Weighing big data’s potential
This is big data – and it’s big news for companies everywhere. Why? “Big data promises to deliver huge amounts of insight that would have not been possible by conventional means,” says Colin Strong, managing director of GfK’s technology division in the UK. And these insights are expected to be game-changing. A 2012 survey by Capgemini, published in the report The Deciding Factor, found that on average business executives expect big data to improve organisational performance by 41% over the next three years.
There’s clearly a lot of excitement surrounding big data. It’s near the top of the hype peak on the Gartner Hype Cycle. But there’s plenty of action to back up the talk, says David Pittman, an IBM social media strategist. “What we have seen in our research is that maybe 30-40% of companies are actually partaking in some sort of big data initiative right now,” he says.
Many companies are still firmly in the “investigative” phase, according to Pittman. But early days or not, Pete Cape, global knowledge director of SSI, says: “I don’t think we as market researchers can ignore it. People wonder why anyone would pay for a well-constructed survey of a thousand people if they have a database sitting there with a million customer records they could mine.”
Digital exhaust
Market researchers approach big data with a curious mix of fear, envy and intrigue. For some, it’s a threat – Research has heard tales of clients warning that big data will render survey work redundant – while others see opportunity. Generally speaking, says Cape, “it causes us mental discomfort to think about”.
He’s right when he says that big data is too big to ignore. But also, in many ways, it is too big, too messy and too widely dispersed to do much useful with, at least in its raw form. Much of what constitutes big data is what the McKinsey Global Institute calls “digital exhaust”: the stuff given off as a by-product of consumers going about their business – visiting websites, buying food, checking in on Facebook or tweeting what they had for breakfast.
Cape defines big data as “data that has been gathered sideways – that is, it wasn’t deliberately set out to be collected, but it’s there”. In his paper Three Eras of Survey Research, former US Census director Bob Groves refers to it as “organic data”, drawing a clear distinction with the “designed data” produced by market research.
“The questions we ask of households create data with a pre-specified purpose, with a use in mind,” wrote Groves. “This means that the ratio of information to data (for those uses) is very high, relative to much organic data.
“What has changed in the current era is that the volume of organic data produced as auxiliary to the internet and other systems now swamps the volume of designed data. The risk of confusing data with information has grown exponentially.”
Bigger isn’t always better
Plenty would agree with Groves’s point that just because you have a lot of data, it doesn’t mean you have a lot insight waiting to be discovered. In her paper Six Provocations for Big Data (co-authored by Kate Crawford of the University of South Wales) Microsoft researcher Danah Boyd spoke of “a problematic underlying ethos that bigger is better, that quantity necessarily means quality”. In reality, she says, “the size of data being sampled should fit the research question being asked: in some cases, small is best.”
“It’s fashionable to talk about how much data we have available,” says David Boyle, head of insight for EMI Music and Zeebox, “but any business question is usually answerable by a sub-sample of that.” Speaking at the first gathering of the Big Data Insight Group this year, Boyle said that the first steps in any successful big data project are identifying the business question you want to ask and working out what data you need to answer it.
“You have to know what data is available, or could be available, and whether it is appropriate,” he says. “You don’t want to use data about the wrong subset of users. So if you’re interested in mainstream consumers you wouldn’t want to use Twitter data – they’re not mainstream consumers, they’re a very specific type of consumer.”
Meanwhile, it is important to keep in mind that no matter how big a big data set is, there’s only so much it can tell you about a customer – as opposed to customers. 2CV chief executive Doug Edmonds, the agency’s former head of numbers, likens many big data sources to Google in its earliest days. “Initially, Google knew an awful lot about various small moments in time, and that moment was when you typed your search into its search box and hit enter,” he says.
“That’s what big data is about. It can involve slightly more complex interactions but it’s still a snapshot of a small amount of behaviour in the lives of consumers.”
Perhaps the biggest criticism to be levelled at big data is that it is simply a bunch of facts without all the messy details, like a history book recounting everything that happened without explaining why it did. Take customer loyalty card data: it can tell you what someone buys going back years and years, but when purchase behaviour changes it offers little in the way of explanation.
“A Coca-Cola drinker might suddenly switch to Pepsi because of an accumulation of things that have happened, none of which could be predicted by their past behaviour,” says SSI’s Cape. You might be able to make an assumption to explain this behaviour based on other changes in the purchase data record, such as whether a person has started buying other new products or has switched to other cheaper brands (perhaps they have a new flatmate or have lost their job?). But they might not be correct.
Blocking the view
“What is not the same as why,” says GfK’s Colin Strong, who similarly highlights a lack of context as a weakness in many big data sets. “One of the key issues with the mainstream approach to big data is the apparent reliance on analysing associations. The problem is, association does not always get it right.”
