Protecting the science
So, rather than just throw stones, here are my ten key points in ensuring that we protect the scientific method that underpins quantitative market research.
( 1 ) The assumptions behind a new method need to be stated
Every method requires some assumptions to be accepted. Providers of research methods should spell out what users are expected to believe.
For example, in social media monitoring the user is often required to believe that those who post are broadly typical of those who do not. With predictive markets the user usually does not have to accept the sample is representative of the population, just that the method has been sufficiently validated (for example, by establishing and testing a theory or by providing relevant benchmarks).
( 2 ) Estimates and predictions need probability ranges
When we make estimates or predictions the user should be given a sense of their accuracy and reliability. This information should say whether this is based on established theory or empirical data. For example, with a random probability sample of 1,000 people, theory suggests the sampling error is +/-3% at the 95% confidence level. By contrast, an election poll prediction might assert, “In the last 20 elections our average error was 1.5% and the largest error was 4%”.
( 3 ) The link between a sample and the target population needs to be explained
A random probability sample represents the population it was drawn from, but these are rare in market research. If somebody has a sample, for example of Twitter users or viewers of a specific channel, and they wish to use this sample as a proxy for the wider population, they should specify why it works, the extent to which it works as a proxy, and when it does not work.
( 4 ) A large sample is still only a sample
The large sample myth should have died in 1936, when the Literary Digest had a sample of over 2 million people, and still produced the wrong forecast of the US Presidential election (and George Gallup got it right with a small, but representative sample).
A large unbiased sample is usually better than a small unbiased sample, but a small unbiased sample will often give a better prediction than a large biased sample. Large samples are great because they allow more sub-groups to be analysed, but understanding the sources of bias is key to using any sample, small or large.
( 5 ) People are usually unaware of their own decision hierarchy
Questions like, “When you buy breakfast cereal, what is the most important feature?” or, “If I were to offer you a coffee at twice the normal price, would you still buy it?” tend to lead to inaccurate responses, because people are poor witnesses to their motivations.
Although researchers have known this for 50 years, we still see it being abused. Every week there are reports saying things like, “80% agree that ‘Visiting this Facebook page makes me more likely to buy the product’” or, “75% of respondents agree that ‘The effective use of Big Data is the most important challenge to my business’”.
If and when there is a link between what people say in response to a survey question and their behaviour it is usually a link that requires modelling. For example, the link between purchase intent and market performance is usually based on re-weighting the responses and adding in factors for distribution, advertising, and promotional activity.
( 6 ) Correlation is not causation
Yes, we all know this, and we repeat the words on a regular basis, but we don’t always take it on board. Research magazines, websites, and conference presentations are full of claims that pinning on Pinterest leads to purchase, that Facebook usage leads to sadness, and tweeting leads to action. In most cases this is simply correlation, not causation. Just because A followed B does not mean B caused A.
For example, before I go out, I put on my shoes. Putting on my shoes precedes going out, but it does not cause me to go out.
( 7 ) Big Data still needs causation
Chris Anderson, editor of Wired Magazine, has written that in the age of Big Data we won’t need the scientific method and won’t need to need to worry about the difference between causality and correlation. This is simply wrong! Big Data will produce many more spurious associations than real ones. Because the data is so big, there are an almost endless number of ways that data could interact, purely by chance, making the development and testing of hypotheses essential.
( 8 ) Reverse inference introduces subjectivity
Reverse inference is the process of mapping an observable phenomenon to a stimulus, typically requiring a subjective, qualitative element. For example, if somebody is shown a TV commercial and at a specific point in the ad we see from the fMRI scanner (or from biometrics, or from facial coding) that they respond strongly and negatively, then we need to link the ad to the response. The process of linking the data to what is shown in the ad is reverse inference, and typically requires human judgement – not science.
( 9 ) Successes are sometimes just survivor bias
Survivor bias is typically caused by looking at successful cases and asking, “What do they have in common?” The assumption is that the things they have in common are the reason for their success. However, unless the cases that failed are reviewed, the characteristics of the successes are almost useless.
