In the Mix
Marketing mix modelling (MMM), in which historical data is fed into a model to try to optimise the return on marketing investment, has been a tool for marketers since the early 1990s. In the 2010s, it has created a flourishing sub-discipline: predicting the demise of marketing mix modelling. “To be provocative, I believe it will become obsolete,” said Laura Desmond, then CEO of Starcom MediaVest Group, in 2011.
“If MMM is to survive, it is essential that it change and experience a rebirth,” the editors of GreenBook claimed in September 2016. For this article, Michael Wolfe – one of the pioneers of the technique in the 1980s – told Impact that he believes the discipline is “in crisis” (see p30, A crisis in MMM?).
Like any statistical tool, MMM can’t please everyone all of the time. There are marketers who can’t learn much of any interest from the available data – for example, if there is a particularly long and complex path to purchase. For others, their market is simply too volatile, or too focused on one aspect of the marketing mix. For digital platforms, competition is increasingly for the market – rather than in the market – so this makes a sophisticated mix model less relevant.
Steven Levy’s book The Plex, which tells Google’s early story, reports that when Scott Epstein joined the company as vice-president in 1999, he proposed a marketing plan based on optimising the mix of the ‘four Ps’ – product, place, price and promotion. It was rejected by the founders, and Epstein left the company. A Google insider dismissed the relevance of marketing mix for a start-up that had market dominance as its goal: “Do we want to put money into the technology, into the infrastructure, into hiring really great people? Or do we want to blow it on a marketing campaign we can’t measure?”
There are also econometric and data-quality limitations for all marketing mix models, which may frustrate clients when they are explained, and give false confidence if they aren’t. Variables in the model affect each other, as well as the outcome that the modeller is trying to predict, making it hard to do accurate ‘what if’ predictions.
“It’s no longer a journey where you see a TV ad and, when you’re in Sainsbury’s, you buy a can of beans. It’s more like: you see a TV ad; you might search for it; you might end up on a brand site; you might have a friend who sent you a video; you might see an ad that you hate and you start complaining about it on social media. There are a lot more paths of communication and of interaction with brands,” says Rob Sander, a principal consultant at Data2Decisions, who is working with digital media owners to build models that capture the role of influencers such as social media.
The data sources can also be hard to mash up into a single model, as they are measured at different levels of granularity – store level, household, individual or segment – and with different frequency and precision. Models compare many types of data that correlate poorly: some behavioural, some financial and some attitudinal. This means small changes in the model structure or weight given to each can lead to very different conclusions. A marketing mix model takes months to build, and may use years of data, so it attempts to predict the future using information that might be out of date – or not match the market structure – by the time the modelling process is complete.
Finally, even if the modellers can solve the complex problems of creating a robust model, there is a danger that the process creates a black box that clients struggle to trust. The allocations it predicts could be either a compelling insight demanding action, or a side-effect of the modelling process.
Given these flaws, why do it? Because, for many brands, the results of using this type of data – even if the models are far from perfect – often clearly outperform any other approach. In a 2013 study published in the International Journal of Research in Marketing, Frank Germann et al found that marketing analytics created an 8% higher return on assets for the companies that used it, rising to 21% in highly competitive industries, with all sizes of organisation benefiting. If the answer to doubts about accuracy in the modelling process is to increase trust in gut feel, or do the same thing as last year, that isn’t palatable to most clients. Which is why, Sanders says, any dissatisfaction with large-scale marketing mix models is not a rejection of the principle of using data to try to optimise marketing.
“It’s always exciting to say something’s dead,” he says.
Other vendors – all of whom offer alternative types of marketing analysis if clients require it – have similarly not noticed a reduction in demand for the models. “We build models for 60% of MediaCom’s billings – and the other 40% are, more than likely, building MMMs with another third party. Our clients realise that it’s an important way to measure the effectiveness of their advertising budgets,” says Matthew Wragg, head of attribution at MediaCom. “Today, this isn’t just about finding out how well TV is doing compared to press, but also how this fits into the wider marketing picture – the wider commercial perspective.”
“I think marketing mix modelling still has legs,” says Nat Greywoode, who works in the market insights and analytics team at Twitter, and who has spent his entire career building marketing mix models. “In my mind, there is no alternative.”
