The history of the data economy: Analytics arrives
“Seeing is the new asking.”
That’s what Stephan Gans, chief insights and analytics officer at PepsiCo, constantly tells his colleagues.
“If you ask a mother, or you ask a dad, ‘what do you give your kid in his lunchbox that he takes to school?’ you can predict what the answer is going to sound like,” says Gans, “because the dad wants to be seen as a responsible dad. So, there’s some fruit, there’s some this, there’s some that….”
But by recording what actually happens, using 24/7 cameras in people’s kitchens, analysed by artificial intelligence, researchers found that one conscientious father packed his son’s lunchbox every day with carrots, fruit, and so on, and every day the boy came home with his lunchbox completely intact. The child found eating all those carrots took up too much valuable play time and he bought something quick to eat instead.
“You would have never learned that from the dad,” says Gans. This, in a nutshell, is the problem with asking questions. People might tell you what they believe to be true, or what they want to be true. The actual truth, though, may elude even the most diligent of interviewers.
Henry Durant, the first president of the Market Research Society, knew of these problems with survey-based data collection, and warned about them in the 1950s (as we learned in the previous article in this series). As technology has developed and the data economy has evolved, ‘seeing’ has become ‘the new asking’.
Jon Ward, vice-president of sales EMEA at eye-tracking company Tobii, offers another example of direct observation being more informative than asking questions.
“A lot of people will say they’re not price sensitive, and then completely demonstrate price-sensitive behaviour when they are not being asked, because it’s like saying, ‘Are you cheap?’ ‘Of course I’m not cheap!’” Wearing Tobii’s compact cameras, shoppers can go into physical stores and shop normally. With every detail recorded for analysis, “you see them go in,” says Ward, “and, yes, they 100% are cheap”.
In the age of store cards and barcodes, collecting data on what people eventually buy is easy, but eye-tracking analysis also reveals the moment their eyes stop on competitor brands, the two bottles they pick up, and even the glance between price per 100ml on both brands that clinches the deal. Analysing what people look at online is easier still, when volunteers put eye-tracking cameras above their screens and give the researchers access to what they are viewing.
Mike Follett, managing director at eye-tracking company Lumen, thinks implicit observation techniques share three key advantages over asking questions explicitly.
“The first one is: people don’t know what they don’t do,” he says. “It’s very, very hard to know that you definitely didn’t see something. Given the fact that attention is selective, we have been ignoring the vast majority of human experience to focus on explicitly remembered actions and opinions.”
The second advantage is that people are very bad at accurately remembering things that they actually did do or see, even in situations as important as giving evidence in court.
The third advantage, says Follett, “is that, when it comes to marketing, ads might be developed in isolation, but they’re always seen in context. So, making sure that you serve up the experience to be as close to reality as possible, and then understand how attention works within that reality, is very important.”
Although 21st-century technology allows observations to be filmed by cameras smaller than your thumb and analysed by machine-learning algorithms, implicit observation has its roots in methods from the 20th century and before. The first eye-tracking was done by a human looking through a flap in a hoarding, hand sketching where passersby were looking.
From the 1950s, behaviourist approaches became more popular as a way to understand human beings. Polymath Herbert Simon brought together his interests in psychology, economics and computing to develop the idea that simply observing what people do is a better guide to what they will do in future than asking them explicitly. We may be rational, but our rationality is bounded by the finite amount of time and attention we have to spend.
Today’s data-driven consumer insight combines the kind of individual observation described above with aggregated data, to see overall trends and behaviours.
Media monitoring – the observation of what consumers are looking at, listening to or reading – is as old as media. Early newspaper adverts offered discount coupons to find out how many readers of different newspapers paid enough attention to the advert to cut them out and use them.
Nielsen, founded in 1923, pioneered automatic monitoring of a household’s radio and television habits, supplemented by diaries to track individuals within a household. They also pioneered indexes of retail sales data in the 1930s, combined with panels of consumers who recorded, and later scanned, their actual purchases, enabling advertisers to compare adverts seen with goods bought.
Early point-of-sale systems enabled larger stores and chains to track what was being bought directly. When American chain JCPenney first installed cash registers linked to mainframe computers at its distribution centre in 1969, as well as speeding up customer transactions, the Arizona Republic reported: ‘The Glendale store manager knew, day by day, just what merchandise was being sold and how much.’ After the invention of the barcode in 1971, chains such as Walmart could track goods in enough detail to predict demand.
Tesco was the first to offer shoppers a direct trade of discounts for data in 1995: sign up for a Clubcard and get targeted offers, in return for letting the retailer observe your shopping habits. In the first year, five million people signed up. Edwina Dunn and Clive Humby, who devised and ran the Clubcard, eventually sold their data company Dunnhumby to Tesco for more than £90m.
In the 1970s, marketing databases began to merge with another rich source of data: the credit report. Since the early 19th century, merchants have exchanged information on their customers, to predict which ones might be a bad risk for settling their bills.
The Manchester Guardian Society, founded in London in 1826, merged with mail-order giant GUS in 1996, and eventually became part of international credit bureau and data broker Experian. A similar process saw American credit bureau Equifax expand into the field of marketing by acquiring data companies and demographic modelling software. Now it could segment people not only into creditworthy and uncreditworthy, but also into marketing categories, such as ‘upper crust’ and ‘living off the land’.
