker answers or solutions, we are likely to challenge the information presented more rather than less. For example, a 2022 IBM survey found that 84% of executives said that “being able to explain how their AI arrives at different decisions is important to their business”. Put simply, we need to create a root of trust in how we use AI.
That’s not to say we should be inherently mistrusting. For insights managers, generative AI-based tools are a game changer. This technology promises drastic cuts in the time it usually takes them to dig into the details of enormous amounts of information in order to find insightful answers. But a quick answer isn’t always the right one, and that’s a barrier to adoption.
Trusted data sources
The information that some large language models (LLMs) provide isn’t always correct, a tendency otherwise known as hallucination. These out-of-the-box LLMs neither have access to current data, as they have only been trained on data up to 2021, nor a company’s proprietary data. Instead, they have also been trained specifically to make up the best and most plausible response to the prompt (in this case the question) they are given. Without knowledge or critique, it is not always possible to tell whether the response is legitimate or not, irrespective of how professional or convincing it sounds.
Research and insights teams need to ensure the sources being used by their chosen AI tools to create an answer contain the right data and are trained to prevent them from making up an answer whenever they can’t identify relevant knowledge. To achieve this requires the AI to be trained to understand the full context of questions before exploring knowledge pools. Before serving an answer, they need to further check if the content fulfills the intent of the question, and most importantly of all that the information provided contains links to citations from verified and trusted sources.
Technical capabilities will need to develop quickly in order to not to run aground when faced with research projects and reports that contain more than words alone. Most reports contain valuable information in terms of data charts and tables that are a treasure trove from which to generate new insights. Consider, for example, that many presentations use creative visual styles to convey information through methods like colour coding, visual hierarchies and arrows. AI tools will need to develop new skills to faithfully interpret and disseminate the full richness of information that is contained.
Learning to work alongside AI
While building trust takes time, losing trust often happens quickly. You’ll need a clear application strategy for AI adoption to ensure it becomes widely accepted. Businesses should begin by identifying small areas of low-hanging fruit, applying AI where there is limited risk to their operations but a lot of upside and efficiency, like knowledge management. Once comfortable, moving higher up in the value chain to areas that may have more risk if something goes wrong, such as customer or market facing activity, will be easier.
Successful AI adoption is as much about teaching people about the limitations and behaviours of AI as it is about the technology capabilities itself. It’s vital to start your AI programme somewhere rather than putting it off, because a large part of the recipe for success is developing new skills in your people. For us to entrust AI to handle complex tasks and jobs, we need to become familiar with the details and nuances of how different AI tools work, respond and evolve.
Orchestration of trust
Future technology stacks supporting insights will include a multiplicity of new tools interfacing directly with one another. Imagine an orchestra of AI agents, each playing a clearly defined and optimised role to achieve a joint task or outcome.
You might have one agent to generate hypotheses for new activation campaigns, delegating to an analyst that picks the hypotheses apart and uses an army of fact-finders and fact-checkers to gather all available information to assess and improve the hypotheses. This might then be passed onto another AI when a certain action triggers the next step. A creative generating agent, for instance, might then employ automated online research to assess and iterate creative approaches, even running a/b tests on digital channels. All this could be executed in line with corporate best practices, brand guidelines and policies.
Establishing an AI to take on this orchestration role will be critical for insights operations, but this will also demand that insights managers themselves develop their digital skills to steer their AI operations firmly. When we establish this trusted orchestrator, our AI insights ecosystem will be able to support creative strategies and options that are grounded in truth and not fantasy.
Olaf Lenzmann is chief innovation and product officer at Market Logic Software
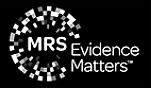
We hope you enjoyed this article.
Research Live is published by MRS.
The Market Research Society (MRS) exists to promote and protect the research sector, showcasing how research delivers impact for businesses and government.
Members of MRS enjoy many benefits including tailoured policy guidance, discounts on training and conferences, and access to member-only content.
For example, there's an archive of winning case studies from over a decade of MRS Awards.
Find out more about the benefits of joining MRS here.
0 Comments