The idea that companies might, at some point, want to do away with conventional research and rely solely on big data analysis seems absurd. The overriding view is that big data and survey data complement each other
He gives the example of a data analyst investigating relationships between different people in a network. Strong says: “It is easy to assume that the frequency of contact is equivalent to the strength of relationship. However, we know this is not the case, otherwise our strongest relationships would be with our work colleagues – which is patently not the case.”
Big data also offers companies a fairly limited view of the world outside their own customer base – with each business holding their own commercially sensitive information close to their chests. Indeed, 2CV’s Edmonds sees a danger here that big data obsession could distract companies from paying attention to what the competition is doing. “Big data is a strength,” he says, “but it might also expose companies to weaknesses.”
David Boyle is acutely aware of these risks. At EMI he has access to a number of big data sources, including streaming data from services like Spotify. “There’s billions of records in the data and data people love it,” he says, “but the problem is it’s very specific. It’s about how people use the Spotify service. There’s a whole lot of extra info on those people we would like to know beyond what track they listen to.
“We want to understand what they’re like as a person and how the rest of the market behaves. In the end, it’s survey data that ends up answering most people’s questions on most projects most of the time. For us, nothing can replace survey data for that base level of understanding about what’s going on in the world and the context into which everything else fits.”
Still asking questions
Doug Edmonds says we are living in an age of behavioural enlightenment. “Not only can we measure behaviours more accurately, we also know more about the decision-making processes – be they rational or emotional – that are driving these behaviours,” he says. “These two trends go hand-in-hand.”
The idea that companies might, at some point, want to do away with conventional research and rely solely on big data analysis is, it would seem, absurd. All data sources have their individual strengths and limitations. In the case of big data and survey data, the overriding view seems to be that they complement each other.
For market research, Strong says, “The era of big data allows us to start exploring human behaviour and specifically human social interaction in a way that has never before been possible.
After all, our existing theories of human behaviour, including human social behaviour, have previously been based on relatively small samples”. Meanwhile for big data, surveys bring much-needed attitudinal and emotional context in which to understand the behaviours that take place.
Success in a big data world, though, does require several things – starting with a new set of skills. “Most people aren’t familiar with how to work with big data, or even medium data,” says Boyle – but people are important. Lots of big data projects fail because companies don’t have the right people in place. The question is, are market researchers up to the task?
The Value of Big Data
A 2011 report by the McKinsey Global Institute identified five ways that big data would create value for organisations:
- Creating transparency
In areas like manufacturing, McKinsey said, integrating data from across business units could significantly cut time to market and improve quality. - Enabling experimentation
Real-time delivery and analysis of performance data would allow businesses to test and adapt more easily. - Customising actions
Tailor products and services to highly specific segmentations. - Automated decision-making
Retailers, for instance, could use algorithms to fine-tune inventories and pricing in response to real-time sales data. - Innovating products and services
Detailed usage data could feed into the development of new and updated products.
Big Data at Work
American Express analysed customer behaviour and found that people racking up large bills on their cards and then registering a forwarding address were likely to declare bankruptcy.
Academic researchers used Google Earth to develop a theory that cows have a magnetic sixth sense. Satellite images showed that two-thirds of cows around the world align their bodies with the magnetic north.
US retailer Target analysed shopping baskets to identify pregnant women based on the purchase of items like unscented body lotions and vitamin supplements. One pregnant high-schooler in Minneapolis started receiving offers for baby clothes before she’d even told her parents.
Google tracks the use of certain search terms around the world to monitor the spread of the flu virus. Its data closely matches the official data but is available much more quickly.
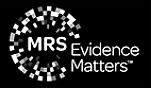
We hope you enjoyed this article.
Research Live is published by MRS.
The Market Research Society (MRS) exists to promote and protect the research sector, showcasing how research delivers impact for businesses and government.
Members of MRS enjoy many benefits including tailoured policy guidance, discounts on training and conferences, and access to member-only content.
For example, there's an archive of winning case studies from over a decade of MRS Awards.
Find out more about the benefits of joining MRS here.
2 Comments
Maury Giles
13 years ago
Great piece. Explains also why the synthesis of big data must include pattern recognition, associations, AND simulation to get at emergent behavior and better understand the dynamics of human behavior and interaction systems. In other words, we have to be able to go beyond statistics and mathematical tools with these data. We have the capacity now to use computation such that we can simulate system dynamics to deconstruct what combination of factors yield specific outcomes - this is why agent-based technology is a key element of the future of making big data actionable for better decision-making and strategic planning.
Like Reply Report
Swati Arora
13 years ago
Great Article! Puts a lot of perspective to the big data analytics and how it complements the survey data.
Like Reply Report