A smarter response to survey challenges
For random sample surveys, it’s long been considered not only good practice but absolutely essential to strive for a high response rate if we are to draw valid conclusions about the population we are studying. The long-term decline in response rates worries researchers who fear that those who aren’t taking part in surveys are somehow different from those who are, and that these differences will introduce bias in the data.
As a result, data collection agencies have adopted expensive strategies for increasing response rates such as increasing the number of calls to non-contacts, refusal conversion attempts and respondent incentives. To some extent these efforts have paid off. At NatCen Social Research we’ve seen the speed of decline slowing and some indication of it having halted on a number of surveys.
But it has taken a lot of money to get where we are – and rising fieldwork costs are particularly problematic at a time when research buyers of all types, be they private or public sector, are looking to reduce costs.
“Are we getting our money’s worth from efforts to maximise response rates by whatever means necessary and at any cost? Studies have shown it is possible to have high levels of non-response bias despite a relatively high response rate”
So it’s time to ask: Are we getting our money’s worth from efforts to maximise response rates by whatever means necessary and at any cost? After all, a high response rate only reduces the risk of non-response bias – it does not remove it completely. Several studies have shown it is possible to have high levels of non-response bias despite a relatively high response rate, and vice-versa. Even within the same survey, some estimates could suffer from non-response bias where others don’t.
Why is this? Because non-response bias only occurs when there is a relationship between the likelihood of responding and the survey variable of interest. For example, a travel survey with a relatively high response rate could still produce biased estimates of travel behaviour if those who travel often are more difficult to contact and therefore less likely to take part in the survey. A survey about science is more likely to produce inflated estimates of science knowledge and show more positive attitudes towards science because those who are not interested in the topic are less likely to cooperate.
If efforts to increase response only result in more of the same types of people taking part, there will obviously be no reduction in non-response bias. Efforts to increase response rates should therefore be based on an understanding of who isn’t responding, why they aren’t and whether their non-response will bias survey estimates.
Yet attempts to increase survey response rates tend to follow the line of least resistance. When reissuing non-contacts and refusals to a different interviewer to chase up, it is common practice to select those cases that are most likely to be converted. However this could potentially increase non-response bias if those who are the least likely to be converted are under-represented in the survey and differ with respect to what the survey is trying to measure.
So what is stopping us from pursuing high response rates in an informed manner? The main obstacle is the lack of good-quality information about non-respondents as well as respondents. Sometimes the sampling frame includes information which could be informative, or the sample could be linked to other data sources, such as administrative records. But more often than not this information is not readily available and extra efforts are needed to collect this data.
In face-to-face surveys, for example, it has become common practice to get interviewers to record observable information to identify those that are less likely to take part in a survey. Such information tends to be limited to what the interviewer can observe without having to make contact or gain cooperation with the respondent. This might be evidence of barriers to access (an entry phone system in a block of flats, for example), the type of accommodation and the interviewer’s subjective assessment of the external condition of the accommodation.
But even when these observations are related to the likelihood to respond, they are not necessarily related to what the survey is trying to measure. And this is the challenge we currently face: to identify information that can be recorded for all sampled cases and is correlated with key survey items.
“Efforts to increase response rates should be based on an understanding of who isn’t responding, why they aren’t and whether their non-response will bias survey estimates”
At a minimum, this kind of information could be used to improve post-survey adjustment for non-response bias. But there is also great potential for using this information during the course of data collection to improve survey representation of reluctant respondents. Furthermore, we can use this information to control costs of data collection by not wasting resources on respondents whose characteristics suggest that they are unlikely to respond even after intensive efforts.
This kind of intervention during data collection is called ‘responsive design’. In a 2006 paper on the subject, Bob Groves and Steve Heeringa explain that: “Responsive designs use paradata to guide changes in features of a data collection in order to maximise the quality of estimates per unit cost. Responsive designs require the creation and active use of paradata to determine when a phase of the survey has reached its phase capacity and what additional features might be complementary to those of the current phase.”
Groves and Heeringa give several examples, some dating back half a century, of multi-phase sampling, and how incentives and re-contacts can be tweaked in each phase to “reduce the cost inflation common in the later stages of survey data collection” and reduce non-response errors.
Although research using responsive design is still in its infancy, some encouraging results have been reported by the University of Michigan and Statistics Canada, and many other organisations are exploring their own opportunities for trying out responsive designs.
Meanwhile, methodologists are tackling challenges such as the collection of relevant information about non-respondents compared to respondents, but also other indicators of data quality and cost. There’s still some way to go, but the application of responsive design is likely to increase over the next five to ten years – especially given the continued pressures on researchers to control survey costs.
Gerry Nicolaas is head of data collection methodology at NatCen Social Research
- For more on managing costs in survey design, see Friday’s article:ICM sees cost benefit of adding mobile to poll samples
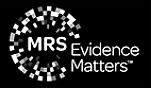
We hope you enjoyed this article.
Research Live is published by MRS.
The Market Research Society (MRS) exists to promote and protect the research sector, showcasing how research delivers impact for businesses and government.
Members of MRS enjoy many benefits including tailoured policy guidance, discounts on training and conferences, and access to member-only content.
For example, there's an archive of winning case studies from over a decade of MRS Awards.
Find out more about the benefits of joining MRS here.
0 Comments