For example, assume we look at 100 of the best TV ads for the last few years and we notice that 80% have music. With 80% having music, we might determine that music is a key feature of success. However if we inspect all the ads aired over the last five years we might find that music appeared in 90% of these. In this case the initial assumption changes, since only 9% of ads with music were successful and 20% of ads without music were successful.
( 10 ) To know if something works you need to try to break it
Any new approach should first be tested in favourable conditions to see if it works. If it works under favourable conditions, it should then be tested in less favourable conditions to find out when it fails.
It often seems that market researchers are too quick to publish results based on favourable cases. Side-by-side comparisons between Twitter and surveys, CATI and online, and online and mobile often seem to reflect favourable cases. For example, by using scales that relate to deeply held beliefs, rather than brand association scales.
New is good
Market research should be trying new things, but it needs to ensure that what it is selling is based on sound methodology, and these ten points will help protect market research from the encroachment of pseudoscience.
Ray Poynter is director of Vision Critical University and author of The Handbook of Online and Social Media Research. He also founded NewMR.org.
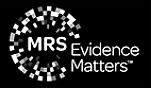
We hope you enjoyed this article.
Research Live is published by MRS.
The Market Research Society (MRS) exists to promote and protect the research sector, showcasing how research delivers impact for businesses and government.
Members of MRS enjoy many benefits including tailoured policy guidance, discounts on training and conferences, and access to member-only content.
For example, there's an archive of winning case studies from over a decade of MRS Awards.
Find out more about the benefits of joining MRS here.
10 Comments
Pete
12 years ago
Little thumbs up logo - these are all useful points. Thanks for sharing (reminding!)
Like Reply Report
Jasper Lim
12 years ago
The best sample is to sample the entire population :)
Like Reply Report
John Grono
12 years ago
Great article Ray. Like all good research it is precise and concise. Kudos.
Like Reply Report
Martin Silcock
12 years ago
We could do with the same for the qual research as well.
Like Reply Report
Anon
12 years ago
A very nice reminder and a good read - thanks Ray. Often we see social media style articles on here where they talk a lot about the handful of successes but have not disclosed its limitations or assumptions. Nice to bring some 'robustness' back to the practice of research!
Like Reply Report
Peter Mouncey
12 years ago
All good points, Ray, and well worth stating. In today's world of commercial confidentiality, I would add transparency as a further key issue. The need to know how something was done and the supporting eevidence to back up claims. Often sadly lacking today. In terms of Lim's response, the entire population would be a good start point, but will the actual responses achieved be representative?
Like Reply Report
Muder Chiba
12 years ago
Timely piece! Whatever data one uses, however its collected or collated , and no matter how sophisticated the processing sieve we run it through- the basics of good scientific practice need to be observed. Many of the shiny new toys are really useful and future-centric but not all of them.
Like Reply Report
Louise Brice
12 years ago
Good article Ray.
Like Reply Report
Glenys Wolstenholme
12 years ago
Thanks for such a concise and erudite exposition of the topic, Ray. Just because we have bright, shiny, new techniques does not mean that the earlier, well-crafted, well-reasoned, robust methodologies fall by the wayside. New approaches can add to insight, but don't mean that what's gone before is rubbish - particularly if the "new" has not yet been properly validated. I suspect we could find parallel analogies from the world of medical/pharma research to caution us against premature adoption of untried "remedies".
Like Reply Report
Miket Thompson
12 years ago
All good stuff Ray. However, I fear things have gone too far for our industry and the norm is fast becoming 'we don't have the budget to do it properly' or 'nobody does it like that any more' . For too long now the industry has gone along with the notion that somene being paid £1 to do a survey on on access panel constitutes a valid respondent. Add to that the fact that most regular access panelists are working for 4 or more companies and doing 30 surveys a week one can see why standards are falling. I feel it will reach a stage where research is devalued so much that companies will not have dedicated research departments. When that happens agencies will be selling directly to line management and I can't help feeling that this might be a good thing.
Like Reply Report