Can AI understand emotion?
“Can a machine grasp the emotions of a warm-blooded human being?” That was the question Leo Paas, professor of marketing and director of the master of business analytics programme at the University of Auckland asked the audience at the start of his IJMR presentation in London this week.
Across multiple studies, Paas, associate editor of the IJMR, and the research team utilised a transformer, or large language model (LLM) called Roberta. Built by Facebook – an upgrade on Google’s Bert – this model utilises 25,000 neurons, which is the same amount of neurons as a sea slug. And while a sea slug has taken millions of years to evolve, said Paas, Roberta was built in two.
The presentation focused on two studies in which Roberta was trained on millions of pieces of unstructured social media data to identify text references that corresponded to one of Ekman’s six emotions (anger, surprise, disgust, enjoyment, fear, sadness). The aim was to see if this type of text analysis could benefit two real-world challenges – fundraising and podcast engagement.
In the case of the Fred Hollows Foundation, whose mission is to end avoidable blindness, the findings could help the organisation to tailor its communications to encourage donations.
Having trained the model on five million tweets (harvested from the pre-Musk period, more on that later) the study found a clear correlation between the emotional tone of the organisations’ own tweets – that of ‘sadness’ – and the amount of funds raised in a given month.
“We're dealing here with avoidable blindness. If the foundation can encourage feelings of sadness about it – that increases people’s intention to donate,” said Paas.
The second study looked at what emotional levers could be pulled to increase engagement in podcasts; engagement is measured here in number of reviews and ratings.
Analysing two million Apple podcast reviews, the dominant emotion in 89% of user reviews was, perhaps unsurprisingly, ‘enjoyment’.
However, alternative emotions were present too, for example ‘anger’. While much less prevalent, their presence significantly added to engagement. What was critical in this instance, said Paas, was not to overplay the alternative emotion, but to find the optimum amount. If exceeded, the users would be pushed away and wouldn’t engage.
Paas explained: “If you just annoy them a little, that’s where you’ll get a review. The predominant emotion of podcasts is enjoyment, but if you want reviews and engagement, don’t make it all happiness. There has to be mixed emotions.”
While Roberta outperformed other machine learning models, there is room for error considering the nuance of text-based language. An emotion can be interpreted in several contradictory ways – for example, ‘surprise’, which can describe a positive or negative emotional state.
The conclusion was one we’re hearing across the industry when applying artificial intelligence to research processes – AI can detect emotions in limited scenarios, but it still requires quite a high level of ‘human oversight’. It is most useful in the pre-research phase – for example, to test a hypothesis.
Of course, both studies were dependent on having access to huge amounts of public data – something that is not so easy today, said Paas, now that X is under new management and is less willing to collaborate with academia.
What’s more, considering X’s well-documented bot problem, the exponential increase in fake data here, and across social media more generally, is a challenge for the whole enterprise of social media listening.
Ultimately, said Paas, issues around data quality could lead to AI ‘model collapse’. It’s a glum outlook, and not one that Paas necessarily ascribes to, but has gained traction especially since the July publication of a paper in Nature. The theory is that models are trained on an ever-increasing body of synthetic data created by earlier generations of themselves; so the connection to the original real world data recedes further and further. In a recursive death loop, the output of these models becomes increasingly distorted and redundant.
In such a doomsday scenario, despite the faster pace of evolution for Roberta, the sea slug may still win the race to understand human emotion.
About the study
The fine-tuning of Roberta was conducted during the PhD research of Sanghyub John Lee who was supervised by Paas, for marketing and business analytics perspectives, and Ho Seok Ahn, with an enginering perspective. Successful application of AI technology requires such interdisciplinarity.
The complete list of co-authors on these projects are:
- Sanghyub John Lee (University of Auckland)
- Ho Seok Ahn (University of Auckland)
- Rouxelle DeVilliers (Auckland University of Technology)
- Hyeyeon (Christine) Yuk (Korea University)
Access the paper:
'The power of specific emotion analysis in predicting donations: A comparative empirical study between sentiment and specific emotion analysis in social media': Sanghyub John Lee, Leo Paas and Ho Seok Ahn, IJMR Volume 66, Issue 5, June 2024.
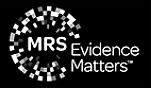
We hope you enjoyed this article.
Research Live is published by MRS.
The Market Research Society (MRS) exists to promote and protect the research sector, showcasing how research delivers impact for businesses and government.
Members of MRS enjoy many benefits including tailoured policy guidance, discounts on training and conferences, and access to member-only content.
For example, there's an archive of winning case studies from over a decade of MRS Awards.
Find out more about the benefits of joining MRS here.
0 Comments