Control your category with conjoint
Manufacturers of fast moving consumer goods face a challenge. Their products not only have to appeal to consumers, they also need to offer a range with a strong presence and clear position in their category. Retailers also need assurance that these products contribute to the overall success of the category if they are to achieve presence on the shelf.
Much can be learned from analysis of sales data, but this has a number of limitations. It can only give information on what has happened in the market, not what could happen (e.g. the introduction of a new product or a major change in price). It is also difficult to pull apart the value of individual products to consumers, especially in categories that are heavily promoted or where distribution is very uneven. This in turn makes it challenging to identify the optimum portfolio and what each product brings to it.
To assist manufacturers and retailers, market researchers can use information from consumer surveys to describe the characteristics, usage patterns and attitudes of category shoppers. These descriptive methodologies enhance understanding of detail behind sales data and provide the basis for modelling future consumer behaviour. However, they still lack sufficiently detailed information about shoppers’ purchase intentions when faced with new product offerings with a variety of prices and features.
This is where category conjoint analysis comes into play. Conjoint analysis has been around for decades, but on the whole, market researchers tend to think of what it can do to assist new product development and ignore its wider applications, especially in simulating purchase behaviour. Category conjoint uses the principles of experimental design and choice modelling to produce realistic models of consumer purchasing within a category.
Optimising category strategy
Some examples of questions that clients ask are:
- What is the optimal range that will significantly increase category share and grow the category as a whole?
- If we expand our product line, will overall revenue grow, or will we suffer too much cannibalisation?
- How should we price our new product to maximise adoption?
Category conjoint addresses all of these. Consumers make buying a product look simple, but they are in fact processing information at an astonishing speed. Much of this process is intuitive and they are unable to explain the complex trade-offs they are making between price, brand, product features etc. Category conjoint looks to replicate the point-of-purchase decision across all elements that drive product choice: brand, variant, pack size, price and promotion. The challenge for researchers is to understand consumer sensitivity to these factors in a realistic but controlled context.
Category conjoint (a form of discrete choice modelling) is a survey technique that draws on the well-established principles of choice-based conjoint. Using large samples (typically a minimum of 1,000 category users) and an online self-completion survey method, researchers are able to build robust statistical models of consumer choice. These go beyond the classic ‘shares of preference’ outputs to present realistic estimates of category volume and values.
Combining market data to enhance business decisions
The ‘share of preference’ outputs from conjoint reflect what consumers say they will do, given a clear set of choices. This alone can be a good guide to how consumers will react to changes to the category. However, out in the real world, consumer choices are constrained by lack of information, habit, advertising, distribution and other factors. The most useful models therefore combine the strengths of conjoint (sensitive measures of relative consumer preferences) with those of actual market data (absolute changes in purchasing behaviour).
If suitable market data is available (e.g. point of sale), it is possible to calibrate the conjoint results to closely match current market shares. If information on current distribution and awareness is also incorporated, the process of aligning shares of preference with actual category shares requires only moderate adjustments. More challenging is the calibration of price movements – where market information exists relating to the introduction of a new product or a change in price (together with changes to competitor products), the analyst can represent these changes in the simulator and observe the changes that actually occurred in the market. If necessary, this can then be used to adjust the price sensitivities in the model.
Conventional ‘usage and attitudes’ market research and sales data describe the current state of a category but do not fully capture the dynamics of the category, particularly in relation to new products, prices or promotions that are not currently present. Some proprietary forecasting methods have proved effective for predicting the potential of individual new products launched at a certain price, but they are expensive and inflexible when it comes to modelling a whole product range, let alone a complete category.
Category conjoint therefore fills a useful gap for manufacturers and retailers who need a good quantitative understanding of their category, the ability to simulate a wide range of possible scenarios and who don’t want to spend much more than the cost of a conventional market research study. The simulator tool that category conjoint produces allows manufacturers to optimise their offering; at the same time it enables them to do this in a way that also effectively serves the needs of retailers.
David Pearmain is director of advanced methods for Impact Research
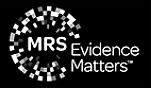
We hope you enjoyed this article.
Research Live is published by MRS.
The Market Research Society (MRS) exists to promote and protect the research sector, showcasing how research delivers impact for businesses and government.
Members of MRS enjoy many benefits including tailoured policy guidance, discounts on training and conferences, and access to member-only content.
For example, there's an archive of winning case studies from over a decade of MRS Awards.
Find out more about the benefits of joining MRS here.
0 Comments