Why it’s time to move beyond sentiment analysis
One of the first, rudimentary attempts to analyse text data at scale resulted in producing so-called ‘word clouds’, which simply surface words which appear most frequently in a body of text. Similarly, sentiment analysis emerged as one way to get a number, or a sliding scale, out of text – after all, the analytics world has become very wedded to quantitative ways of thinking. This, at least, has the advantage of being relatively easy to communicate to stakeholders, and while still not perfect, has come on leaps and bounds since the first attempts.
But the field of natural language processing (NLP) has gone through a number of paradigm shifts in recent years and we can aim far higher than this. The desire to get a quantitative measure from qualitative data has long been there, but only recently has it become actionable.
At its core, NLP is the process of taking unstructured data and making it possible to analyse. While ‘natural’ might imply human intervention, it centres around the computerised analysis of language as it is spoken and written naturally. This, in turn, can enable granular insights. If you run a delivery service, for instance, you’ll want to know if your customers are happy with it; but this is a multifaceted question, not easily answered by a sliding scale or a quant figure alone. Might the communication be a potential stumbling block? The packaging? How do the priorities of particular groups vary?
AI in the public consciousness
Today, artificial intelligence (AI) – of which NLP forms a part – is in the public consciousness more than ever, thanks in no small part to OpenAI’s ChatGPT. Yet, this attempt to make conversing with an AI feel smooth and natural, and to provide information and answers through a conversational interface, despite being impressive, has its limitations. It lacks critical thinking skills and can present misinformation.
Similarly, when it comes to NLP, the largest problem right now is a lack of real world outside knowledge embedded within the technology. For instance, to put a recent spike in people talking about non alcoholic beer in context, you’d ideally need to know about Qatar’s abrupt reversal of its beer policy for the World Cup, when it decided only to sell non-alcoholic options at its stadiums.
Clearly, we are a long way off from AI taking over analysts’ jobs. While there is always hype around new technologies, just because a technology is cool, doesn’t mean it needs to be used for every task. Sometimes people get excited and want to make complex models with AI, just because they can – but in general, in order to derive tangible benefit, it can be wise to start small and to keep a specific goal in mind.
That said, moving beyond frequency when it comes to the analysis of language is particularly important in a cost-of-living crisis and at a time when consumer behaviour continues to shift at pace. By way of example, if your brand is very similar to others in the market, you need to uncover nuance, to discover what’s unique about what you offer. This sort of robust competitive analysis requires sophisticated NLP – something which can help hugely when it comes to effective brand positioning.
The growing significance of first-party data
Businesses which stand to benefit the most from new technologies are those which understand the power of data and attempt to organise and structure it. Those with large amounts of first party data, in particular, tend to be ahead of the pack.
What’s more, in this increasingly complex world, combining NLP with other research methods is hugely beneficial. Right now, insights professionals don’t do this nearly enough. As an industry, we need to look at data in a more layered, nuanced way. Are you considering NPS score? Reviews? Frequency of interaction? Take surveys: these offer qualitative understanding, thanks to open ends, as well as quantitative data. Using various data sets in combination enables far deeper discovery.
The last few years has seen a huge increase in sophistication in the analytics industry. To continue this progress, it’s important to keep front-of-mind what it is we want to achieve. No single tool does everything. Think, is your research goal to confirm, to discover, or simply to keep an eye and monitor?
Technologies such as AI and NLP are not magic. Ultimately they exist to be useful. It follows that our objectives should determine our approach. We are more likely to achieve this if we blend and layer data sources and if we move beyond the basics; using automation to uncover the ‘why’ behind the ‘what’.
Ryan Callihan is head of AI at Relative Insight
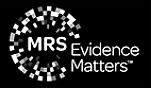
We hope you enjoyed this article.
Research Live is published by MRS.
The Market Research Society (MRS) exists to promote and protect the research sector, showcasing how research delivers impact for businesses and government.
Members of MRS enjoy many benefits including tailoured policy guidance, discounts on training and conferences, and access to member-only content.
For example, there's an archive of winning case studies from over a decade of MRS Awards.
Find out more about the benefits of joining MRS here.
0 Comments