Learning from stock market prediction flaws
The stock market is the perfect example of a ‘prediction market’. The share price of a company is a reflection of the market’s prediction of the future performance of that company. However a high share price is no guarantee of success.
The same is true for the betting industry. People’s bets influence the ‘favourite’ but, while it may have a better chance, the favourite does not always win. One of the reasons being is that the net aggregation of shares bought or sold, or money bet has one big built-in flaw – it presumes a lineal relationship between amount bet and predictive accuracy.
A person buying or selling 100,000 shares or betting a few hundred thousand pounds will have much more impact on the share price/betting odds than 10 people each buying or selling 100 shares or betting £100. That individual will be 100 times more influential. But are they 100 times better informed about the future profitability of a company or the outcome of an event than the 10 other smaller investors/betters?
The answer is, of course, ‘no’, and from a market researcher’s perspective this is significant. There might be a relationship between ‘confidence’ (reflected in the amount that is invested or bet) and prediction outcome accuracy, but that is slight, and non-linear.
So what you have is an unbalanced weighting protocol sitting at the heart of all stock markets and betting odds which contributes to the slightly less than perfect correlation between stock prices and company profits (which hovers as I understand between c0.5 and c0.6 in most markets and an even poorer correlation between being the favourite and winning).
Why is this relevant to market researchers? Well I see a similar flawed aggregation algorithm sitting at the heart of the Net Promoter Score (NPS) rating, designed to gauge the loyalty of customers and often used as an alternative to customer satisfaction research.
There is a similar non-linear relationship between the answers given (on a satisfaction rating of 1-10 ) in response to the question: ‘How likely is it that you would recommend our company/product/service to a friend or colleague?’ and the score which supposedly measures the loyalty of a firm’s customer relationships.
In the calculation to determine the NPS there is an overemphasis in the weighting protocol on the significance of the answers of those who give a satisfaction rating of 9 and 10. Scores of 7 and 8 are regarded as ‘passives’ and disregarded.
But if you statistically correlate individual scores with the overall net sentiment, as we have done recently analysing more than 10,000 individual NPS scores for a cross-section of around 50 brands, we found that 9 and 10 were not significantly more predictive and 7 is, in fact, the most predictive score, but is completely ignored.
Along the same lines, who has fallen into the trap of devising their own points-based aggregation scoring system founded upon ranked selection? Take for instance a brand ranking question scoring 1st place 3 points, 2nd 2 and 3rd 1 point and totting all these up to form a comparative metric – I certainly have in the past. But this is a really flawed aggregation process for the same reasons.
My thought is as market researchers, we could be a lot smarter in the way we go about aggregating opinion in lots of areas. We have the opportunity to set up more intelligent prediction market systems that are actually a little more sophisticated than stock market style protocols. Systems where forecasts are not based purely on net aggregated scores given, but also take into account factors such as personality traits and the track records of individuals, with more nuanced aggregation processes where each score is weighted by its historical predictive accuracy.
Jon Puleston is vice-president of innovation, Lightspeed GMI
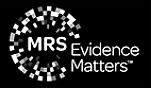
We hope you enjoyed this article.
Research Live is published by MRS.
The Market Research Society (MRS) exists to promote and protect the research sector, showcasing how research delivers impact for businesses and government.
Members of MRS enjoy many benefits including tailoured policy guidance, discounts on training and conferences, and access to member-only content.
For example, there's an archive of winning case studies from over a decade of MRS Awards.
Find out more about the benefits of joining MRS here.
0 Comments