What fantasy football can teach us about predictive modelling
Fantasy football isn’t just a fun game. Steadily over the last decade football modellers have become increasingly good at predicting the performance of footballers to an almost uncannily accurate degree.
From developing a new, more accurate metric for predicting future scoring potential to pioneering the use of synthetic data years ahead of any market researcher, fantasy football pros have honed their skills to now use advanced AI modelling techniques that could put market researchers to shame.
Examining the evolution of the data analytics techniques being used in fantasy football can offer valuable lessons for market researchers navigating the evolving research landscape.
From football pitch to supermarket shelf
Predicting the performance of a football player on the pitch and a brand on the supermarket shelf presents surprising similarities. Both involve analysing a complex web of interconnected factors that are constantly in flux.
For football players, this means not only tracking how good the player is, but also how good their team is, which team and players are they are playing against, the formation and tactics of the team, and even the mood and form of the player and whims of their managers.
Likewise for brands, it is not just about monitoring product appeal. Distribution networks, competitor activities, promotional strategies, marketing campaigns, macroeconomic trends, evolving consumer needs and even seasonal influences all play a role in predicting performance.
However, unlike the readily available and meticulously analysed performance data in fantasy football, gathering consistent and reliable brand data to build robust predictive models can be a significant hurdle for market researchers.
Four game-changing lessons from fantasy football data modelling
There are four key takeaways from the evolution of fantasy football analytics that market researchers can utilise in their own data analysis. First, is that performance data alone tells an incomplete story.
Relying solely on past performance statistics is a recipe for inaccurate predictions in football and brand analysis. Simply adding up how many goals a player has scored is no way to reliably predict their future performance – a star player on a struggling team might appear mediocre, while an average player on a dominant team could rack up impressive figures.
Similarly, a brand’s future performance cannot be predicted by sales alone which can be heavily influenced by external factors like distribution, competitor promotions or economic conditions.
Fantasy football analysts recognised this early on and started to develop more sophisticated prediction metrics like expected goals (xG) that consider shot quality and location, not just raw goal tallies, to provide a more accurate predictor of future scoring potential. They further refined their models by incorporating other calibration metrics such as fixture difficulty ratings, players predicted playing time, and a vast array of marginal factors like the time of day a match is played and period between games.
The lesson for market researchers is clear: contextual data is crucial. Market researchers can be overly fixated with the brand’s tracking metrics. However, brand tracking alone is not enough to build robust predictive models. Market researchers must collate wider contextual data on distribution, competitor activities, marketing spend, and other relevant external factors that will impact performance and build these into their predictive models.
Choosing the right metrics: Focus on clarity and impact
The quest for the perfect performance predictor led fantasy football analysts to experiment with countless metrics, of which there is a sea of different types of football performance data to choose from. They meticulously evaluated each one, ultimately settling on xG as the most reliable indicator. This process highlights the importance of selecting clear, impactful metrics that genuinely contribute to the model’s predictive power.
There is a temptation when developing market research models to load up as many data points as you can, but adding metrics that overlap or correlate strongly with existing ones introduces noise and can reduce not enhance accuracy. Researchers could learn the importance of rigorously evaluating potential metrics and prioritising those real causal factors that provide unique insights and enhance the model’s ability to discern meaningful patterns.
The power of synthetic data
There is a lot of talk in the research world about synthetic data. Well, fantasy football analysts have been utilising synthetic data generated through multi-simulations to enhance data models for several years. By introducing controlled randomness that mimics real-world variability through a process known as ‘Kalman filtering’, fantasy football pros can test their models under various scenarios and refine their predictions. In fact, the best ones are now working almost entirely from hundreds of synthetically generated data simulations to help them deal with the chaos and randomness of real-life footballer performance data.
There are some really interesting opportunities for market researchers to adopt some of these techniques by creating synthetic datasets based on their existing data. This is especially useful when gathering human survey responses. Each batch of responses are likely to be quite erratic as different people are surveyed each time, therefore producing a sample error. However, with enough data, market researchers can start to model the answers in the same way as football data and create a synthetic copy of slices of the market data. This would allow for more rigorous testing and validation of models, leading to more accurate and reliable predictions.
Embracing machine learning
The most successful fantasy football analysts have embraced machine learning algorithms to process vast datasets and identify complex patterns that human perception alone would not be able to see. This AI-driven approach has been instrumental in achieving remarkable predictive accuracy and boosting a competitive edge in the game in recent years. Humans all too often succumb to seeing phantom patterns in data.
By taking advantage of these machine learning techniques and using the right blend of data, there are opportunities for researchers to better analyse complex datasets, uncover hidden relationships, and develop the same powerful predictive models that have taken over Fantasy Football and won.
While brand sales don’t always play out quite like a football championship tournament, with market share increases stacking up much slower than goals scored, the success of fantasy football predictive analytics underscores universal data modelling best practices that can be applied to a wide variety of applications within market research.
Gathering consistent and reliable secondary data to consolidate into impactful metrics remains the foundation of strong predictive models. This means that even without the immediate feedback loop that weekly football results offer fantasy football players, market researchers can utilise comprehensive data collection, meticulous metric selection, and the strategic application of machine learning techniques and synthetic data to unlock new levels of predictive accuracy and deliver more impactful insights.
Jon Puleston is vice-president of innovation, Profiles division at Kantar, and has held the global number one spot in the Fantasy Premier League at various points over the past three years
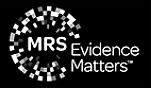
We hope you enjoyed this article.
Research Live is published by MRS.
The Market Research Society (MRS) exists to promote and protect the research sector, showcasing how research delivers impact for businesses and government.
Members of MRS enjoy many benefits including tailoured policy guidance, discounts on training and conferences, and access to member-only content.
For example, there's an archive of winning case studies from over a decade of MRS Awards.
Find out more about the benefits of joining MRS here.
0 Comments