While the challenges to MMM’s effectiveness are getting more complex, Greywoode’s argument is that techniques to improve the models are known, even if they are not universally implemented yet. The problems of integrating new types of data – especially the social media data that Greywoode is working with modellers to incorporate into their MMMs – are the sort of knotty challenges that, in every industry, inspire econometricians to greatness or drive them to despair. But they are problems with solutions.
How the sausage is made
Statistical models – not just in marketing – create two significant and related problems. The first is that the map is not the territory; every model is a subjective simplification of reality that is built to solve specific problems. “My favourite analogy – the most complete map of the Earth is Earth itself, but a simpler map is much more useful,” says Koen Pauwels, a professor of marketing at Özyeğin University, Istanbul, the author of It’s Not the Size of the Data – It’s How You Use It, and a specialist in the theory and practice of modelling the value of marketing.
This means that the modeller will always be trading off simplicity – and transparency – against realism. Experience can suggest how to build a good model, but it cannot offer a definitive guide to every structural decision. Again, like all econometrics, modelling the marketing mix is both an art and a science – and the state of that art is constantly being tweaked and refined. “It’s not just a mechanical process of chucking some data into a piece of software, which spits out some numbers that you provide back to the client,” says Sanders. “What we try to focus on is building the first stage – then you can make recommendations and have discussions with your client about what the insights may be.”
This often leads to a second misconception: that a market mix model will give definitive answers to every question asked of it. In reality, it can only suggest evidence, based on historical information, that has been measured as accurately as was practical. “You’re trying to assist decision-making. Because it is based on numbers, I can see how people jump to the conclusion that it will give a true answer – but there is no true answer,” Sanders says.
This is why the teams doing the modelling have learned to be sceptical of surprising results from their prototypes, or radical innovations from newcomers. Wragg says he regrets that a few “cowboys” attempt to catch attention by creating models that seem to show exciting results. “That’s a benefit of being in a big team,” adds Emma Whitehouse, associate director, MediaCom Business Science. “When you get surprising or unexpected results, there’s always another client that has probably run something similar. You can look across a group of clients to get some benchmarks, to give you a better feel.”
In a recent case, Whitehouse was surprised to find a cinema campaign performing unexpectedly well. She cross-checked with colleagues, and found that similar effects had been seen in other models – but with the proviso that the effects were mostly short-term. That gave her the contextual information to trust the result and present it appropriately.
If using context, precedent and common sense improve modelling – and data quality is fundamental – at least one of those factors may be in short supply. In which case, sometimes smaller, cheaper or more generic models are all that is possible. Marketing-mix modelling may not be easy, or even possible, for new entrants to a market or start-ups, which don’t have enough historical data to feed the models. In those cases, the client can still rely on the breadth of data that the model builder can access.
Nielsen, which has more depth than most, now offers marketing mix modelling built to solve exactly this problem. Earlier this year, it launched Benchmark Media Optimizer; this is designed to use benchmarks for its custom models as proxies for marketers who want to improve their performance using data, but who don’t have data to feed a model. Because MMM uses aggregates, Nielsen’s aggregated data – on the returns on marketing investment of 1,600 brands in 30 categories, in more than 40 countries – may improve a client’s decision-making, but, as the company admits, it “won’t deliver the breadth and depth of results” of a custom-built model. One constraint might be that a model for a new entrant – as in the Google anecdote mentioned earlier – does not account for the customer’s perception of the quality of the product, which may be one of the dominant drivers of growth.
At the other end of the scale, as more marketing becomes data oriented, it may be tempting to throw every piece of marketing data into the mix. It’s intuitive that, by doing this, you will end up with a model that captures everything useful – as close to Pauwels’ globe-sized map of the Earth as it’s possible to get. Unfortunately, econometrics doesn’t work this way.
Apart from the time and cost of collecting data, the models are confounded if many of the inputs used are closely related to each other, and all related to the outcome – so it may give exciting, but wildly inaccurate, predictions. If you use the accepted threshold that you are 95% confident something is affecting sales, and you report 20 influences, one or more of them will randomly seem important.
“Just by chance,” Sanders explains, “some things will correlate positively. Then, if you chose to interpret that as an actual causal relationship, you’ll say ‘oh, look – this tiny thing drove 10% of sales’. You can end up in quite ridiculous situations if you’re not careful.”
