Different versions of the truth
It’s the age-old question – ‘is size important?'. If you’re less than a terabyte you’re not coming in! Big data may not be the buzzword it once was, but it’s the stick that traditional research gets beaten with time and time again.
Big data is the ‘cheap’ and more ‘accurate’ version of its smaller cousin. Survey data is just a few hundred people, guessing what they did and why they did it. Qualitative research may as well not exist, because social media analytics gives me everything I would want to know about the inner psyche of the average consumer!
But this position falls under the same fallacy of comparing qual with quant and asking which is better. The answer is they do different things and both are different versions of the truth.
Both big data and more traditional research have the same issue though – in the hands of an amateur they can be dangerous.
Let’s first of all look at big data – we need a definition for that to be applicable. I’ll talk about it as the vast data streams of transactions, digital data, passive data and social media that are occurring continuously. It is estimated that we produced 2.5 quintillion bytes of data every day in 2017– that’s a lot of data. Why would we need any more? Bring on the analytics and let’s go get me some insight!
We need to be careful – analytics is a loaded word. Many analytic platforms produce nothing more than frequency counts – 30% did this or 60% did that. That’s useful to the extent that it gives us a measure, but it does not give us insight. We have to be cautious because the same rules that we apply to small data do still apply to big data, although many will tell you that because of its size, this is not relevant.
The key question is, what does the data you have represent – is it a good reflection of what you truly want to measure?
Social media analytics argues it reveals what is really going on in peoples’ lives. I’d argue that in many cases it is actually worse than a focus group. Why? Because we project a false persona actively in social media forms. From #greathubby to #blessedlife, we want to be thought of by our peers in a certain way and therefore portray ourselves to meet this requirement.
Social media tools will give us this surface level of detail, but not the qualitative understanding of what lies beneath. It’s this sub-surface that really helps us understand behaviour and motivation and so provides the vehicle to change it.
Transactional data allows us to look at real time behaviour. If we tag a few algorithms on there, then we can cue up products that we’re sure you’ll be interested in. But this is where we are producing dumb data. Every time I search for an item, I’m inundated with ads.
I search for wetsuits and all of a sudden for the next x number of weeks I’ll be seeing ads for wetsuits – even once I’ve bought one. The numbers will show the advertising effective, because if I send it out to enough people, I’ve got to be right a few times – it’s monkeys with typewriters producing the great works of William Shakespeare in a different way.
I’m not saying that’s not useful, but our research shows it leaves a negative customer experience and can lead to suspicion around brands. The trail of data that we leave online is immense and we are starting to see the backlash against those that are using it in blunt ways that create a jarring experience.
Smart data use is where the customer’s data is used to enhance the experience. Amazon is probably the best at this, with its suggestions – which in itself is a customer friendly word, not ads, so algorithms can work effectively to increase sales.
The issue is that they become self-fulfilling. They will start to focus on high probabilities and not allow you to look beyond what they think you need. Algorithms work towards averages and ignore the interesting outliers. They are made to focus the conversation, rather than expand it.
That for me is the issue of using big data alone. The small data approaches paradoxically open up the aperture of thought. We look to understand the outliers and why they occur. We seek to look how the new can change a market, influence customers and create new dynamics. Humans are complex creatures and it needs professionals to unravel the stream of data to understand the question of ‘why’ – not ‘what'.
Without the ‘why', you can’t instigate controlled change. If you focus too much on ‘what', you end up producing more of the same. The ‘why’ gives the capacity for disruption.
The power of multiple aspects of the truth is that you are able to have the ‘what’ and the ‘why'. It’s only then you can get to the ‘what next'.
Lee Naylor is managing partner at The Leading Edge
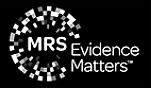
We hope you enjoyed this article.
Research Live is published by MRS.
The Market Research Society (MRS) exists to promote and protect the research sector, showcasing how research delivers impact for businesses and government.
Members of MRS enjoy many benefits including tailoured policy guidance, discounts on training and conferences, and access to member-only content.
For example, there's an archive of winning case studies from over a decade of MRS Awards.
Find out more about the benefits of joining MRS here.
0 Comments