Geodemographics, the ability to sort people into demographic and consumer categories linked to where they lived, emerged in the 1970s from newly available, computer-readable census information.
The Claritas Corporation, founded in 1971, sorted Americans into 40 types, including ‘money and brains’ and ‘hard scrabble’. Meanwhile, in the UK, social scientist Richard Webber used the 1971 census to study inner-city deprivation in Liverpool. His Classification of Residential Neighbourhoods (CRN) system formed the basis for the Acorn system later used by data company CACI1. This social segmentation used experimental work being done within companies and independent agencies.
From diesel to jet fuel
Phil Barnard, who would later run Kantar, describes the UK market research field of the 1970s as “almost a cottage industry”. Research projects would alternate qualitative and quantitative methods to define and explore questions, designing experiments almost like medical trials, with Latin squares and ‘control groups’.
Even in the early days, when analysis was done with log tables and slide rules, and questionnaires were hand-tabulated on paper, sophisticated statistical models underpinned the work of Barnard and his contemporaries. The Fishbein Model, for example, captures consumers’ attitudes to a brand or product in mathematical form. If those attitudes change in certain ways, the model helps predict the change in sales or market share.
Cluster analysis could segment people by interests and habits, as well as by basic demographic categories. “You’d have a battery of questions,” says Barnard, “which you knew from your basic research clung together. You’d ask somebody how much they agreed with a particular statement: ‘I like going on holiday’; ‘I like meeting people’. From that, you would produce a small subset of those questions, maybe three of them. You could use those responses to classify the person on that particular criterion and do that over a number of different dimensions. That would enable you to classify people into different segments.”
All these methods and approaches were being used together, often by the same people. The thing that transformed data from diesel to jet fuel, however, was the ability to combine diverse data sources and create one multidimensional picture.
In 1989, Ken Baker, Paul Harris and John O’Brien presented the results of their experiment in data fusion to the Market Research Society conference.2 They concluded that different datasets could be combined to give results comparable to putting a larger questionnaire to one population.
When they wrote up their work seven years later, they commented that “we have a new buzzword – integrated targeting – the merging/linking/matching of market research databases. Is this the way the industry is moving as the millennium approaches? A lot of researchers would conclude that this development seems to be inevitable.”3
Writing in the same year, Bill Blyth, chief statistician at TNS, and Tim Bowles comment that the problem for market researchers has changed from a lack of data to a proliferation of data from different sources: electronic point of sale data, consumer panel data, pooled retailer records, and so on.
Given this proliferation, they say, “it is inevitable that market research practitioners will move away from their traditional stance as collectors and purveyors of research data. Since they will have to obtain appropriate market intelligence to establish adjustment factors, they will inevitably become involved in the organisation and analysis of diverse data sources.”4
The ‘inevitable’ has certainly come to pass. The Esomar 2020 industry report describes the impact of data analytics on market research: “As new ways to gather data have emerged, which do not require a ‘real-time’, one-to-one personal interaction between researcher and respondent, methodologies have increasingly moved from being an ‘active’ process or collection to a passive, less intrusive, less conscious (and in some people’s view, a more accurate) recording of behaviour and generation of information, for the researcher to use to generate insights.”5
Today, Esomar estimates the value of analytics to the data, research and insights industry at around $47bn, slightly more than half the sector’s entire worth.
I asked Gans to estimate what value data analytics adds to his work for PepsiCo. “I think we spend $2bn a year just on advertising,” he says. “Say that you’re 10% more effective in targeting the right consumers and convincing people to buy your brand: you’re talking about saving millions and millions.”
Follett sees the switch to data-led marketing as a revolution comparable to Robert Hooke’s Micrographia, a 17th-century bestseller that introduced readers to images seen through early microscopes, revealing hidden details of everyday life.
Just as Hooke’s work established experiment and observation as the basis of science, and changed readers’ ideas about their place in the world, Follett thinks data-led research will transform not just methodology, but our understanding of ourselves. However, as a group of Harvard College students would soon demonstrate, this was only just scratching the surface.
You can download the complete four-part ‘History of the data economy’ here.
The author thanks Adam Phillips and the Archive of Market and Social Research (AMSR) for assistance with researching this article.
Reference:
1. Post-war developments in market research (The Archive of Market and Social Research, n.d.), 39.
2. John O’Brien, Paul Harris, and Ken Baker, Data fusion: an appraisal and experimental evaluation, Journal of the Market Research Society, 39, no. 1 (January 1997 ): 1-52, https://doi.org/10.1177/147078539703900101.
3. O’Brien, Harris, and Baker, 225-26.
4. Tim Bowles and Bill Blyth, How do you like your data: raw, al dente or stewed?, Journal of the Market Research Society 39, no. 1 (January 1997 ): 163-74, http://search.proquest.com/docview/214810877/abstract/4D9635B56D044861PQ/1.
5. Global Market Research 2020, an ESOMAR industry report (Esomar 2020 ), 57.
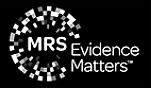
We hope you enjoyed this article.
Research Live is published by MRS.
The Market Research Society (MRS) exists to promote and protect the research sector, showcasing how research delivers impact for businesses and government.
Members of MRS enjoy many benefits including tailoured policy guidance, discounts on training and conferences, and access to member-only content.
For example, there's an archive of winning case studies from over a decade of MRS Awards.
Find out more about the benefits of joining MRS here.
0 Comments