This limitation – that too much data may sometimes be as big a problem as too little – is driving dissatisfaction in some clients, because their fastest-growing sales channel generates large amounts of data: “The biggest question around MMM at the moment,” says Wragg, “is what we do about digital.”
The digital challenge
“In the past, networks such as ours – and social media in general – have formed a small percentage of the media budget,” says Twitter’s Greywoode. “It is growing, but, historically, this hasn’t been the case. So the pressure inside brands to come up with an accurate RoI for digital marketing has been a lot less.” As digital channels mature, he points out, that’s changing; clients need hype-free, defendable recommendations.
But digital activity needs careful modelling. For several years, this has been a problem of attribution models, which are the bottom-up equivalent of the top-down MMM. Attribution draws inferences from the brand and product interactions and behaviour of a representative sample of individuals; MMM uses the aggregate behaviour of groups, and attempts to tease out the contribution to the aggregate of each marketing activity.
Both are valid ways to answer different questions, and both have limitations in the questions they can answer. Attribution is often constricted in that it models only what it knows; for example, it can identify that an online search led to a buying decision, but not always what prompted the search. As search is highly correlated with TV advertising, for instance, switching budget from TV to paid search on the basis of a poor attribution model would be self-defeating. This has occasionally led to unsustainably high RoI predictions for digital channels.
With MMM, the challenge for digital media owners is to work out not just how much they contribute to the success of marketing, but also how the effect works. Twitter is just one such company partnering with model-builders to create sophisticated models that reflect its true place in the marketing mix.
Ideally, improvements in modelling would be a win-win-win, even before brands get involved; digital media owners capture sustainable marketing budgets that represent the true returns on marketing investment, model builders improve their quality, and brands can make better decisions.
Digital is far from a simple challenge for modellers, because social media often works by catalysing other types of marketing spend. This means that there are collinearity problems – when the return on offline goes up, so does the return on online – and temporal issues; not all the sales effects of digital happen at the same time, so may not be captured in a slice of data. This means that naively adding spend on Facebook, Google search or Twitter to the model without careful thought may decrease that model’s ability to predict.
“We’ve seen not only a direct impact of Twitter exposure on sales, but also an indirect impact. So Twitter kind of influences your customer on to the next step of the journey, which is intuitively understandable,” Greywoode explains. “This is the No 1 area where brands are missing a step, in that they don’t tend to look at the stages along the way to a sale. They look at how Twitter exposure equals sales, whereas there are other impacts that would maybe ladder-up to that.”
Neustar MarketShare is another model builder attempting to improve how it treats digital in the marketing mix. It recently partnered with Google, and can offer its clients slightly more granular search data in its marketing mix models.
A recent example of how this data could be used was a benchmark analysis on the respective contributions of television, digital, video, social media, search and other forms of advertising as an influencer of sales for action films. It found that TV was the most effective influencer, but it swallows 82% of marketing budgets, so is over-invested. On the other hand, increasing digital investment from 10% to 34% of marketing budget would improve RoI for distributors.
In other areas, Neustar MarketShare predicts where the influence of digital on sales has been hyped – and then its models may also dampen expectations, it admits. Dirk Beyer, vice-president of data science research, doesn’t try to pretend that modelling digital’s influence is easy. “Paid search, for example, is highly correlated to who is in the market, or how many people are in the market for your product at a given time,” he says. “It’s highly correlated to seasonality, to the hype that’s around the product, and so on. If you just throw in paid search as a single variable in a monolithic model, it will look like everything you do has been driven by paid search.
“We’re now in a position where we get that data directly from Google, at a level of granularity that is exactly what our brands need. That allows us to do a much better job teasing out the actual impact of search versus – for example – the upstream advertising that got people to the point where they started searching. That’s a big deal for our clients.”
The difficulties of working with digital data should not cause clients to abandon their models, Sanders says – just to question the providers about how digital has been integrated. He also argues that it’s not a reason to abandon this type of modelling, because these are the type of econometric challenges that model builders such as IRI (see p34, More than one tool) have been facing for years.
It has similarities to the way models face the challenge of splitting the immediate sales effect of advertising from its long-term brand-building effect (see p30, A crisis in MMM?), or the interaction between advertising and promotion. “It is interesting to compare how something like Twitter or Facebook works compared with TV, press or out of home. Actually, we have found they are quite similar,” Sanders says.
