Why now is the golden age of marketing measurement
Measuring the effectiveness of marketing spend and the best allocation of media investment regularly ranks as one of marketers’ top objectives – not least when budgets are under ever more scrutiny. Marketing mix modelling (MMM) has become the go-to method of measuring the individual, and combined, impact of multiple marketing channels on business objectives – and intriguing developments in MMM are making it more accessible and powerful than ever before.
For much of the past 20 years industry attention has been focused on user-level digital attribution – driven by the emerging dominance of digital advertising and the comfort and interpretability that this deterministic form of measurement offers. There were limitations, namely that it only works with online channels, allows for duplicate conversions across digital channels and has a propensity to over-attribute conversions to digital channels, especially those lower down the purchase funnel like retargeting display and paid search.
But the positives were seen to outweigh the negatives, leading to a near hegemony for digital attribution in the 2010s. But in recent years it has been undermined by the deterioration of the data signals that it relies upon. The introduction of Apple’s app tracking transparency (ATT) in 2021, alongside other initiatives aimed at giving people more control over their personal data, have resulted in digital campaigns achieving fewer conversions, and marketers increasingly becoming uncertain about the effectiveness of digital channels.
This uncertainty has led to a renaissance in alternative measurements – in particular, econometrics (see Figure 1 ). Renewed interest in MMM has also been aided by exciting recent developments, which have significantly lowered the barrier to entry. Today, anyone – or team – with a decent understanding of the R or python programming languages and a good grasp of marketing effectiveness theory can run an end-to-end MMM project.
Figure 1: Google Search Trends
The recent release of several open-source (free to use) libraries, including those developed by tech giants like Meta, Google and Uber, has revolutionised MMM. The algorithms underpinning these solutions sit at the cutting edge of data science and machine learning, helping deliver reliable models with a high predictive accuracy.
MMM isn’t perfect; detractors label it more of an art than a science because the process requires human involvement. MMMs often produce multiple candidate models, and analysts must evaluate the models through the lens of past experience, to select the ‘best’ model for a given project. But the same criticisms can be levelled at all modelling, from government use to plan pandemic responses to those employed in the finance industry. This blend of science and art is invaluable in the explanation of complex systems underpinning critical decision making.
In comparison, digital attribution is less open to interpretation and more directional – once settings are selected (for example, attribution window) the metrics are simply provided and often treated thereafter as fact. Both digital attribution and MMM can suffer from a lack of causality. MMM’s can uncover relationships between marketing deployment and incremental uplifts in sales, but they don’t automatically prove a cause-and-effect relationship between media spend and sales. Compared to digital attribution, causality tends to be more explicitly stated within MMM, and the evaluation of the models via experiments can do a good job of resolving this dilemma.
The holistic approach of MMMs generally make them more insightful, actionable and impactful than digital attribution, especially for advertisers investing in offline and online channels. It remains the only marketing measurement tool that allows advertisers to compare all channels like-for-like on business-critical metrics like return on investment.
MMM is now evolving into unified marketing measurement (UMM). The biggest providers’ proponents of MMM, including Kantar and Google, are introducing UMM in 2023. Rather than referring to one specific framework, each firm has its own definition of UMM, the one unifier is that MMM lies at the heart of any UMM project. It’s the synthesis of MMM with a combination of other measurement solutions like digital attribution, experimental results and brand equity studies that makes UMM unified. The glue that holds these disparate measurement solutions together is typically Bayesian inference – a statistical model itself. In effect, UMM is an ‘ensemble’ approach – a collection of sub-models interacting within a grand model.
While UMM’s development is fascinating, it’s an incredibly nascent technology. There is no one best approach, and UMM projects are often expensive to deliver. Any advertiser looking to improve campaign impact measurement should begin with MMM and build from there. And with more paths to MMM than ever before, it’s a fantastic time to start.
Jamie Parks-Taylor is director of insight and analytics at Cream
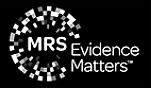
We hope you enjoyed this article.
Research Live is published by MRS.
The Market Research Society (MRS) exists to promote and protect the research sector, showcasing how research delivers impact for businesses and government.
Members of MRS enjoy many benefits including tailoured policy guidance, discounts on training and conferences, and access to member-only content.
For example, there's an archive of winning case studies from over a decade of MRS Awards.
Find out more about the benefits of joining MRS here.
0 Comments