How research companies should respond to the AI revolution
FOBO (fear of becoming obsolete) is a key driver in the massive efforts businesses are taking to ensure their long-term success given what is becoming another technical revolution: the generative artificial intelligence (gen AI) revolution. The management consultants McKinsey have estimated that gen AI could add around $2.6 trillion to $4.4 trillion annually in productivity gains. For context, Europe’s largest economy, Germany, had a GDP of $4.36 trillion in 2021.
While the hype in gen AI definitely warrants serious attention, the research industry should be cautious about the temptation to utilise this amazing technology in the pursuit of misguided strategies, such as an over-focus on efficiency and cost cutting. Taking this route could lead to the tech becoming a disastrous red herring.
Here are my top four recommendations for research companies looking to incorporate AI into how you operate – without losing sight of what is truly important: providing added value to your clients.
1. Invest in your human capital
Specialists are the heart of research companies. So you should view and develop AI applications with a goal of enhancing your human capital. View this technology as tools that can empower your experts, and then invest in their training so they can have the confidence to use these tools well in order to develop bolder insights, faster.
There is a common saying with regards to AI which holds true: good data in, good data out. However, an often overlooked component is just as important: it isn’t only about the tool, but how you use it. A well implemented application of AI can free up more of your experts’ time. Time that can be used to turbocharge their thought leadership capabilities, time to delve deeper into their research in order to surface bolder insights, and time to form closer relationships with clients.
While it’s tempting to see AI purely as an opportunity to downsize your teams in a cost-cutting drive, I would caution against this route, as the short-term gains will leave you vulnerable to watered-down insight and a loss of touch with your client needs in the medium to long term.
2. Focus on improving clients’ user experience
Research companies should be wary of ‘shiny object syndrome’ with regards to generative or conversational AI. Equally, you should not be blind to the merits of traditional machine learning nor have a closed mindset about future technical advancements. In fact, your focus should be on the clients’ user experience: finding tools to solve problems, not problems that can be solved by tools.
While generative and conversational AIs are the hot topic of the moment, your goal should not be to find out where these tools can be used. Instead your goal should be to help clients get to the right insights faster, while reducing the chances of them missing important details. If gen AI helps you do this, then great, but be focused on the goal, not the tool. More data does not mean better data.
3. Importance of ‘closed-loop’ systems
The internet is an incredible resource, but it contains lots of data and content from unreliable sources. Brands can’t afford to make decisions about innovation and marketing or business strategies based on untrustworthy information.
How can you develop an AI that your clients can trust, and that also accurately cites its work? This is the value of a closed-loop system, which only works with the research generated by your own experts. It’s a conversational medium for your clients to get to the research they need faster, without having to wade through irrelevant information, while also maintaining proper referencing and citation. This all helps to keep clients more engaged in the research and ultimately place a higher value in the research services they’re paying for.
Ensuring your application of AI is confined to operate within a universe that you can control is extremely important in ensuring that users will be able to trust its output. Trusted data in, trusted data out.
4. Integrate AI into your business model
You should be restructuring your businesses to respond to the AI revolution, while also remaining nimble for change in the future. This doesn’t necessarily mean outsourcing your AI projects. Instead I would recommend that you focus dedicated time to respond to AI, and how the tech impacts your business. You should also give your colleagues the opportunity and time to become AI experts themselves.
So we can see that while gen AI is without doubt an exciting technology, we have hopefully convinced you not to blindly jump on the tech bandwagon – but instead take a holistic approach. This involves using technology to both prioritise your clients’ needs by guiding them to the right insights in the fastest possible way, while also providing them with more relevant thought leadership content by empowering your experts with the right tools.
Ben Arnold is global head of data science at Mintel
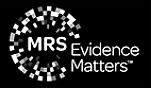
We hope you enjoyed this article.
Research Live is published by MRS.
The Market Research Society (MRS) exists to promote and protect the research sector, showcasing how research delivers impact for businesses and government.
Members of MRS enjoy many benefits including tailoured policy guidance, discounts on training and conferences, and access to member-only content.
For example, there's an archive of winning case studies from over a decade of MRS Awards.
Find out more about the benefits of joining MRS here.
0 Comments