Lessons from experiments: The new age of AI
It’s only been eight months since the release of ChatGPT and the progress made incorporating large language models (LLMs) into research tech has been stratospheric. Since the start of this year, artificial intelligence (AI) has been touted as a solution for survey creation, moderation, analysis, presentation writing and even replacing participants.
The pace of change has been rapid – but what have we learned? 2CV has been exploring the new frontier of AI-assisted research, experimenting, learning and adopting new tools and techniques – here are a few of the lessons we’ve learned along the way.
- AI creates conversational relationships with data – but researchers have to ask the right questions and interrogate the answers
AI helps us make data human – our relationship with data has evolved to the point where we can converse with it. Data has become discursive – AI facilitates discussions with our datasets.
The ability to process transcripts of qualitative sessions or survey open ends and ask questions of the dataset feels truly magical, but ultimately the responsibility lies with researchers to interrogate the analysis, check the evidence and weave the narrative. Crucially, AI can help us to overcome researcher bias, enabling rapid and accurate access to verbatim from specific audiences across an entire sample.
- AI generates reportage, not insights
AI can tell you what happened, but it struggles with what’s important. Asking an AI to ‘write a topline’ is not appropriate usage – it will over-generalise, miss nuance, misunderstand and even hallucinate conclusions.
AI tools are excellent at reportage, they can tell you what happened in a discussion, but lack a sense of what’s important. Your AI model doesn’t have a detailed understanding of your objectives, what certain stakeholders need to know or what details might be of particular interest.
- Input is everything – AI can’t improve bad data, it can only work with what you give it
Transcribed recordings of qualitative sessions are a limited datasets – they can’t tell the AI everything. Transcripts don’t contain tone of voice or body language – they lack emotional nuance. If the quality of recording is poor – AI will struggle to understand it. If you are referring to external visual stimulus without naming it, AI might struggle to know what stimulus is being discussed.
Smart AI can’t make up for poor moderation – AI won’t solve poor question phrasing or missing questions you should have asked.
- Referenced results are essential – AI analysis can’t be trusted implicitly
Be wary of any AI tool that offers ‘instant answers’ without mechanisms for checking where it got the answer from. Researchers must provide editorial oversight for AI – checking the sources to mitigate against hallucination, bias or overclaim. Remembering core skills – referencing the raw data is key, ensuring you have adequate supporting verbatim.
- Don’t believe the hype – AI platforms make big claims, do due diligence, test and learn
Avoid ‘AI-washing’ – marketing materials that emphasise ‘AI-powered everything’ without clear evidence should be treated with suspicion. The intelligence of AI tools vary wildly depending on both the underlying model and the sophistication of the applied usage. Put the tech to the test – conduct small scale pilots before you sell anything to a client. Do due diligence before processing any data whatsoever.
The AI research revolution has arrived – as researchers we need to recognise the opportunity and the urgency of getting to grips with the huge potential of this new technology. Research isn’t just about rapid data analysis – it’s about people. We build relationships with clients, we find out what they want, we ask strangers questions, we weave a narrative to explain it all. We provide the human intelligence and emotional understanding that AI can’t replicate.
We have an opportunity to build a symbiotic relationship with AI as a powerful ally in the research process, with AI providing rapid processing power and humans providing independent thought, an editorial eye, emotional understanding and creativity. Building this sort of relationship requires an in-depth understanding of both the strengths and limitations of AI.
We can’t afford to bury our heads in the sand and pretend that AI doesn’t have the capacity to take on roles traditionally taken by the researcher, because it does. Instead, we must understand what it can and can’t do – exploring how we can do better research in partnership with AI, not in opposition.
Jack Wilson is innovation lead at 2CV
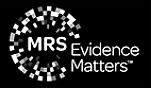
We hope you enjoyed this article.
Research Live is published by MRS.
The Market Research Society (MRS) exists to promote and protect the research sector, showcasing how research delivers impact for businesses and government.
Members of MRS enjoy many benefits including tailoured policy guidance, discounts on training and conferences, and access to member-only content.
For example, there's an archive of winning case studies from over a decade of MRS Awards.
Find out more about the benefits of joining MRS here.
0 Comments