“There would be a danger if you obsess about measuring Facebook or Twitter’s RoI, and you change your model in other ways to make that happen. Then you’ve probably just built a bad model.”
A better model
The solution to building the best possible marketing mix models may sometimes lie outside the models themselves. With new data sources that interact with each other, markets that evolve, and the need for rapid decision-making, creating a monolithic piece of software that answers every marketing budget question (until machine learning and artificial intelligence can replace econometricians) may not always suit the commercial imperative that created MMM in the first place.
In that case, MMM may become a toolbox of methods, rather than one model, designed to answer a range of questions in a coherent way.
The first challenge is how to model smaller customer segments using MMM. While large FMCG brands such as Mondelēz (see p28, Mondelēz has a 3P mix) do not use MMM for this, other clients do. IRI, for example, is creating models that can capture the returns in increasingly small segments – but granularity of data is a constraint.
“MMM is a top-down, aggregated viewpoint,” says Wragg. “And with only so many data points, there are small channels that we have, historically, not spent lots of money on, or don’t reach millions of people – so we sometimes struggle, statistically, to pull those factors out of the models.” For such models, he suggests, attribution – enhanced to capture the impact of non-digital spend – may sometimes produce more actionable insight.
At Data2Decisions, a strand of research focuses on how to combine the top-down and bottom-up approaches, in what Sanders calls an “ecosystem” model. “It’s the same kind of metrics, but we just broaden out the scope of our analysis. Rather than simply building a model of sales, we’ll also build a model of each of the digital touchpoints, which will typically be things such as search volume, the website and social pages. There are a lot more paths of communication and paths of interaction with brands. It’s the interaction between the different media and touchpoints.”
Having modelled the relative influences of those touchpoints, the output can then be fed back into the marketing-mix model to improve its recommendations.
Increasingly, marketing-mix models may also take advantage of natural, or planned, experiments. Correlation between an input and a key performance indicator (KPI) of a model – such as social media sharing and sales – might be causal; the more people share, the higher your sales will be. Or both might be caused by a third factor – such as TV advertising. Finding two sets of data for which the main difference is that one group was exposed to TV advertising and the other was not would settle the argument.
Brave clients may even decide to run an experiment to test model predictions – an approach recommended by Pauwels when he builds models for his clients. “Individuals and organisations need to experiment to figure out what really works for them. Unfortunately, experiments on a large scale are often considered costly, risky for one’s career, and tough to sell, politically, in the organisation,” he says. “Who wants to be in the ‘control group’ for a potentially very successful new marketing initiative?”
Pauwels built a model for an office-furniture company, which then decided to settle the question of causality by running a field experiment, which divided the market into two groups. One group doubled the spend on what was predicted to be the most effective channel, funded by cutting the least effective one in half.
“Profit, net of marketing, increased 14 fold in the experimental group versus the control. After the experiment, we again modelled the data and showed the company should stop reducing the least-effective action, but it still had room to increase the more effective one,” he explains. Pauwels calls the approach MEME – model, experiment, model, experiment.
Ultimately, though, the test of a model isn’t just how accurate it is – it’s how effective it is at helping the client to make better decisions.
Increasing complexity isn’t always the way to go; creating the structure to answer precise questions will achieve better results than simply adding more data, says Pauwels, who might use the output of one model to decide how to build the next stage.
“Senior managers often ask me to evaluate the marketing allocation among communication channels. For this purpose, I would not include information on the specific campaigns within each channel. Next, I may build a model that distinguishes the separate effect of TV campaigns, treating the other marketing channels as control variables. Then these smaller models can fit into bigger models.”
“At the end of the day,” Greywoode adds, “MMM is a bet. It’s hopefully as good as you possibly can get. Clients should challenge their vendors to make the models as representative as possible, but – once they are satisfied with that – focus more on the uses and measuring the outcomes.”
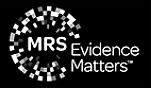
We hope you enjoyed this article.
Research Live is published by MRS.
The Market Research Society (MRS) exists to promote and protect the research sector, showcasing how research delivers impact for businesses and government.
Members of MRS enjoy many benefits including tailoured policy guidance, discounts on training and conferences, and access to member-only content.
For example, there's an archive of winning case studies from over a decade of MRS Awards.
Find out more about the benefits of joining MRS here.
0 Comments