Text analytics needs critical thinking
Organisations are awash with data, but many are not deriving maximum value from it. Too often, they overlook data streams which can answer the critical question ‘why?’ as opposed to the – often more straightforward – question of ‘what’.
This is partly because of the limitations of conventional approaches to text analytics. The capabilities commonly embedded in social listening and survey analysis platforms, for instance, typically rely on frequency-based analysis. Essentially, they count the most common words and phrases.
Herein lie two significant issues. First, the most common words and phrases aren’t always the most insightful. Second, context is necessary to judge the importance of a finding.
Part of the problem is that when people think of ‘analytics’, they tend to think ‘numbers’; so, quantitative research. In contrast, qualitative research is often considered almost ‘anecdotal’ – good for adding colour to the numbers, rather than particularly valuable in and of itself.
And yet, while the numbers can tell us a lot, the vast majority of data businesses have at their fingertips is unstructured. It comes in the form of text, video and images; meaning that many analyse only a fraction of what’s available.
Insights teams tend to shy away from this potential goldmine because words are more difficult to analyse in a scalable, efficient way than numbers, which are black or white. Take the word ‘spring’. This can refer to a mechanical component, a season, a body of water or a verb, depending on the context. It’s far easier, of course, to enter a formula into a spreadsheet.
Alongside this, many choose to monitor social media manually or to rely on rudimentary word clouds. The former being very labour intensive and the latter tending to surface the most commonly occurring and often topic-specific vocabulary: words such as ‘shopping’ in a retail context. This won’t shed light on your unique business problems.
Yet taking this approach does at least give the sense that something is being done. We need to ask ourselves, however, how much critical thinking is happening here. From media monitoring to social monitoring, my suspicion is not very much – and often only from a reporting lens.
What’s more, as with any research, buy-in is needed. Qualitative analysis is often considered more ‘finger in the wind’, or ‘gut feel’. Lack of context is another issue with standard approaches. One-dimensional text analysis, which often relies on frequency, tends not to offer any comparison.
For example, if you know that 20 customers have been complaining about battery life, it would be helpful to compare that to the competition, or to last month, to work out the true significance of this. A single data set in isolation tends to tell very little. Introducing an additional reference point can turn information into something actionable – making insights more robust, more defensible, and better able to hold up under scrutiny from senior management.
What can be done?
First, we must start with data collection and the old adage: good data in, good data out. Ensure open-ended survey questions are carefully, and very intentionally, written. Very often the last question of a survey is simply, “Is there anything else you want to tell us?” Character minimums for answers can help, given that there is a need for both quantity and quality. It’s critical to design surveys which are likely to elicit rich, full bodied responses. Similarly, with social media analysis, think about what you’re trying to learn and why – and do this from the outset and not while a survey or a campaign is running.
It’s also important to understand stakeholder concerns. A director of product is likely to seek very different information to a director of marketing. A clear understanding of objectives must form a guiding light to the approach. Of course, if business leaders gravitate towards the numbers, text analysis may be regarded as a bolt-on. Teams need those who understand the benefits of qualitative insights and who can work to promote their value and to ensure a considered approach is taken.
While technologies such as artificial intelligence (AI), and natural language processing (NLP) are evolving and becoming more accessible all the time, insights teams must distinguish between the hype and the reality of new tools. It’s right to be both excited and wary of AI. While it can offer welcome objectivity and efficiency, removing humans from insights-gathering can be risky.
Deploying AI in the portion of the process that’s most laborious makes the most sense. There remains a hugely important role for analysts to sift through and identify what’s most important and relevant to their stakeholders, with AI being used as a means to move humans away from low-value tasks.
After all, even with the most advanced tools, qualitative analysis is rarely black and white. Understanding and narrative building is required. So be wary of those promising to automate the whole process. Will this yield high quality results? How well can we train AI to understand the needs of stakeholders?
Let’s not forget, either, that senior stakeholders can be prescriptive, and that they don’t know what they don’t know. Be intentional with the design of insights gathering. Ensure critical thinking and a consideration of context, and leverage text analysis in a way which generates insights which help qualitative research shed any out-dated, legacy associations of ‘finger in the wind’ analysis.
David Koke is senior performance marketing manager at Relative Insight.
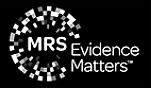
We hope you enjoyed this article.
Research Live is published by MRS.
The Market Research Society (MRS) exists to promote and protect the research sector, showcasing how research delivers impact for businesses and government.
Members of MRS enjoy many benefits including tailoured policy guidance, discounts on training and conferences, and access to member-only content.
For example, there's an archive of winning case studies from over a decade of MRS Awards.
Find out more about the benefits of joining MRS here.
0 Comments